Dialog Act Tagging Using Grapiucal Models
2005 IEEE INTERNATIONAL CONFERENCE ON ACOUSTICS, SPEECH, AND SIGNAL PROCESSING, VOLS 1-5: SPEECH PROCESSING(2005)
摘要
Detecting discourse patterns such as dialog acts (DAs) is an important factor for processing spoken conversations and meetings. Different techniques have been used to tag dialog acts in the past such as hidden Markov models and neural networks. In this work, a full analysis of dialog act tagging using different generative and conditional dynamic Bayesian networks (DBNs) is performed, where both conventional switching n-grams and factored language models (FLMs) are used as DBN edge implementations. Our tests on the ICSI meeting recorder dialog act (MRDA) corpus show that the factored language model implementations are better than the switching n-gram approach. Our results also show that by using virtual evidence, the label bias problem in conditional models can be avoided. Also, we find that on a corpus such as MRDA, using the dialog acts of previous sentences to help predict current words does not improve our conditional model.
更多查看译文
关键词
hidden markov models,language model,dynamic bayesian networks,bayesian methods,dynamic bayesian network,neural network,graphical model,speech recognition,testing,hidden markov model,predictive models,conditional models,neural networks,graphical models,natural languages,time series data
AI 理解论文
溯源树
样例
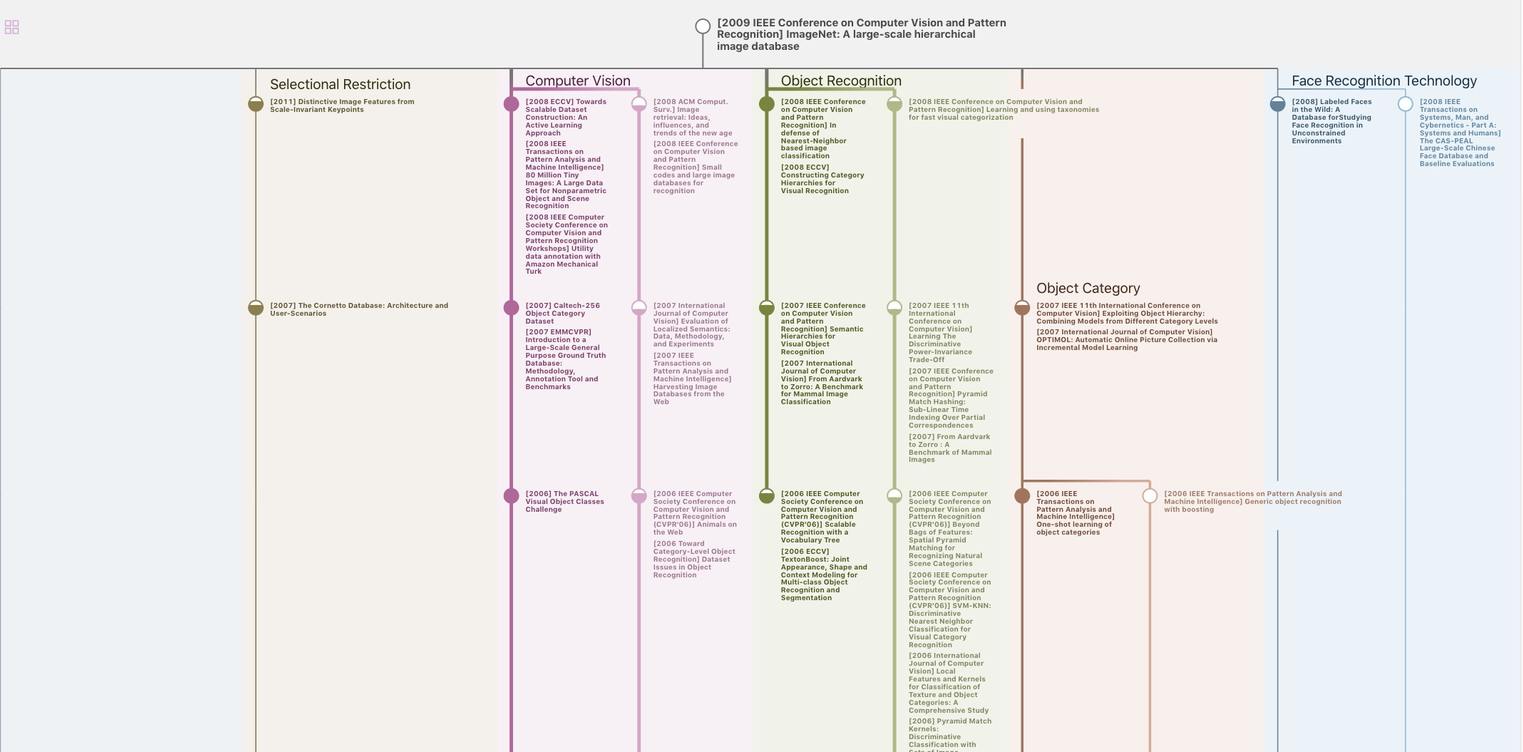
生成溯源树,研究论文发展脉络
Chat Paper
正在生成论文摘要