Increasing consumers' understanding of recommender results: a preference-based hybrid algorithm with strong explanatory power.
RECSYS(2010)
摘要
ABSTRACTRecommender systems are intended to assist consumers by making choices from a large scope of items. While most recommender research focuses on improving the accuracy of recommender algorithms, this paper stresses the role of explanations for recommended items for gaining acceptance and trust. Specifically, we present a method which is capable of providing detailed explanations of recommendations while exhibiting reasonable prediction accuracy. The method models the users' ratings as a function of their utility part-worths for those item attributes which influence the users' evaluation behavior, with part-worth being estimated through a set of auxiliary regressions and constrained optimization of their results. We provide evidence that under certain conditions the proposed method is superior to established recommender approaches not only regarding its ability to provide detailed explanations but also in terms of prediction accuracy. We further show that a hybrid recommendation algorithm can rely on the content-based component for a majority of the users, switching to collaborative recommendation only for about one third of the user base.
更多查看译文
关键词
recommender results,consumers,understanding
AI 理解论文
溯源树
样例
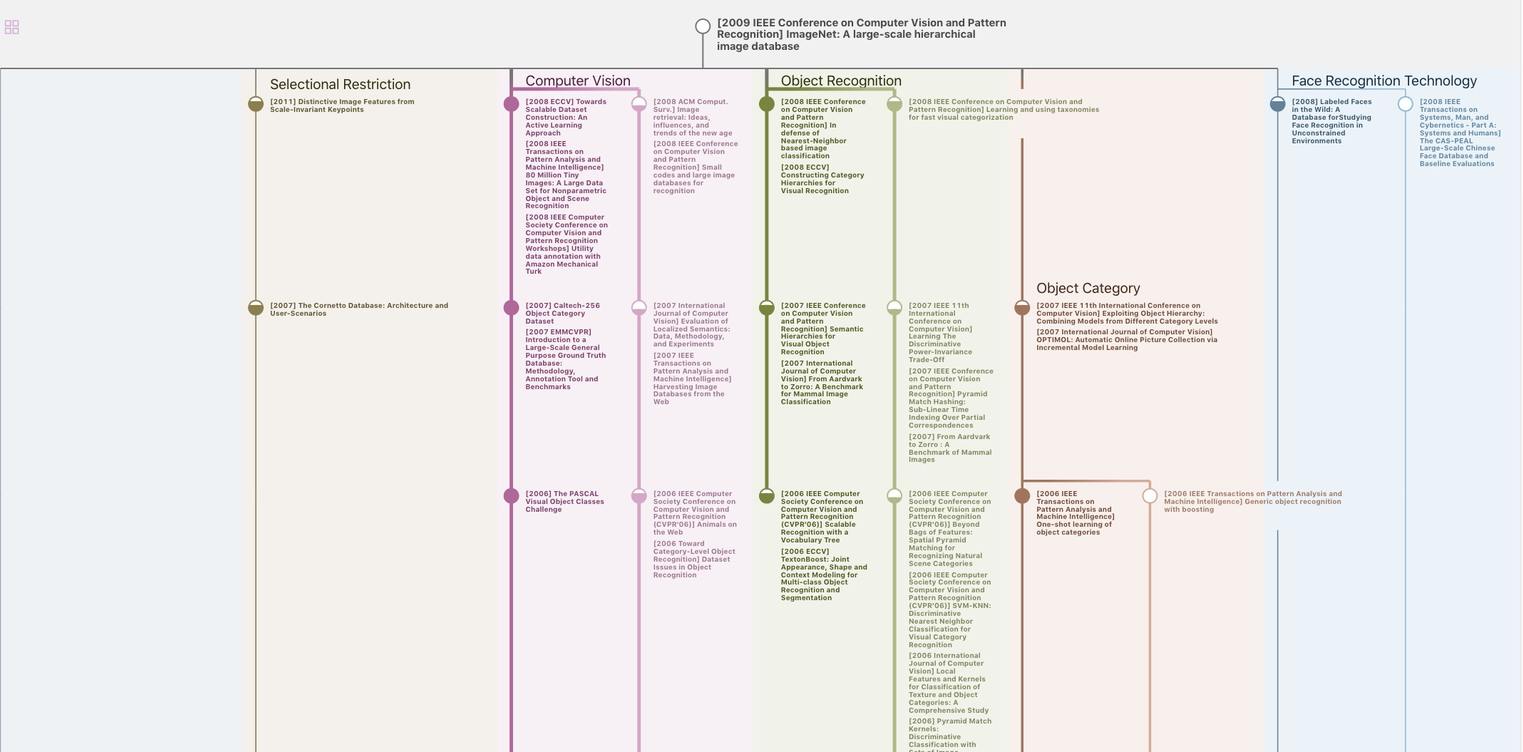
生成溯源树,研究论文发展脉络
Chat Paper
正在生成论文摘要