Estimating Photometric Redshifts With Artificial Neural Networks And Multi-Parameters
CHINESE JOURNAL OF ASTRONOMY AND ASTROPHYSICS(2007)
Abstract
We calculate photometric redshifts from the Sloan Digital Sky Survey Data Release 2 (SDSS DR2) Galaxy Sample using artificial neural networks (ANNs). Different input sets based on various parameters (e.g. magnitude, color index, flux information) are explored. Mainly, parameters from broadband photometry are utilized and their performances in red-shift prediction are compared. While any parameter may be easily incorporated in the input, our results indicate that using the dereddened magnitudes often produces more accurate photometric redshifts than using the Petrosian magnitudes or model magnitudes as input, but the model magnitudes are superior to the Petrosian magnitudes. Also, better performance results when more effective parameters are used in the training set. The method is tested on a sample of 79 346 galaxies from the SDSS DR2. When using 19 parameters based on the dereddened magnitudes, the rms error in redshift estimation is sigma(z) = 0.020184. The ANN is highly competitive tool compared to the traditional template-fitting methods when a large and representative training set is available.
MoreTranslated text
Key words
galaxies : fundamental parameters, techniques : photometric, method : data analysis
AI Read Science
Must-Reading Tree
Example
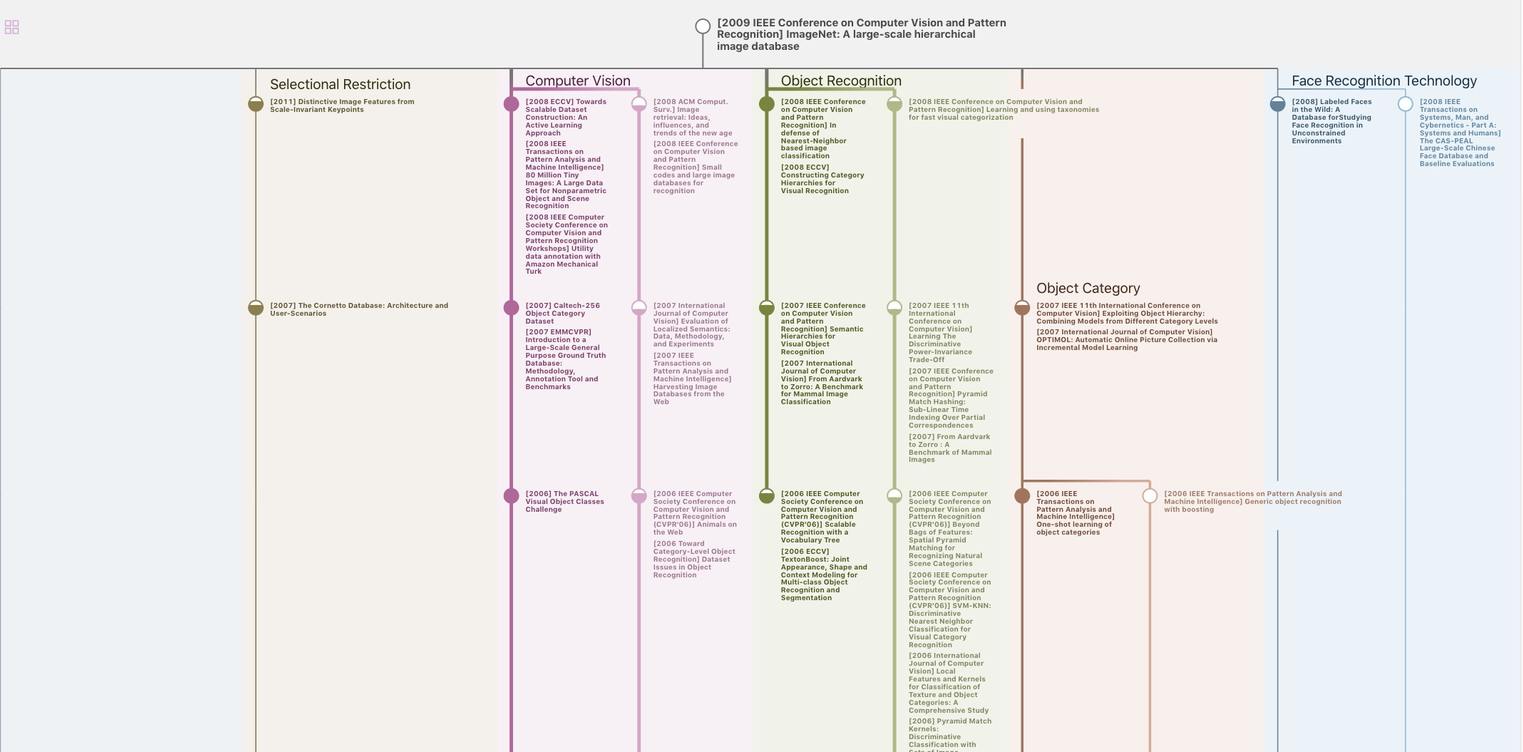
Generate MRT to find the research sequence of this paper
Chat Paper
Summary is being generated by the instructions you defined