MESH participation to TRECVID2008 HLFE
TRECVID(2008)
摘要
A group of four organizations from the MESH consortium (www.mesh-ip.eu) participated this year for the first time in the High Level Feature Extraction track in TRECVID. The partners were Telefónica I+D (TID, Spain), Informatics & Telematics Institute (ITI, Greece), National Technical University of Athens (NTUA, Greece) and Universidad Autónoma de Madrid (UAM, Spain). We submitted a total of 6 runs, using different variations and configurations over a common model. With only one exception, results obtained by those runs were below expectations, mostly due (we believe) to some implementation bugs discovered afterwards. Some of those errors have already been solved and we hope to correct the rest and improve the performance of the system for future editions. 1. Introduction This is the first participation of the partners in the MESH consortium in the TRECVID HLFE track (though some of them had previous experience in past editions separately). The MESH project developed a common visual analysis infrastructure to detect high level concepts in visual scenes; though the set of concepts was only partially coincident with those of TRECVID 2008 (in MESH it is tuned to news content). The system had then to be adapted and trained for the concept set in TRECVID, and for the MAP metric used for evaluation here. In the course of the development a few new techniques, not originally present in the MESH system, were also tried. With only one exception (a motion activity computed over the video stream) all the remaining data extracted from the media was done on still keyframes; for those the reference shot segmentation provided by Fraunhofer-HHI for TRECVID (2) was used. We did not use audio information for any of the runs. The main architecture of the HLFE system is based on well-known paradigms in visual analysis, such as MPEG-7 descriptors, SIFT interest points and SVM classifiers. Nevertheless, we hoped that the specifics of their combination would provide good results. Moreover, one guiding principle in the development was not to include human intervention in model selection and configuration for each individual feature. This rule stems from our aim to be able to generalize the system to any additional feature without resorting to human intelligence to select and combine adequately the available set of tools. The system, thus, gets trained blindly with a ground-truth training set, and adapts automatically to the specifics of each concept during this training phase.
更多查看译文
关键词
visual analysis,ground truth,model selection,feature extraction
AI 理解论文
溯源树
样例
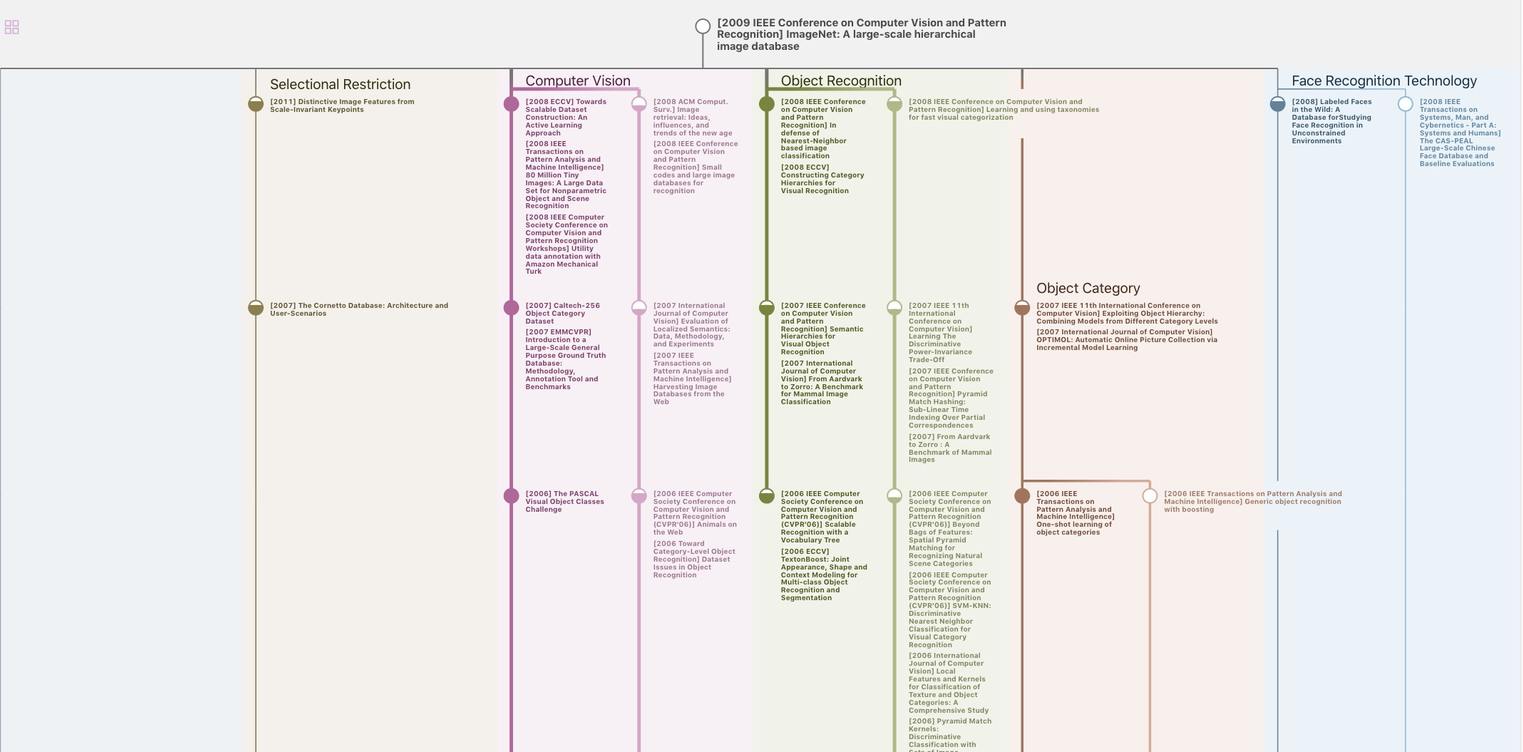
生成溯源树,研究论文发展脉络
Chat Paper
正在生成论文摘要