Using AFDL Algorithm to Estimate Risk of Positive Outcomes of Microbial Tests at Food Establishments
msra(2008)
摘要
OBJECTIVE The objective of the research summarized in this paper is to evaluate utility of the Activity From Demographics and Links (AFDL) algorithm [1] in predicting likelihood of positive isolates obtained from microbial testing of food samples collected at the U.S. Department of Agriculture (USDA) controlled establishments. BACKGROUND One of the common tasks faced by the USDA food safety analysts is to estimate the risk of observing positive outcomes of microbial tests of food samples collected at the slaughter and food processing establishments. Resulting risk estimates can be used, among other criteria, to drive allocation of FSIS investigative resources. The AFDL is a computationally efficient method for estimating activity of unlabeled entities in a graph from patterns of connectivity of known active entities, and from their demographic profiles. It has been successfully used in social network analysis and intelligence applications. In order to test its utility in the food safety context, we treat a co-occurrence of the same strain of bacteria (in particular a specific serotype of Salmonella) in samples taken at different establishments at roughly the same time, as a link in the graph spanning all of the USDA controlled establishments. Now, given the historical patterns of linkage and the information about the distribution of the currently observed microbial positives (which make the corresponding establishments “active” in the AFDL terminology), we aim at predicting which of the remaining establishments are likely to also report positive results of tests. Even though such definition of a link produces uncertain data given that the co-occurrences of specific test results at different establishments may be purely coincidental and our analysis does not attempt to distinguish them from truly correlated instances, we expect that using this inherently noisy data in combination with demographic features of establishments, would lead to useful predictability of microbial events. RESULTS We tested AFDL’s ability to predict which of the monitored establishments would record at least one positive for Salmonella Montevideo over six months following two years worth of training data. Figure below presents lift charts summarizing the results. Using only demographic features of the establishment to make predictions does not help much as the result is barely distinct from what can be obtained by randomly sampling the entities. But adding noisy linkage information data improves recall of active establishments. Using only Montevideo cooccurrences to create links does not in general surpass the results obtained previously, but it does help much at the left hand side of the graph, that is for shorter subsets of the top ranked establishments. That actually brings about practical utility as the investigators would typically begin their work by inspecting the most likely active entities first, and that is where Montevideo-based AFDL provides an immediate improvement. However, using all types of matching serotypes to estimate connectivity patterns substantially boosts the characteristic, both in the context of a short list, as well as overall. That can be explained by the benefits of the higher density of graphs created using more observed connections when using all known serotypes rather than just one to model potential relationships between establishments.
更多查看译文
AI 理解论文
溯源树
样例
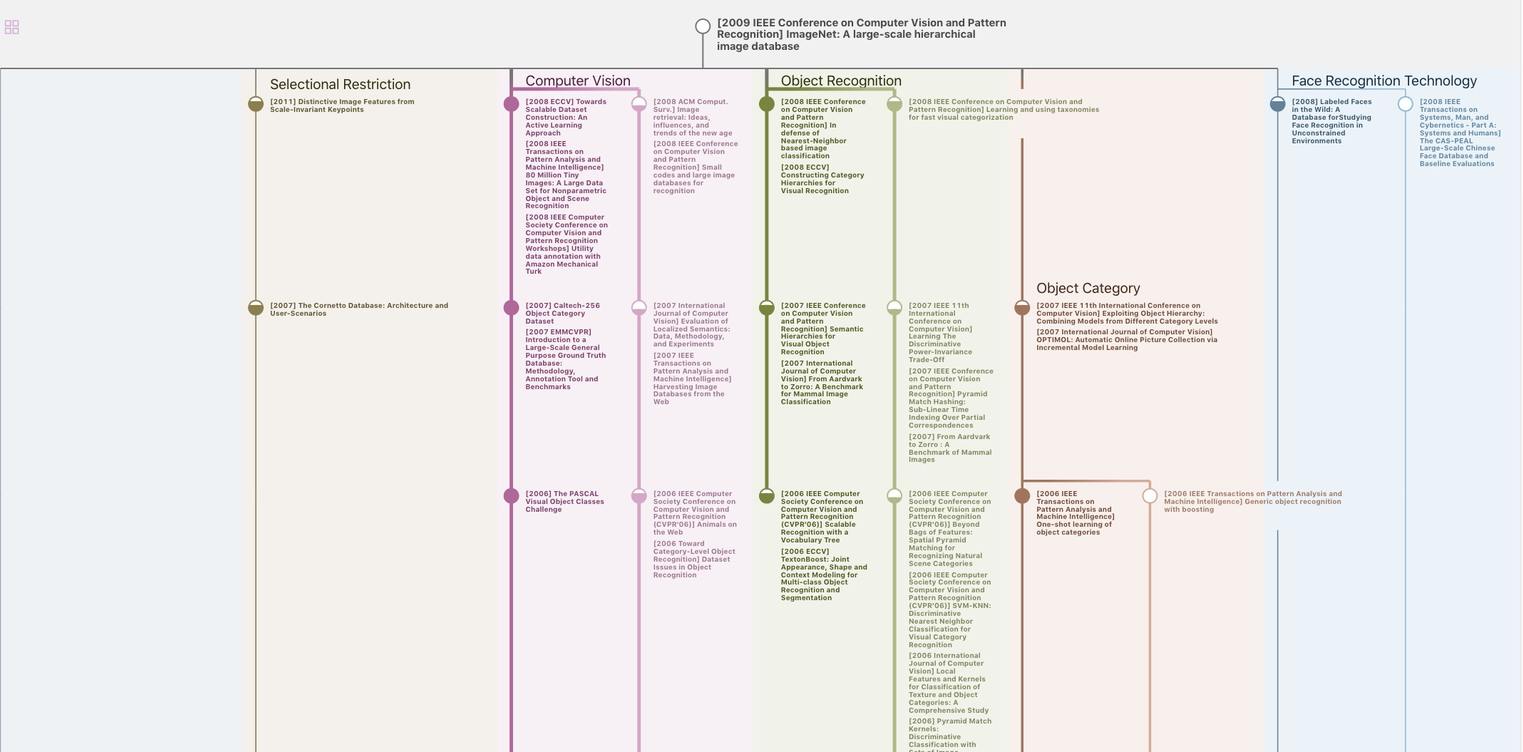
生成溯源树,研究论文发展脉络
Chat Paper
正在生成论文摘要