A study on improving training speed in support vector machine
Geoinformatics(2010)
Abstract
In a SVM classifier, the training speed is sensitive to the quantity of dataset. Therefore, the methodology of choosing some useful data that can decrease the number of training data and accelerate the training speed is usually a topic to be discussed on the SVM data process. The hyperplane of SVM is constructed by a small number of vectors. These vectors, whose locations are distributed in other kind of classes, are not only helpless for corrected classification but also increase computation loading during the training process. In addition, it may generate a weak training model for classification. In this paper, we propose a new method using ellipsoid region of n times of distribution standard deviation (σ) in feature space to choose useful data. Each ellipsoid region of class is formed by variance co-variance matrix among multi-bands of training data. After the course of choosing data, the locations on feature space of selected data are mapped into image space and these kinds of data are collected for training process. Experimental results assessment was adopted by number of selected samples, overall accuracy, and CPU time. The proposed method produces promising classification results for reducing training data analysis problems.
MoreTranslated text
Key words
variance covariance matrix,distribution standard deviation,svm data process,training process,ellipsoid region,training speed,pattern classification,data analysis,covariance matrices,svm classifier,svm,computation loading,support vector machine,classification,training data analysis problems,support vector machines,elliptic equations,ellipsoids,kernel,pixel,training data
AI Read Science
Must-Reading Tree
Example
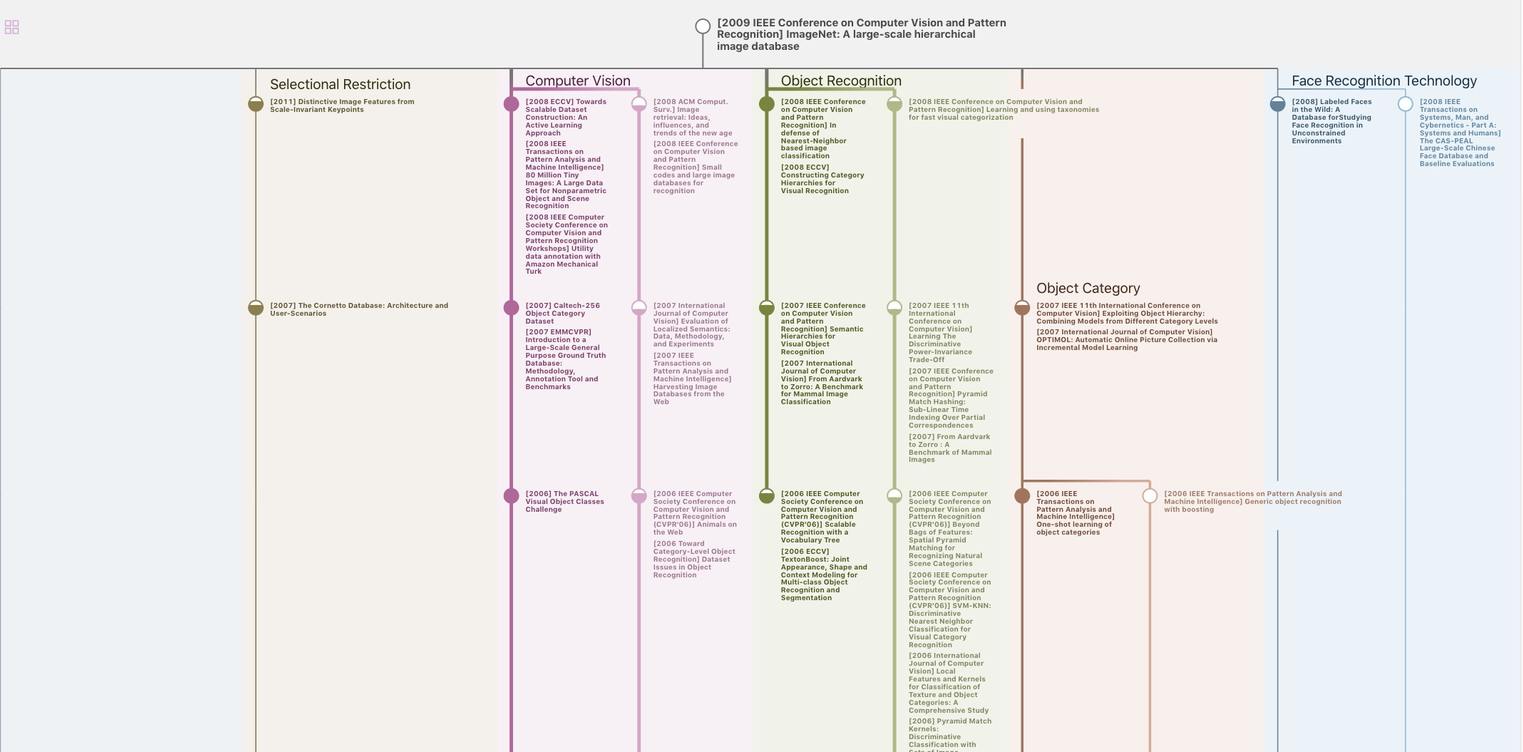
Generate MRT to find the research sequence of this paper
Chat Paper
Summary is being generated by the instructions you defined