A comparison of GEC-based feature selection and weighting for multimodal biometric recognition
IEEE Congress on Evolutionary Computation(2011)
Abstract
In this paper, we compare the performance of a Steady-State Genetic Algorithm (SSGA) and an Estimation of Distribution Algorithm (EDA) for multi-biometric feature selection and weighting. Our results show that when fusing face and periocular modalities, SSGA-based feature weighting (GEFeWSSGA) produces higher average recognition accuracies, while EDA-based feature selection (GEFeSEDA) performs better at reducing the number of features needed for recognition.
MoreTranslated text
Key words
feature weighting,distributed algorithms,eda,estimation of distribution algorithm,eigenface,feature selection,steady-state genetic algorithm,multimodal biometric recognition,local binary pattern,biometrics (access control),feature extraction,genetic algorithms,gec,ssga,face,face recognition,accuracy,iris recognition
AI Read Science
Must-Reading Tree
Example
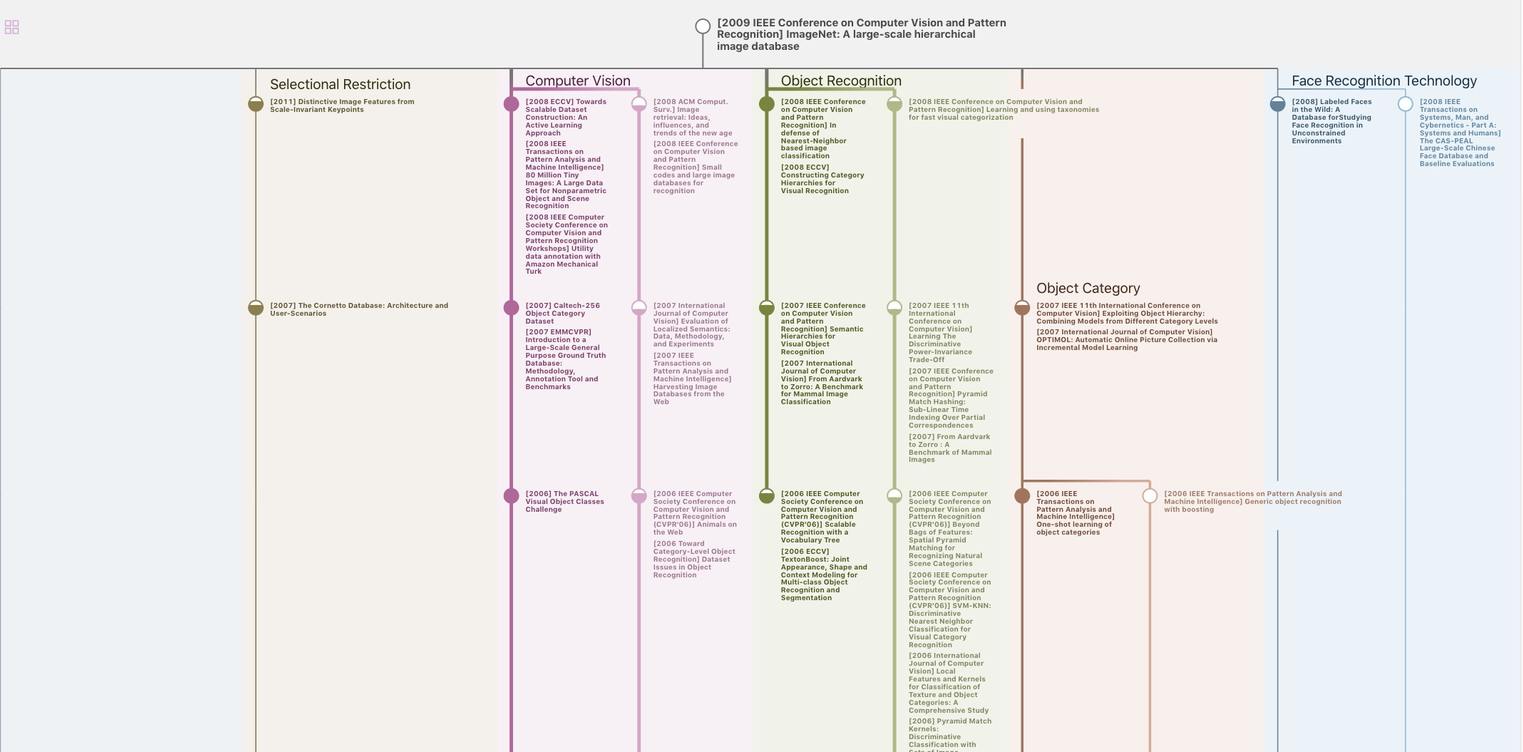
Generate MRT to find the research sequence of this paper
Chat Paper
Summary is being generated by the instructions you defined