Towards efficient semantic real time mapping of man-made environments using Microsoft's Kinect.
ROBIO(2011)
Abstract
We propose a novel approach for efficient semantic mapping of Manhattan-like structure i.e. the frequently observed dominance of three mutually orthogonal vanishing directions in man-made environments. First, we estimate the Manhattan-like structure by using an MSAC variant that estimates the Manhattan system directly from the 3D data. In contrast to other methods we use only the normal vectors of each voxel rather than estimating it indirectly using plane estimation. In a next step we estimate the translative motion of the robot relative to the Manhattan system using constrained visual odometry. The mapping is done using a geometric constrained ICP using a-priori knowledge on the estimated Manhattan system. The ICP registers only points within the same geometry to each other. We show that the geometric constrained ICP generate maps with a significant smaller angular drift than an unconstrained one. Octrees are used for map representation in combination with kd-Trees for ICP. We show the robustness of our Manhattan-estimation using real world data. In this paper we demonstrate our approach using a Microsoft Kinect, while the approach will work with all kind of 2.5D sensors.
MoreTranslated text
Key words
mobile robots,a priori knowledge,robots,estimation,real time,semantics,visual odometry,real time systems,kd tree,geometry,vectors,motion control,sensors
AI Read Science
Must-Reading Tree
Example
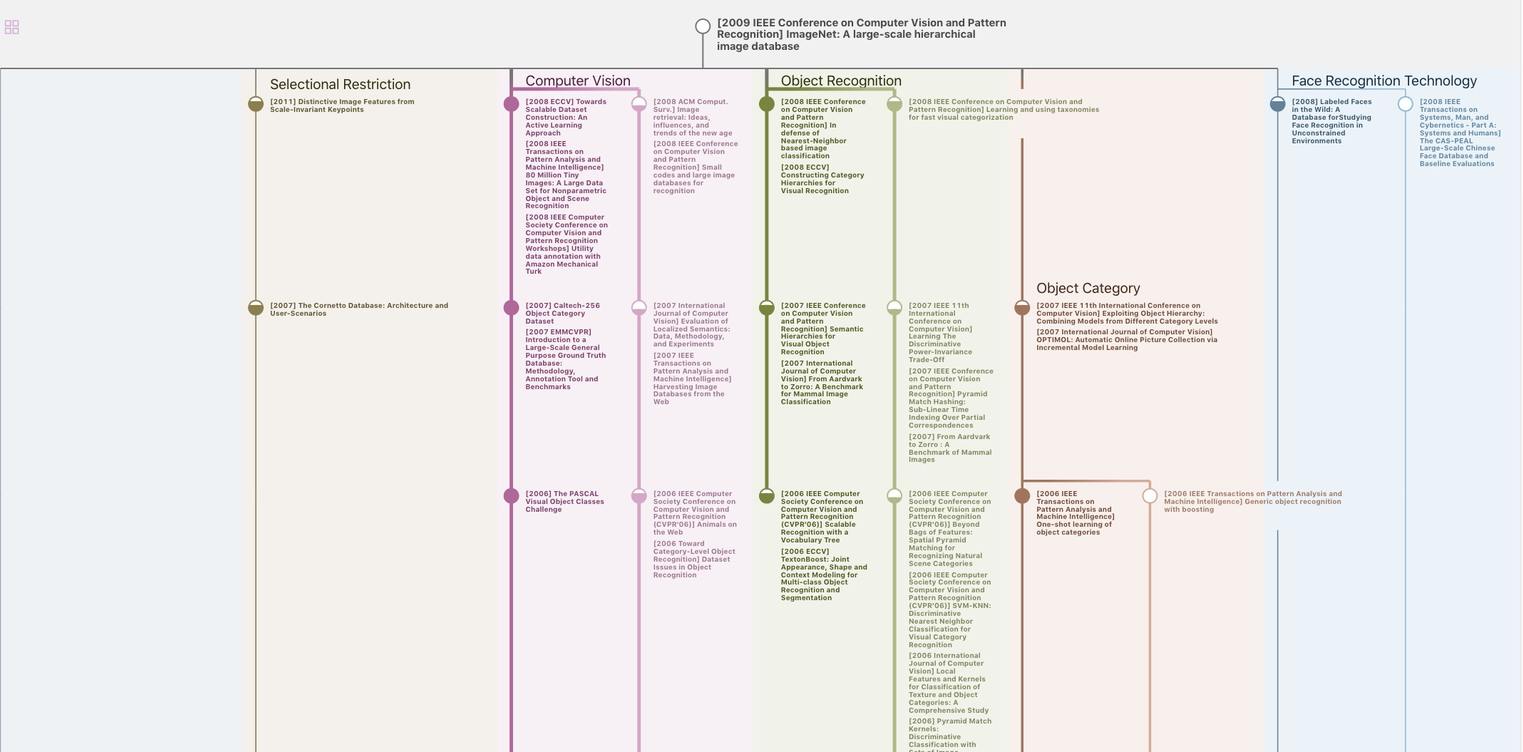
Generate MRT to find the research sequence of this paper
Chat Paper
Summary is being generated by the instructions you defined