Context identification for efficient multiple-model state estimation
IROS(2007)
摘要
This paper presents an approach to accurate and scalable multiple-model state estimation for hybrid systems with intermittent, multi-modal dynamics. The approach consists of using discrete-state estimation to identify a system's behavioral context and determine which motion models appropriately represent current dynamics, and which multiple-model filters are appropriate for state estimation. This improves the accuracy and scalability of conventional multiple-model state estimation. This approach is validated experimentally on a mobile robot that exhibits multi-modal dynamics.
更多查看译文
关键词
multiple-model state estimation,multiple-model filters,hybrid systems,finite automata,index terms— hidden markov models,timed automata,state estimation,intermittent multimodal dynamics,multiple-model filtering,hidden markov models,discrete-state estimation,filtering theory,context identification,continuous systems,discrete systems,hybrid system,mobile robot,hidden markov model,indexing terms
AI 理解论文
溯源树
样例
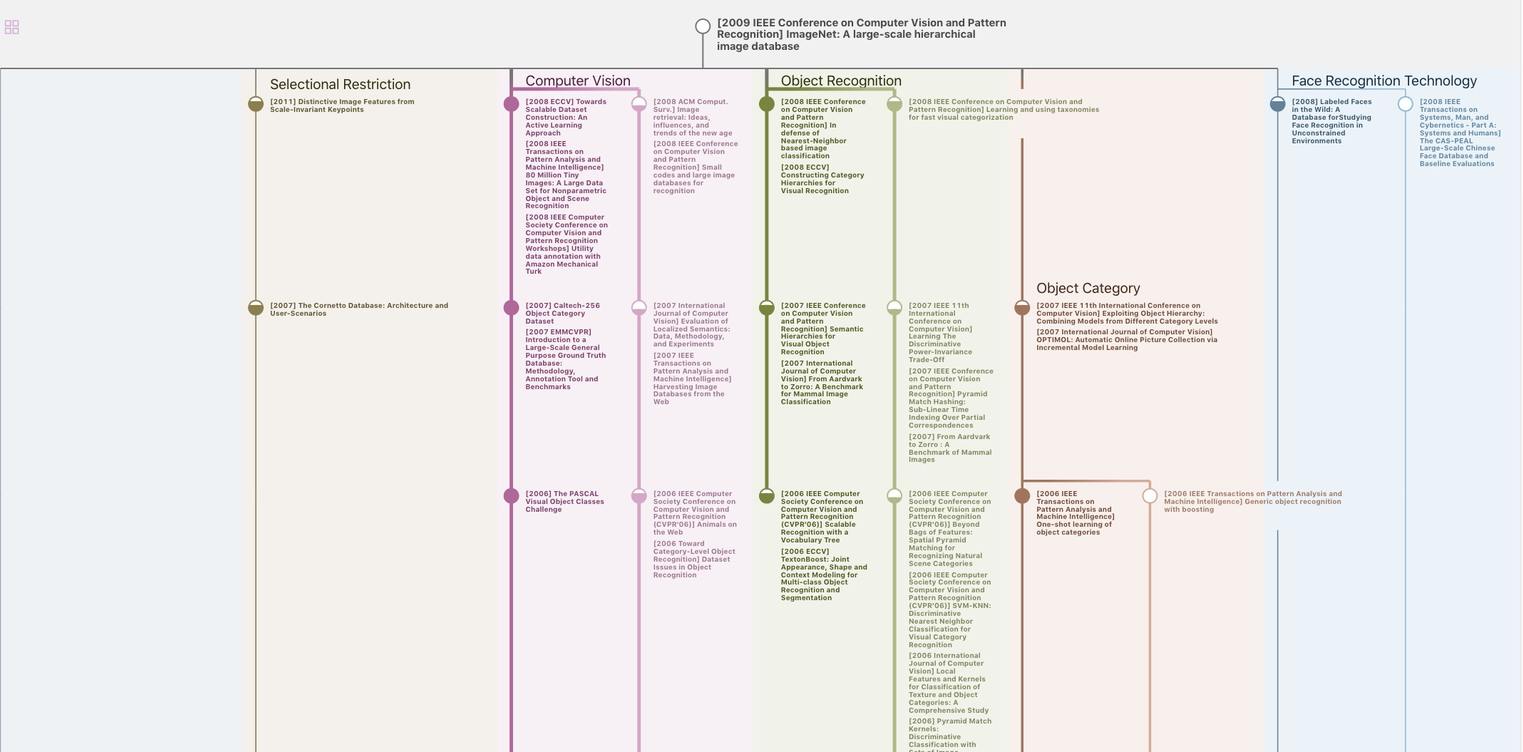
生成溯源树,研究论文发展脉络
Chat Paper
正在生成论文摘要