A Novel Hierarchical Cross-Stream Aggregation Neural Network for Semantic Segmentation of 3-D Dental Surface Models.
IEEE transactions on neural networks and learning systems(2024)
摘要
Accurate teeth delineation on 3-D dental models is essential for individualized orthodontic treatment planning. Pioneering works like PointNet suggest a promising direction to conduct efficient and accurate 3-D dental model analyses in end-to-end learnable fashions. Recent studies further imply that multistream architectures to concurrently learn geometric representations from different inputs/views (e.g., coordinates and normals) are beneficial for segmenting teeth with varying conditions. However, such multistream networks typically adopt simple late-fusion strategies to combine features captured from raw inputs that encode complementary but fundamentally different geometric information, potentially hampering their accuracy in end-to-end semantic segmentation. This article presents a hierarchical cross-stream aggregation (HiCA) network to learn more discriminative point/cell-wise representations from multiview inputs for fine-grained 3-D semantic segmentation. Specifically, based upon our multistream backbone with input-tailored feature extractors, we first design a contextual cross-steam aggregation (CA) module conditioned on interstream consistency to boost each view's contextual representation learning jointly. Then, before the late fusion of different streams' outputs for segmentation, we further deploy a discriminative cross-stream aggregation (DA) module to concurrently update all views' discriminative representation learning by leveraging a specific graph attention strategy induced by multiview prototype learning. On both public and in-house datasets of real-patient dental models, our method significantly outperformed state-of-the-art (SOTA) deep learning methods for teeth semantic segmentation. In addition, extended experimental results suggest the applicability of HiCA to other general 3-D shape segmentation tasks. The code is available at https://github.com/ladderlab-xjtu/HiCA.
更多查看译文
关键词
3-D dental models,3-D shape segmentation,geometric deep learning,multistream information fusion,orthodontic treatment planning
AI 理解论文
溯源树
样例
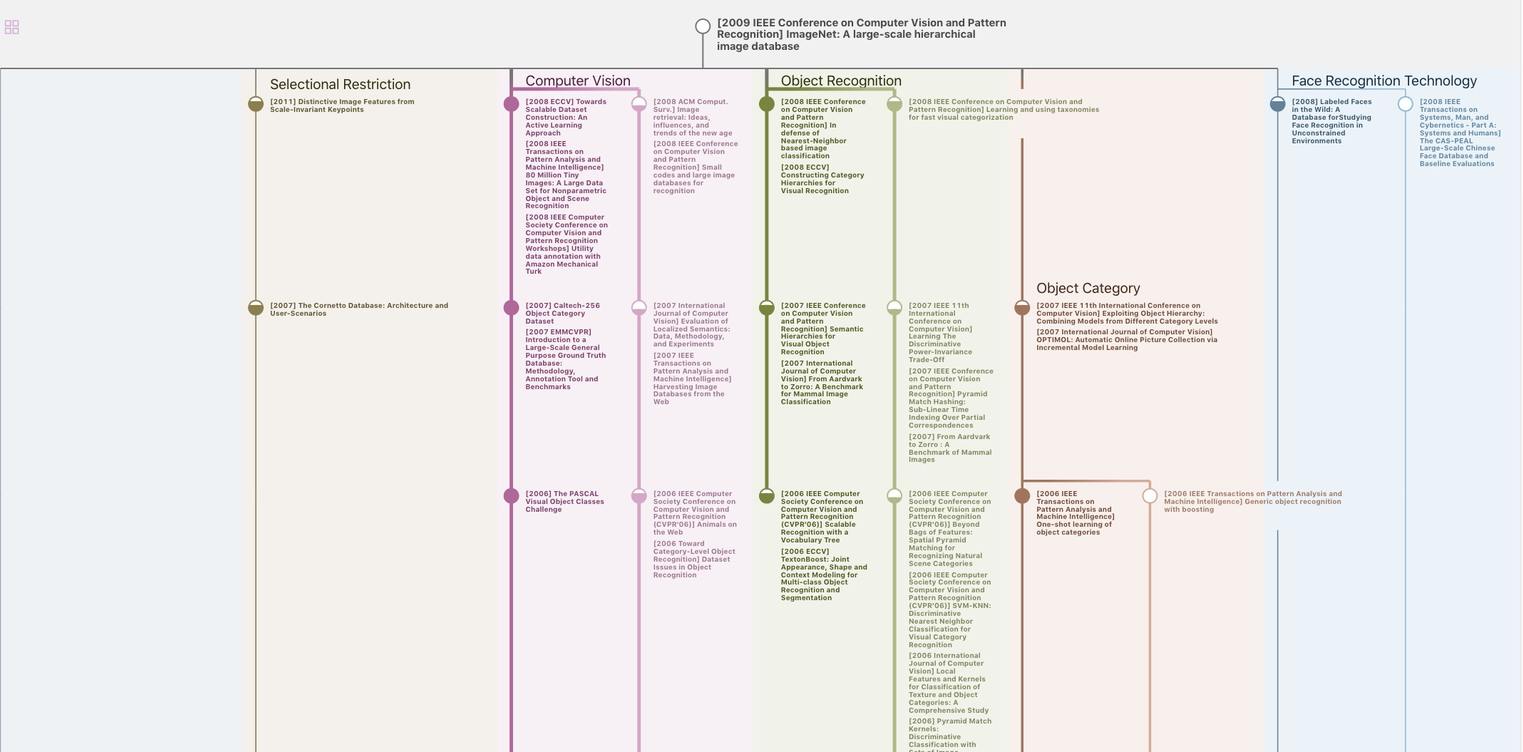
生成溯源树,研究论文发展脉络
Chat Paper
正在生成论文摘要