Developing speech recognition systems for corpus indexing under the IARPA Babel program
ICASSP(2013)
摘要
Automatic speech recognition is a core component of many applications, including keyword search. In this paper we describe experiments on acoustic modeling, language modeling, and decoding for keyword search on a Cantonese conversational telephony corpus collected as part of the IARPA Babel program. We show that acoustic modeling techniques such as the bootstrapped-and-restructured model and deep neural network acoustic model significantly outperform a state-of-the-art baseline GMM/HMM model, in terms of both recognition performance and keyword search performance, with improvements of up to 11% relative character error rate reduction and 31% relative maximum term weighted value improvement. We show that while an interpolated Model M and neural network LM improve recognition performance, they do not improve keyword search results; however, the advanced LM does reduce the size of the keyword search index. Finally, we show that a simple form of automatically adapted keyword search performs 16% better than a preindexed search system, indicating that out-of-vocabulary search is still a challenge.
更多查看译文
关键词
keyword search performance,keyword search,language modeling,neural network lm,acoustic modeling,speech recognition,interpolation,hmm model,out-of-vocabulary search,telephony,iarpa babel program,cantonese conversational telephony corpus,search problems,acoustic signal processing,model m interpolation,deep learning,relative character error rate reduction,gaussian processes,speech coding,gmm model,natural language processing,hidden markov models,bootstrap,decoding,neural nets,automatic speech recognition,lattices,acoustics
AI 理解论文
溯源树
样例
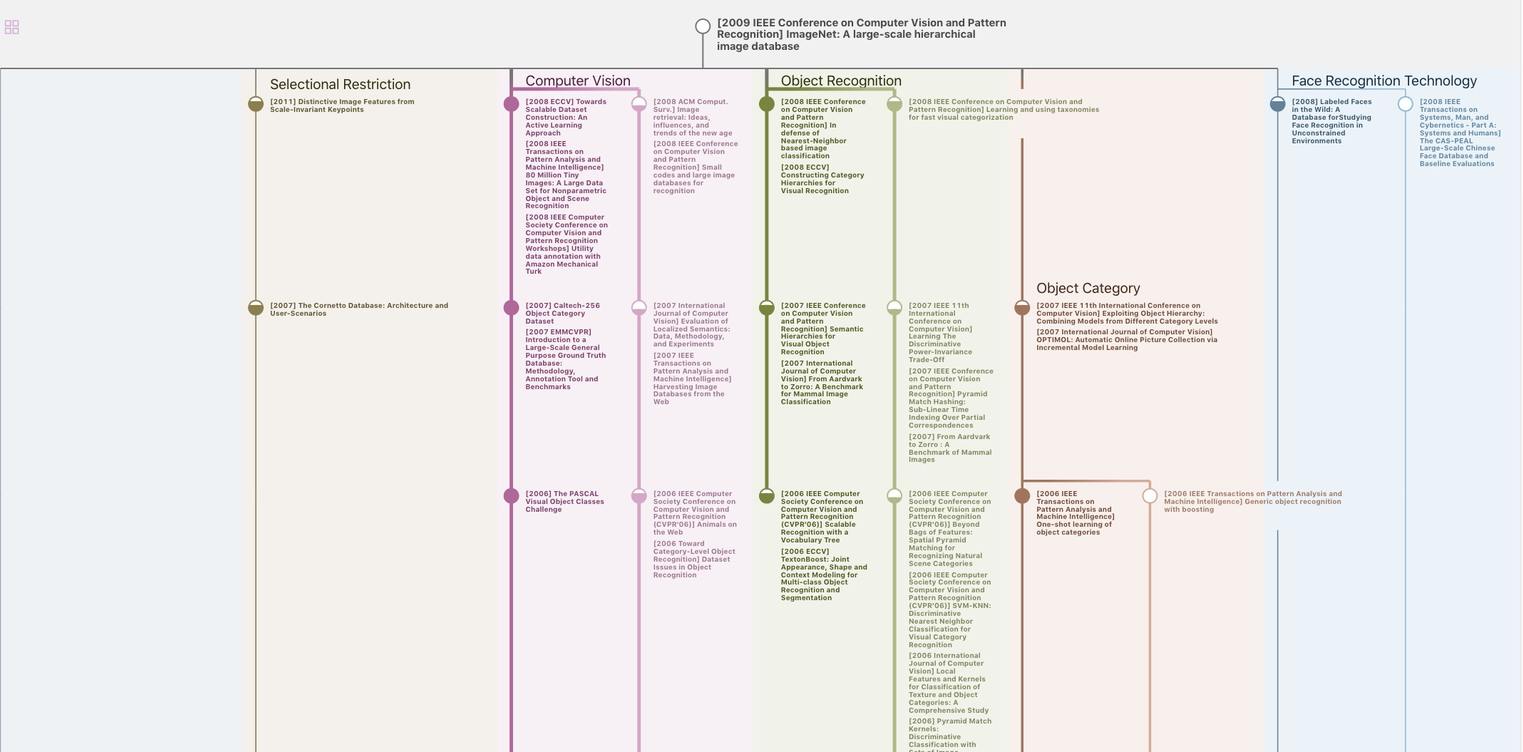
生成溯源树,研究论文发展脉络
Chat Paper
正在生成论文摘要