A Learning Rule Safe From Local Minima for a Generalized Perceptron
msra(1996)
摘要
Known learning rules for the Perceptron are in some sense incomplete since no one ensures to detect non linear sep- arability, to achieve linear separability when it is possib le and to yield an optimal generalization error. We present in this paper KLR, a new learning rule for the Percep- tron derived from the Kullback Distance. Our approach consists in introducing a stochastic perturbation which al - lows us to approximate an error function by a convex one. Hence, the minimization of this function is safe from lo- cal minima. We moreover prove that KLR asymptotically learns all linearly separable sets of patterns, and that it d e- tects the non linearly separable ones. Simulations con- firm these theoretical results. They also show that KLR provides a generalization error very close to the optimal. KLR moreover yields a sturdy learning process. Fine tun- ing of parameters is not needed to obtain good results. We also show that it is possible to use KLR in order to test architecture inadequacy of classical networks as Mask Perceptron or Polynomial Classifiers (?, ?). KLR detects the non separability, hence it indicates when the considered architecture is to be upgraded. It provides an interesting information for methods of architecture devel - opment.
更多查看译文
关键词
generalization error,local minima
AI 理解论文
溯源树
样例
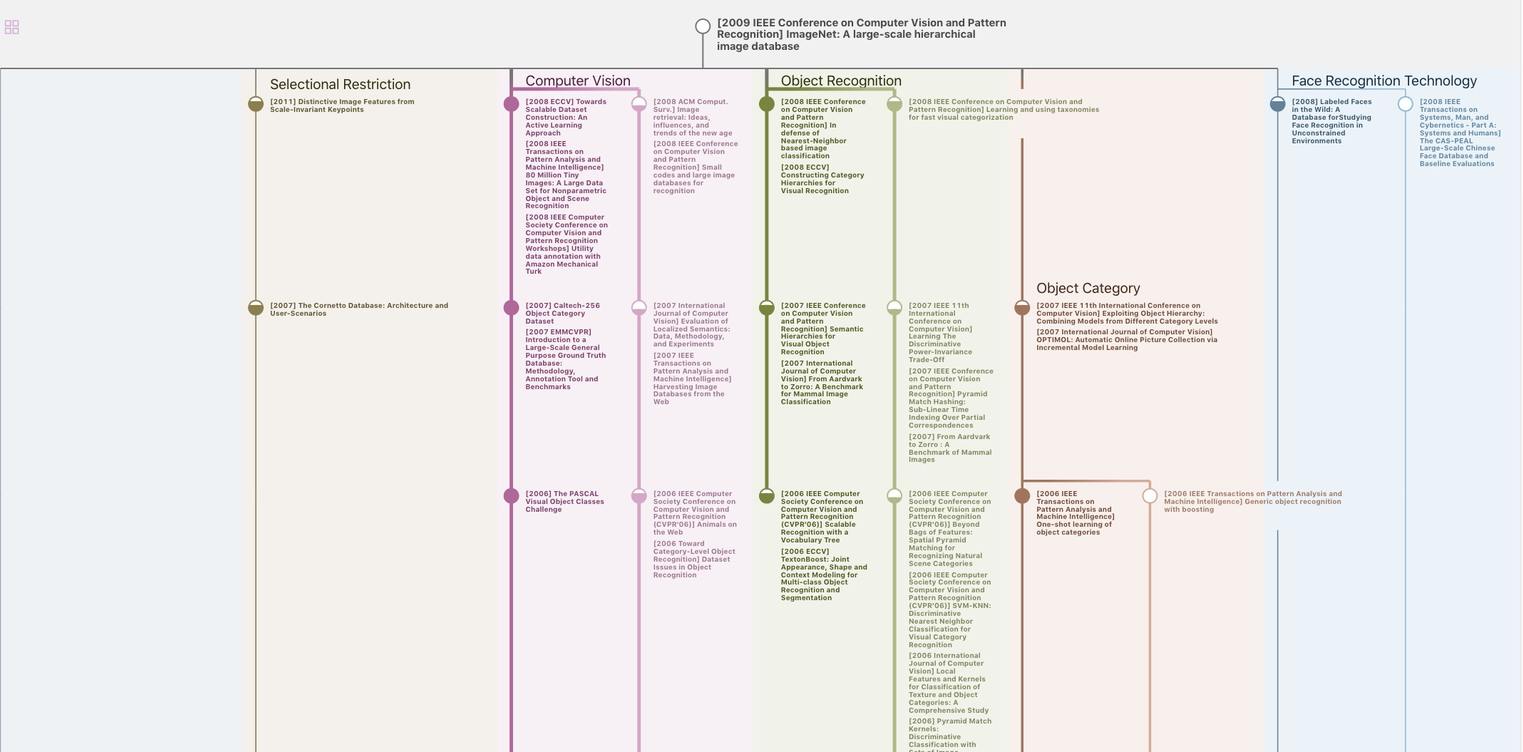
生成溯源树,研究论文发展脉络
Chat Paper
正在生成论文摘要