A re-evaluation of the spawning fraction estimation procedures for Bay of Biscay anchovy, a species with short interspawning intervals
Fisheries Research(2012)
Abstract
Highlights ► Splitting the staging of histological indicators of spawning from their ageing (i.e. assignation to spawning classes), is proposed for the Bay of Biscay. For the ageing, a matrix system is defined according to knowledge regarding final oocyte maturation and degeneration of post-ovulatory follicles (POFs). The matrix provides the probabilities of examined females belonging to pre- or post-spawning daily classes, according to the time of capture. ► Several spawning fraction estimators based on the prevalence of day_0 and/or day_1 spawning classes were evaluated, from a large data set of 14 DEPM surveys, in terms of variance and bias. These properties depended on the pattern of aggregation of the daily classes and the oversampling of day 0. An alternative estimation of S , based upon the reciprocal of the individual spawning frequencies of females, produced a result highly consistent with the other S estimators, all of which were around 0.4. ► We conclude that for species with high spawning fraction (usually above 0.33), little oversampling of day_0, and/or a strong negative correlation between day_0 and day_1, S (0 + 1), the mean proportion of these two spawning classes can be considered a robust estimator, providing the most precise estimate of S for this anchovy, with negligible bias. Abstract The estimation procedures of the spawning fraction ( S ) of the Bay of Biscay anchovy ( Engraulis encrasicolus ), a species with short spawning intervals, have been revised using the samples collected in 14 surveys for the application of the daily egg production method in the period 1990–2007. Towards this aim, the procedure to assign mature females to daily spawning classes was first improved by incorporating all the available knowledge on final oocyte maturation and degeneration of post-ovulatory follicles (POFs) in a matrix system which defines the probabilities of females with either of these histological indicators belonging to pre- and post-spawning daily classes according to the time of capture. Subsequently several S estimators and their biases were evaluated: The proportion of day_0 and day_1 spawners showed a high consistency over a 24-hour cycle around the mean S value of 0.395 (CV = 18%), although some oversampling of day_0 females, up to 0.48, was observed around peak spawning time (at 23:00 hours). Individual daily spawning classes had a clumped distribution, resulting in strong negative correlation (−0.73) between day_0 and day_1 spawners. For all these features the mean proportion of day_0 and day_1 S (0 + 1), was practically unbiased and slightly more precise than the traditional S (1) corrected estimator (based upon the proportion of day_1 females, corrected for oversampling of day_0). The new estimates were very similar throughout the study period and were independent of the fishing gear or sampling time. Female size had a small but significant influence on S . In support of the high spawning fraction recorded, it is shown that many females received a double allocation to a pre and a post spawning daily class; this allowed an alternative estimation of S based upon the reciprocal of the individual spawning frequencies of females which produced a result very consistent with the other S estimators. Keywords Spawning fraction Post-ovulatory follicles Final oocyte maturation Reproduction Anchovy 1 Introduction The daily egg production method (DEPM) is currently used for estimating the spawning biomass of indeterminate spawning fish ( Stratoudakis et al., 2006 ), according to its original formula ( Lasker, 1985; Parker, 1980 ), i.e. dividing the daily egg production at sea by the population daily fecundity. Thus, adult reproductive parameters such as batch fecundity and spawning fraction; which are needed to estimate population daily fecundity, are crucial for a successful application of DEPM. Among the adult parameters, spawning fraction ( S ), or the fraction of mature females spawning per day, is probably the most relevant and, at the same time, most difficult parameter to be obtained ( Stratoudakis et al., 2006 ), requiring a time-consuming and expensive histological analysis. This parameter is usually estimated by the proportion per sample of mature females with post-ovulatory follicles (POFs) pertaining to an identifiable daily spawning class ( Hunter and Macewicz, 1985 ). Alternatively, S can also be obtained from the proportion of imminent pre-spawning females, as detected by the presence of ovaries undergoing final oocyte maturation (FOM) ( Dickerson et al., 1992; Lowerre-Barbieri et al., 1996; McBride et al., 2002; Priede and Watson, 1993; Roumillat and Brouwer, 2004; Yamada et al., 1998; Yoneda et al., 2002; Zeldis and Francis, 1998 ). However, in small pelagic fish S is rarely estimated using females undergoing FOM as those females can be often oversampled due to species spawning behavior ( Ganias, 2008 ). Consequently, there are few examples for small pelagic species where S was estimated using the proportion of imminent pre-spawning females ( Lo et al., 2005; Luo and Musick, 1991; Macewicz et al., 1996; Rogers et al., 2003; Ward et al., 2001 ). Application of the post-ovulatory follicle method for the estimation of S requires understanding the degeneration process of POFs through time, as POFs have to be aged, i.e. assigned to a daily spawning cohort (daily class) according to their degeneration state. However, many stocks lack studies on the degeneration process of POFs and, therefore, either assume or adapt former studies. In particular, the pioneer studies in aquaria relating the POF states with past daily spawning classes for northern anchovy Engraulis mordax , by Hunter and Goldberg (1980) and Hunter and Macewicz (1985) , guided most of the subsequent applications of the post-ovulatory follicle method for estimating spawning fractions of anchovies worldwide ( Cubillos et al., 2007; Dimmlich et al., 2009; Pájaro et al., 2009; Stratoudakis et al., 2006 ). Adaptation of the original description or new validations of the correspondences between POF degeneration and the daily spawning classes for other species were usually achieved by repeated sampling of schools at sea over a 24-hour cycle ( Alheit et al., 1984; Goldberg et al., 1984; Macewicz et al., 1996; Roumillat and Brouwer, 2004 ) or for several days, but by sampling at limited periods throughout the day ( Funamoto and Aoki, 2002; Ganias et al., 2003, 2007 ). In a few cases new experiments in aquaria were used to age POF degeneration ( Alday et al., 2008; Dickerson et al., 1992; Fitzhugh and Hettler, 1995; Pérez et al., 1992 ). The Bay of Biscay anchovy ( Engraulis encrasicolus ) is a batch spawner of indeterminate fecundity ( Motos, 1996 ). Santiago and Sanz (1992) noted that day_0 (actively spawning) females around peak spawning time (between 20:00 and 04:00 hours) were sampled in higher proportions than the other daily spawning classes; This is a quite typical phenomena which occurs with small pelagic fishes called oversampling of day_0 ( Alheit et al., 1984; Picquelle and Stauffer, 1985; Ganias, 2008 ). As sampling at the beginning of the series used to take place mostly at night, in order to avoid this oversampling, the day_0 females were discarded from the estimation of S . As such, since the beginning of the applications of the DEPM to the Bay of Biscay anchovy (starting in 1987, Motos et al., 2005; Santiago and Sanz, 1992; Somarakis et al., 2004 ), S was derived from the average proportion of the day_1 spawners ( Motos, 1996; Sanz et al., 1992 ), although since 1994, the day_2 spawners have also been included. Examination of the state of POF degeneration and the assignment of POF ages was directly done by an expert judgment in a single step following the descriptions of Hunter and Macewicz (1985) . This resulted in a mean S of 0.25 (ranging from 0.17 to 0.33) in May and early June, during the DEPM surveys ( Somarakis et al., 2004 ). Recently, a validation of POF degeneration stages with time was made available for this population ( Alday et al., 2008 ), indicating a faster degeneration process of POFs than previously thought. This study suggested that the spawning fraction could be higher than formerly estimated. This demanded a revision of the basis for ageing POFs, i.e. allocating them to past spawning daily classes, as well as a revision of the estimators used for spawning fraction, including here an evaluation of whether day_0 spawners could be included in estimates of S . In addition, the reproductive dynamics of fishes with medium-high spawning fractions have features of particular interest for the estimation of S , such as: (a) an increasing negative (complementary) relationship between the incidence of day_0 and day_1 spawners in the samples. This might confer some stable properties to an S estimator based on their combined incidence, given that a negative covariance should reduce the variance of a combined estimator ( Ganias et al., 2003 ). (b) An increasing number of females displaying the co-occurrence of both pre- and post-spawning stages, allowing the direct estimation of spawning frequency (or interspawning intervals) on an individual basis. This co-occurrence has been reported for many scombroids and other groups ( Dickerson et al., 1992; Lowerre-Barbieri et al., 1996; Macewicz and Hunter, 1993; McBride et al., 2002; Schaefer, 1996; Yamada et al., 1998; Yoneda et al., 2002 ) and also for some small pelagic species including Bay of Biscay anchovy ( Motos, 1996 ). The goal of this study is to review the methodology used to estimate the spawning fraction of the Bay of Biscay anchovy as an example of a species with indeterminate fecundity and short interspawning intervals. Towards this aim, our first objective is to incorporate all the available knowledge, for this anchovy, on final oocyte maturation ( Motos, 1996 , updated here) and POF degeneration ( Alday et al., 2008 ) over time, in an improved system of allocating mature females to daily spawning classes. To achieve this, a two phase procedure which splits the staging of oocyte maturation and POF degeneration from their ageing, or allocation to a daily spawning class, was adopted. For the second step, a matrix system for allocating females to daily pre/post spawning daily classes based on time of capture and stage of oocyte maturation/POF degeneration is proposed. A second objective is to evaluate alternative S estimators regarding their precision and robustness to the potential bias induced by the oversampling of active spawning females and their aggregation pattern: in total five estimators were evaluated: four estimators based on the proportions of daily spawning classes and a fifth based on the individual spawning frequencies of females. Lastly, based on the main results obtained above, the S estimates of past DEPM surveys since 1990 are revisited; along with an examination of the role that temperature or female size may play in determining the level of S . 2 Materials and methods 2.1 DEPM adult samples and processing The set of ovaries obtained for 14 DEPM surveys between 1990 and 2007 ( ICES, 2004, 2006, 2007; Motos, 1996; Motos et al., 2005; Somarakis et al., 2004 ) were reviewed, accounting for a total of 12,837 females and 503 samples ( Table 1 ). No survey was undertaken in 1993 and for some years adult samples were either not available or have not yet been analyzed. Samples of less than 8 females (10 samples) were not taken into account for the sample-based estimations and, thus, 493 samples were used for such estimates. Samples were collected throughout the day from various sources: opportunistic sampling provided by the commercial purse seine fleet, ad-hoc chartered purse seiners, or by research vessels from pelagic trawlers ( Table 1 ). Most of the purse seine samples (295) were collected at night, whilst most of pelagic trawls (208) were undertaken during the day. However, both types of gears also provided samples collected at other times of the day ( Fig. 1 ). The samples consisted of a minimum of 1 kg or 60 anchovies taken at random from the catch (including hydrated females when present) with the aim of obtaining 25 non-hydrated mature females, increasing the sample size up to a maximum of 120 anchovies when necessary in order to attain this goal. The females were immediately preserved in formaldehyde for subsequent analysis in the laboratory or processed fresh directly onboard. For each individual, total length, total weight, sex and gonad weight (in the case of females) were recorded. Ovaries were kept in a formaldehyde solution and processing followed standard histological preparation techniques ( Hunter and Macewicz, 1985 ). Lengths and weights for individuals preserved in formaldehyde were corrected for changes due to preservation by ad-hoc calculated correction factors. Sea surface temperature was available for 242 (49%) of the samples, either recorded whilst fishing, or from in situ measurements recorded ±2 days at the position of the fishing haul. 2.2 Definition of the daily spawning classes In the Bay of Biscay, anchovies spawn between 18:00 to 04:00 with a clear daily spawning peak at around 23:00 hours ( Alday et al., 2008; ICES, 2004; Motos, 1996 ). Daily spawning classes are defined for the group of females which will or have spawned the same night of a daily cycle starting and ending at 06:00 hours. A total of five daily spawning classes, relative to the day of capture, can be identified in the samples: day_−1 is the spawning class that will spawn in the night of the following day after capture; day_0 defines the group that will spawn or has spawned on the day of capture, and day_1, day_2 and day_3 are the daily spawning classes of females which have spawned one, two or three nights previously to the day of capture. In reality, day_3 is a plus group, named day_3+, which includes any female having spawned more than two nights before (grouping here mature females without any POF). 2.3 Histological indicators of spawning The first step for assigning females to a daily spawning class is to assess their gonad histological slides in terms of stage of oocyte development (stages 1–8) as well as stage of POF degeneration (stages I–VII) ( Table 2 ). Classification of oocyte development ( Table 2 ), was based on the previous works of Hunter and Macewicz (1985) and Motos (1996) . As pointed out by these authors, the stages of final oocyte maturation (FOM) which lead to ovulation (oocyte stages 5–8) develop in a day and a half ( Fig. 2 ) and provide information about the timing of the next batch. The degeneration of POFs was classified into seven stages using the criteria of Alday et al. (2008, 2010) ( Table 2 ). For the range of temperatures in the Bay of Biscay during the DEPM surveys (13–20 °C), complete resorption of the POFs occurs in about 2.5 days; therefore POF stages can identify spawning activity up to 2.5 days before capture ( Fig. 3 ). 2.4 Ageing Matrix system to assign females to daily spawning classes The second step to complete the process of determining spawning classes is to assign the former histological stages to pre-defined daily spawning classes according to the occurrence of those histological stages in time relative to peak spawning time – as summarized in Fig. 2 from Motos (1996) – updated here—for final oocyte maturation and in Fig. 3 from Alday et al. (2008) for POF stages. This was done defining an ageing matrix system which provided the probability of a female being in a daily spawning class depending on the ovary histological stage and the sampling time. A first matrix is proposed for the females undergoing final oocyte maturation (stages 5–8), which serves to allocate them to day_−1 or day_0 pre-spawning classes ( Table 3 ), whilst a second one is proposed for the classification based on the degeneration of POFs which serves to allocate females to day_0, day_1, day_2 or day_3+ post-spawning classes ( Table 4 ). As such, the double staging coupled with the ageing matrix system allowed, in principle, each female to belong to two daily spawning classes; one pre-spawning and one post-spawning. Further details follow. 2.4.1 Ageing matrix based on oocyte development stages Preparation for spawning begins with the nuclear (germinal vesicle) migration to the animal pole of the oocyte (stage 5) between 1 and 1.5 days before spawning ( Motos, 1996 ). From Fig. 2 it is evident that minimum occurrence of this stage occurs in the three 2-hour time classes from 12:00 to 18:00 hours, coincident with the maximum occurrence of the advanced nuclear migration (stage 6) and the start of its decline at 16 hours. From this we assume that all day-0 females have already entered the advance nuclear migration and hydration phases prior to spawning (stages 6–8) at 16:00. Therefore, all females having gonads with oocytes in stage 5 sampled between 16:00 and 06:00 hours, will spawn in the night of the following day and belong to day_−1; whereas females in this stage occurring between 06:00 and 12:00 hours will culminate the final oocyte maturation and will spawn on the day of capture, belonging to day_0 ( Table 3 ). In addition, the non complete disappearance of stage 5 at the maximum occurrence of stage 6, between 12 and 16:00, is probably due to the start of the early nuclear migration in females which will spawn the following day after 12.00 hours, compensating thus the departure from the stage 5 of the day_0 females to stage 6. For this reason a transition (overlapping) period of co-occurrence of day_0 females leaving stage 5 to enter stage 6 with those day_−1 just recruited to the stage 5 is allowed between 12:00 and 16:00 hours; this is reflected in Table 3 by the gradual complementary sharing percentages of stage 5 on the two consecutive daily spawning classes (day_−1 and day_0) over this period. Ovaries showing oocytes in advanced nuclear migration (stage 6) vanish around 00:00 hours ( Fig. 2 ), after which an increasing occurrence is seen as result of the recruitment from the stage 5 (initial nuclear migration). This means that ovaries in stage 6 found between 00:00 and 06:00 hours are indicative of a spawning in the following night (day_−1 spawning class), whilst stage 6 seen between 06:00 and 00:00 hours will reach spawning that day (day_0 class). Oocytes in hydration phases (Stages 7 and 8), which last less than a day, are indicative of an imminent spawning in the next few hours; they start recruitment from their earlier stages after 06:00 hours and vanish after spawning, well before the next 06:00 hours of the night of spawning, thus both stages are indicative of a spawning during the day of capture (day_0) ( Table 3 ). 2.4.2 Ageing matrix based on POFs degeneration stages Based on Alday et al. (2008) , the first four POF stages (I to IV) last less than a day, appearing in the samples before 06:00 hours (as day_0 class after the spawning) and ending during the following day (day_1) ( Fig. 3 ). As spawning starts at 18:00, just after the sharp minimum occurrence of POF stages I and II at 16:00 hours (in Alday et al., 2008 ), the occurrence of these stages between 18:00 and 06:00 hours is allocated to day_0 and between 06:00 and 18:00 hours to day_1 ( Table 4 ). For stages III and IV, a marked minimum occurrence in the field samples was detected by Alday et al. (2008) at 20:00 hours and at 00:00 hours, respectively. For these stages, the period of minimum occurrence was used to separate their allocation into successive daily classes (either to day_0, between the respective minimums and 06:00 hours, or to day_1 at the complementary part of the day) ( Table 4 ). For the older POF stages, which can last 24 hours or longer (stages V to VII), direct allocation to a single spawning class can be only applied for the periods around their maximum occurrence, when no overlap between successive daily spawning classes can occur ( Fig. 3 ). However, as these POF stages last for a day or more, some overlap of consecutive daily spawning classes may occur during the entry and exit periods in these stages (corresponding with the shaded areas in Fig. 3 ) ( Alday et al., 2008 ), given that the entry process finishes later than the exit starts. In these overlapping periods some assumptions need to be made in order to allocate these stages to spawning classes. For simplicity, symmetrical and gradual transition percentages for the allocation to daily spawning classes over the overlapping periods are proposed, as suggested in Alday et al. (2008) . For example, stage V has a maximum occurrence mainly between 12:00 and 02:00 hours ( Fig. 3 ); thus, within this period, all females with stage V POFs belong to the day_1 spawning class. However, recruitment to this stage may start at 06:00 hours, as day_1 spawners; and they may not be finished leaving this stage until 12:00 hours of the second day after spawning, as members of the day_2 spawning class ( Alday et al., 2008 ). Hence, for the three 2-hour classes between 06:00 and 12:00 hours, day_1 females recruiting to the POF stage V were given increasing percentages of occurrence within the respective classes of 25%, 50% and 75%. Complementary symmetrical decreasing percentages were for day_2 females leaving POF stage V ( Table 4 ). Finally, a similar approach was followed for the overlapping periods of the POF stages VI and VII, occurring between 14:00 and 22:00 hours and between 00:00 and 10:00 hours, respectively ( Alday et al., 2008 ): Gradually increasing percentages were set for the recruiting spawning classes to the stages (i.e. to day_1 in the case of stage VI and to day_1 and day_2, depending upon the time of capture, in the case of stage VII) ( Table 4 ). For ageing stages within overlapping periods, a random allocation of the female to one of the two potential daily spawning classes was made in proportion to the percentages set in the ageing matrices ( Tables 3 and 4 ). 2.5 Spawning fraction estimators based on the proportions of daily spawning classes Day_0 and day_1 spawning classes were selected for evaluating the spawning fraction. The other daily spawning classes were discarded: Day_−1 cannot be used as it is detected incompletely in a 24-hour cycle whilst the day_2 spawning class requires increasing assumptions for the long overlapping periods of the POF stages belonging to it (mainly stages VI and VII), in this way making its identification far more uncertain than that of day_1 ( Alday et al., 2008 ). In addition, day_2 shows an irregular occurrence throughout the day (see results) which prevents its use for the estimation of S . Finally, four estimators of the spawning fraction ( S ) based on the proportions of the day_0 and day_1 spawning classes were tested ( Table 5 ): Estimators S (0) and S (1) refer to the proportions of these two spawning classes in the samples, respectively. Estimator S (0+1) is the mean proportion of day_0 and day_1 spawning classes in the samples. And finally, estimator S (1). Corr is, in principle, similar to the original estimator proposed by Picquelle and Stauffer (1985) for the proportions of day_1 spawning class, corrected for oversampling of day_0. However, the corrected number of mature females in the denominator is not 2 n ′ 1 + n ′ 2 + as originally proposed, but n − n ′ 0 + n ′ 1 , in which the sub-indices refer to spawning classes; n refers to the total number of mature females in the sample and n ′ 2 + refer to either day_2 or day_3+ spawning classes. Both expressions are equal only if day_2+ totally excludes the day_0 females. This distinction is relevant for high spawning fractions, as there will be an increasing amount of day_2+ females (even with POFs) also belonging to the day_0 spawning class. Unless they are removed from the day_2+ spawning classes, the denominator will be inflated and thus S (1). Corr will underestimate the true S . For the purposes of clarification, we will call day_2+.Mixed the complete day_2+ group of spawning classes, whilst day_2+.Pure will be used only for the females of the day2+ group which are not spawning during the day of capture (i.e. excluding day_0 females). For %day_2+.Mixed and %day_2+.Pure we will refer to their respective mean percentage per sample (note that these are not S estimators as they do not refer to a single spawning class). In all cases the mature population upon which to base the former S estimates should comprise the spawning capable females (stages 3–8 in Table 2 ). In practice, however, as the immature females were so scarce (just 0.7% of the total population) they were allowed to join the denominator of the S estimators, resulting in S at the population level, not restricted to the mature population. Estimates of the mean spawning fraction of a group of samples (either grouped by survey, or by sampling time or by mean weight of females) and its variance were produced based upon the proxy of a sampling proportional to abundance (for a judgment sampling scheme) proposed by Picquelle and Stauffer (1985) (its Eq. (5) ), in which weighting factors per sample were set equal to 1 when the number of females m i in the sample was higher than a threshold value (20 in this paper) or equal to m i /20 otherwise. The expected variance of an estimator based on the combined occurrence of several daily spawning classes – such as S (0 + 1) – in terms of the covariance of the spawning classes, was described by Ganias et al. (2003) . Potential relationships between the individual S values and several covariates, such as sampling gear, sampling time, size of the females and the year-survey factor were tested using generalized linear models ( McCullagh and Nelder, 1989 ). 2.6 Spawning aggregation patterns, oversampling and properties of the estimators The statistical properties of the four estimators considered above, depend upon the type of aggregation and distribution of the spawning classes between schools: a clumped distribution of the daily spawning classes will tend to increase the variance in comparison to random or uniform distributions of the classes across samples. This was analyzed by correlation matrices between the proportions of the spawning classes and by the normal and standardized Morisita' s index of dispersion ( Ganias, 2008; Krebs, 1999 ). This index can be used to test if the spawning classes are distributed at random across samples by a Chi-square test ( Krebs, 1999 ). For its application, all sample sizes were homogenized ( Ganias, 2008 ) by re-scaling them to 20 females (which is an arbitrary value chosen at the 10th percentile of all available sample sizes, leading therefore in most cases to a reduction of the actual sample size and hence losing some of the potential power of the test for the amount of data available). For random distributions, the standardized Morisita index gives values of around 0, with 95% confidence limits at +0.5 and −0.5, whereas values greater than 0.5 indicate a clumped pattern and values less than −0.5 a uniform distribution pattern ( Krebs, 1999 ). In addition, these estimators will be unbiased only if no oversampling of day_0 spawners occurs throughout the day. However, if oversampling of day_0 occurs at a certain period of the day then some of the estimators would be biased for the samples collected from that period of the day. Oversampling of day_0 at a given time t means that the proportion of the day_0 females in the samples is on average higher than the expected one (i.e. than S ). In this paper, oversampling is assessed in relative terms, as the relative oversampling of day_0 throughout the day (i.e. the relative bias in the proportion of day_0 class in the samples taken at any time t, R B i a s ( S ˆ ( 0 ) t ) = ( S ˆ ( 0 ) t / S ) − 1 , with S referring to the true unbiased spawning fraction of the population – see the evaluation procedure below). As shown in Table 5 , the bias of the S estimators, based on samples taken at a certain time of the day, will depend upon the magnitude and type of this oversampling: If random oversampling of day_0 occurs, i.e. the oversampling of day_0 has a negative random effect on all remaining daily classes (proportional to their true abundance), then S (1). Corr will be the only unbiased estimator (see Appendix for demonstrations). In this case, the biases of the other estimators are proportional to the relative oversampling of day_0 at the sampling time t ( R B i a s ( S ˆ ( 0 ) t ) ) and depend also upon the actual value of S . For S < 0.5 the relative biases of S (1) and S (0+1) are smaller than the relative bias of S (0) whilst for S values above 0.25, S (0+1) is less biased than S (1) ( Fig. 4 ). Alternatively, if oversampling of day_0 has a parallel negative effect only on day_1 (repulsion type) then S (0+1) will be the only unbiased estimator ( Table 5 ). Notice that for S = 0.5 the only type of oversampling should be that of “repulsion” because the only two complementary daily spawning classes are day_0 and day_1, given that day_2 will be at the same time the day_0 spawning class. Examination of correlations between daily spawning classes clarified the type of oversampling encountered. Evaluation of the potential oversampling of day_0, which is suspected to happen mainly around spawning time, was made both visually, throughout a 24-hour cycle, and quantitatively by fitting a logistic model to the absolute oversampling as a function of the time to peak spawning time (From 15 hours prior to 15 hours after spawning time): (1) S t = S + O ⋅ exp ( B * ( I − | t | ) ) ( 1 + exp ( B * ( I − | t | ) ) where S t is the observed spawning fraction at time t , based on day_0 (or day_1 for the observations more than 7 hours after spawning time). S is the expected level of spawning fraction outside the oversampling period, O is the maximum oversampling effect, by which S can be increased on average during the oversampling period, I is the inflection time of the logistic equation, offset from the peak spawning time, for which the oversampling effect attains half of its maximum value, | t | is the time in hours (in absolute terms) from the sampling time to the peak spawning time (23:00 hours), and finally B is the parameter defining the steepness of the logistic curve. From Eq. (1) , the expected relative oversampling of day_0 at any time t would be S t / S − 1. As oversampling of day_0 changes with sampling time, the overall final bias of any of these estimators across samples will be the mean of the individual biases of the sample-estimates across all effective sampling time intervals. For example, for the case of S (0 + 1) estimator the overall relative bias will be: (2) Mean ( R B i a s ( S ˆ ( 0 + 1 ) ) ) = = ∑ t = 1 24 N t ⋅ R B i a s ( S ˆ ( 0 + 1 ) t ) ∑ t = 1 24 N t where N t is the number of samples in the time interval t , and R b i a s ( S ˆ ( 0 + 1 ) t ) is the expected relative bias at time t ( Table 5 ) as a function of S and the relative oversampling of day_0 expected at that time ( S t / S − 1). From the former equation, it follows that the bias corrected S (0 + 1) estimator over all samples will be simply: (3) S ( 0 + 1 ) . B i a s C o r r = S ( 0 + 1 ) 1 + M e a n ( R B i a s ( S ˆ ( 0 + 1 ) ) ) with variance (neglecting the errors in the mean relative bias) equal to the original variance divided by the square of the denominator. 2.7 Spawning fraction estimator based upon individual female spawning frequencies For species expected to have short spawning intervals, as for this anchovy, many of the females can receive double assignments to pre- and post-spawning daily classes which should permit an estimation of their respective spawning frequency (measured in terms of inter-spawning intervals ISI , or days passed between successive spawning events; Ganias—this volume ). This double assignment allows an estimation of S for the population based upon the average of the individual spawning fraction of females, deduced from the reciprocal of the spawning frequencies ( 1 / f ), as follows: (4) S ( f ) = ∑ f = 1 inf 1 f ⋅ P ( f ) where P ( f ) is the probability of a female having a spawning frequency of f days. With variance: (5) Var ( S ( f ) ) = ∑ f = 1 inf 1 f 2 ⋅ Var ( P ( f ) ) + 2 ⋅ ∑ f = 1 inf ∑ f ' < f inf 1 f ⋅ 1 f ′ ⋅ C o v ( P ( f ) , P ( f ′ ) ) where f and f ′ refer to different spawning frequencies. In our case, we restricted the expression to the first three daily interspawning intervals, i.e. for f ranging from 1 to 3, assuming that all day_3+ spawned during the night of the third day after their previous spawning ( Table 5 ). Under these circumstances, the estimator is simplified to: (6) S ( f ) = 1 ⋅ P ( f = 1 ) + 0.5 ⋅ P ( f = 2 ) + 0.333 ⋅ P ( f = 3 ) where P ( f = 1) is the probability of a female spawning every day and was estimated as the frequency of day_0 within the day_1 spawning females P ( f = 1| day_1) = P (day_0 | day_1). P ( f = 2) is the probability of a female spawning every other day and was estimated as [1 − P ( f = 1)] · P ( f = 2) | day_2), where P ( f = 2 | day_2) = P (day_0 | day_2) was the probability of a female of the day_2 class of spawning again in that day (i.e. in the second night after their previous spawning). This was estimated by the frequency of day_2 females also being day_0 females, as estimated during the first part of the day (from 06:00 to 17:59 hours). The selection of the first half of the day_2 for estimating the proportion of day_0 females within it was because in this way the spawning period was excluded totally and enabled day_0 to be identified completely. Finally, P ( f = 3) is the probability of past-spawning females spawning every third day, which was estimated as [1 − P ( f = 1)] · [1 − P ( f = 2 | day_2)], where [1 − P ( f = 2 | day_2)] was the probability of a female of the day_2 spawning class spawning during the following night (i.e. during the third night after their previous spawning). Using the P ( f = 2 | day_2) complement avoided having to make direct use of the most likely noisy direct proportion estimates of day_3 females. Notice that in this way a covariance term between P ( f = 2) and P ( f = 3) was generated. Given the simplifications assumed to apply this formula and the little variability in the S estimates between years arising from the estimators based on the prevalence of daily spawning classes (see results), we applied this formula to the pooled set of females across the years (not on a survey by survey basis) in an exploratory way, just for the purposes of verification and discussion. 3 Results The spawning dynamics of actively spawning females in the population throughout a five-day period is shown in Fig. 5 a as the proportion of each daily spawning class in the population, overlaying the relative contribution to each class of the females in a particular ovary maturity stage according to the development of their oocytes. There is an overall consistency of the proportions of day_0 and day_1 spawning classes at around 40%. The incomplete detection of the day_-1 is clearly associated with the early nucleus migration in oocytes during the second half of the day (stage 5). Day_0 females clearly show the progression from early nucleus migration to the hydration stages. Day_0 – after spawning – and day_1 females were composed of females with ovaries showing in majority yolked oocytes (stage 4), although at the end of day_1 more females with oocytes in early nuclear migration (stage 5) were observed. The percentages for day_2 were more irregular than for the former daily classes, being greater than 40% for the first half of the day and markedly lower from 18:00 hours onwards. This endorsed the rejection of this spawning class for the estimation of S . The initial higher proportion of day_2 might be due to potential ageing confusion with the day_3 spawners across the overlapping period of POF stage VII. The subsequent decrease was related to the advanced maturity stages of the ovary of many of these females (with oocytes in nuclear migration or hydrated), indicating that most of the day_2 females may resume spawning that night. Similarly, when looking at the occurrence of POF stages by daily classes ( Fig. 5 b), it can be observed that most day_0 females prior to spawning showed ovaries with POF stages VI and VII, typical of the day_2 spawning class. Most of the identified day_3+ females had no POFs but the oocytes were undergoing FOM. The very few immature or developing females appeared grouped in the day_3+ spawning class. Fig. 6 shows some potential oversampling of actively spawning females (day_0) around peak spawning time, as indicated by a rise in the average S (0) to a maximum average value of 0.485, i.e. an increase above the overall mean S of 0.39–0.4. Conversely, some undersampling of the day_1 appears to be apparent around peak spawning time. As the variance of S (0), 5 hours either side of spawning time, was higher than outside these periods (Cochran's C test: 0.6189, P < 0.001), we performed a Kruskal-Wallis test to compare the median S estimate presented in Fig. 6 , grouping them 5 hours either side of the spawning time (at 23:00 hours), 5 hours either side of the following spawning event (24 hours later) and all remaining estimates outside these time ranges. The medians of the S (0) and S (1) estimates 5 hours either side of the peak spawning times were higher and lower, respectively, than the medians of the S (0) and S (1) estimates outside these periods ( P = 0.001 and 0.062, respectively). The logistic equation fitted to the oversampling of day_0 on the S estimates, 15 hours either side of the peak spawning time, showed a dome-shaped curve ( Fig. 6 ), defining a rise in spawning frequencies of about 0.067 (parameter O , S.E. = 0.021) over the normal S estimate ( S = 0.391, S.E. = 0.012). The oversampling phenomena lasts for about 10 hours (5 hours either side of the peak spawning time, as pointed out by the inflection point I = 4.938 hours, with S.E. = 0.80, and by the curvature parameter B = 1.952, with S.E. = 3.37). The correlations of S (0) with S (1) and with the percentage of occurrence of all remaining classes (%day_2+.Pure) were significantly negative for all times of the day ( Table 6 ), but reaching the maximum around spawning time. Estimator S (1) however, was positively but non significantly correlated with the %day_2+.Pure estimator around spawning time. These observations suggest that oversampling of day_0 negatively affects all of the remaining spawning classes, as corresponds with a random type of oversampling of day_0. The Chi-square values for the Morisita index ( Table 7 ), led to the rejection of the random distribution of spawning classes across samples, except for the joint day(0 + 1) classes (with P = 1). The standardized Morisita index revealed that the individual spawning classes (day_0 and day_1), as the remaining classes (day_2+), tended to show clumped (aggregated) distributions (with values higher than 0.5), whilst day(0+1) tended towards a uniform distribution (with a value lower than −0.5). These aggregation patterns were consistent for all times of the day. The variability of the individual spawning fraction estimates between samples obtained from the direct estimators S (0) and S (1) was very high, ranging between 0 and 1, whilst it decreased sharply for S (1). Corr and S (0 + 1) ( Figs. 6 and 7 , respectively). The latter estimator provided the least variable estimates as most of the very low S values, which appeared in the S (1). Corr estimates, disappeared. The associated coefficients of variation of the pooled estimates (across all samples of all surveys) were high for S (0) and S (1) (CV of 50% and 45%, respectively), lower for the S (1). Corr estimator (CV = 22%) and lowest for the S (0 + 1) estimator (CV = 18%) ( Table 8 ), with the latter being significantly lower than the former ( F = 1.33034 P -value = 0.0016). The survey-based estimates of S ( Table 8 ) revealed low standard errors and low variability in the spawning fraction between surveys and years, around an overall mean of 0.39 (CV = 6%) for S (1). Corr and of 0.40 (CV = 4.5%) for S (0 + 1). The estimates did not significantly differ between years. The Mean relative bias of the estimates by surveys, for the random type of negative effect of the oversampling of day_0, were minimal for S (0 + 1), intermediate for S (1) and maximal for S (0) ( Table 8 ). Application of these mean relative biases to correct the original estimates, produced revisions closer to the S (1). Corr estimates in 9 out of 14 surveys for the S (0) and S (0 + 1) estimators and in 7 cases for the S (1) estimator. Concerning the estimations based upon spawning frequency of individual females, the probability of a female spawning every day, P ( f = 1), was almost negligible, about 0.7% (34 out of 4882 females). The value of P ( f = 2 | day_2) was 60.49% (for 2779 females), and thus P ( f = 2), the probability of a female spawning every other day, was 60.07%. Consequently the complementary probability of past-spawning females spawning every third day was 39.24%. Hence, the overall estimation of S ( f ), based upon the inter-spawning intervals of individual females, was 0.438 with a very low coefficient of variation (CV) of about 0.4% when using a binomial approach, which rose to about 0.8% when variation among samples in the conditional probabilities were included. The small CVs are due to the large number of females (7661) and samples used in the estimation. Sea surface temperature has a weak but significant relationship with the S (0) and S (1) estimators with opposite signs ( Table 9 ). But, as no significant correlation was observed with the S (1). Corr or with S (0 + 1), the role of temperature on S was discarded. Sex ratio was negatively related to the S (0), S (1). Corr and S (0 + 1), whilst mean weight of females was positively related to S (1). Corr and S (0 + 1). The fitting of the spawning fraction (with logistic regressions) as a function of the survey, sampling gear, sampling time and mean weight of females showed that sampling gear did not affect any of the S estimators ( Table 10 ). Estimates of S (0) changed significantly with survey and sampling time; the latter was consistent with the oversampling phenomena described above. On the other hand, the estimate of S (1). Corr . depended on the weight of females and on sampling time, but not on the survey, and finally S (0 + 1) only depended on the weight of females. Sampling time was not significant in the full model for S (0 + 1) and the subsequent removal of survey and sampling gear from the analysis of S (0 + 1) confirmed its non-significant role (final P = 0.0726). For the S (1). Corr estimator, the marginal significance of sampling time arose from the 06:00 to 08:00 hours class (see the means in Fig. 7 a). When this time class was removed from the model, sampling time became non-significant for S (1). Corr . The mean weight of females had a small but significantly positive effect on S (1). Corr and S (0 + 1) estimates ( Fig. 8 ). Nevertheless, most of the variability in S remained unexplained, as the logistic models fitted above only explained 7.5% of the original deviance of the data. 4 Discussion The anchovy in the Bay of Biscay display a fast spawning dynamic, with about 99% of the population being mature – spawning capable – in May–June ( Motos, 1996 ), and with most of the anchovies spawning every other day and the remainder probably every third day. This corresponds with a spawning fraction around 0.4, which is about 60% higher than the previous average estimates reported for this population ( ICES, 2006, 2007; Motos, 1996; Somarakis et al., 2004 ). The current revision of the spawning fraction was made possible due to the biological knowledge behind the definition of the ageing (stage-spawning class) matrix system. Understanding the dynamics of final oocyte maturation and of the degeneration of POFs in time, permitted reliable and complete identification of the two retained daily spawning classes for the estimation of spawning fraction, day_0 and day_1. The validity of the present S estimates, and hence of the ageing matrix system, was supported by the consistency of the relative proportions of day_0 and day_1 spawning classes (around 0.4) throughout most of the day (with the exception of the hours around peak spawning time, Fig. 5 ). This indicated roughly similar estimates of the spawning fractions S (0) and S (1), as should be expected. Discrepancies with the proportion of the day_2 spawning class for parts of the day (in Fig. 5 ), which indicated a higher S for the first half of the day and a lower S during the evening and night, were attributed to the potential confusion with the day_3 spawning class, as reported for other species ( Cubillos et al., 2007 ), and to the recruitment to the next spawning, respectively. The former feature would be related to the ageing uncertainties throughout the long overlapping periods of the POF stages defining this class, whilst the latter was evident due to the fact that most of the females showing POFs of the day_2 spawning class had oocytes undergoing final maturation which corresponded with the day_0 pre-spawning class (resuming the spawning in that night). Additional support for the current S estimates comes from the estimation of spawning fraction based on the individual spawning frequency of females which resulted in a value ( S ( f ) = 0.438) very close to those produced by the standard methods based upon the proportions of day_0 and day_1 spawning classes. The slightly higher S value produced from the individual spawning frequency of females compared to the standard estimates can be understood by some violation of the assumptions that ageing of day 2 was accurate and that all females will spawn again in at least every three days. If some of the identified day_2 females (in POF stage VII) were in fact day_3, or if some of the day_3 (with no POFs) females in fact belonged to the day_4, this would imply some reduction of the S ( f ) estimate, leading to a closer result. One of the reasons for the current S estimates of supposing an upward correction over the previous estimates is due to the revision of the degeneration rates of POFs for this anchovy, being somewhat faster than those for the northern anchovy ( Alday et al., 2008 ). However, this difference was also originated partly from undue or subjective errors in the direct allocation of a large variety of POFs to daily spawning classes, as practiced in the past. The current matrix system for ageing ovaries stages based on their oocytes and POFs is particularly well designed for cases when the collection of adult samples from several sources provides samples from throughout the day. This sampling strategy increases the variety of ovary stages, based on oocyte development or POF degeneration, that can be allocated to the same daily spawning classes and requires a good deal of expertise if staging and ageing are made simultaneously. Separating ovary staging from the ageing makes the process more objective, as staging only describes the histological features of the ovary whilst ageing only assigns ovary stages to spawning classes by an automated procedure based upon biological knowledge. The process then becomes more repeatable for subsequent cross-checking of ovary (oocyte and POF) stages and for any potential future revision of the stage-spawning class matrices according to new ageing criteria. Hence, we particularly recommend this type of approach for cases when adult samples are obtained throughout the day. In addition, given the important role that the validation of the degeneration of anchovy POFs in temperate waters has played in this review, we recommend: (a) that POF degeneration is validated for the range of temperatures each stock inhabits; and (b) that the results of Alday et al. (2008) for the same anchovy species spawning in a similar range of temperatures are used when validation is not feasible. In comparison to other species, the present spawning fraction estimates were, in general, higher than those estimated for other Engraulidae stocks in temperate waters (with SST between 12 and 20 °C), which rarely exceeded 33% ( Alheit et al., 1984; Armstrong et al., 1988; Cubillos et al., 2007; Dimmlich et al., 2009; Hampton, 1996; Hunter and Macewicz, 1985; Pájaro et al., 2009; Somarakis et al., 2004 ). Nevertheless, in areas with warmer waters (ranging between 15 and 25 °C), the spawning fraction may be as high as 50%, or even higher ( Clarke, 1987; Funamoto and Aoki, 2002; Funamoto et al., 2004; Luo and Musick, 1991; Rogers et al., 2003; Takasuka et al., 2005; Wright, 1992 ). Therefore, the high spawning frequencies found for the Bay of Biscay anchovy are compatible with the biology of Engraulidae and Clupeidae. Many of these species can be considered as “income breeders” which obtain the required energy to sustain high spawning frequencies from feeding prior to and during the spawning period ( Clarke, 1987; Ganias, 2009; Luo and Musick, 1991; Maack and George, 1999; Somarakis, 2005; Somarakis et al., 2000; Wang and Houde, 1994 ). This anchovy displays spawning behavior that leads to the oversampling of the most active (day_0) females; but the amount of oversampling is low, adding on average about 0.07 to the normal S , 5 hours either side of the peak spawning time (23:00 hours) ( Fig. 6 ). The start of the oversampling of day_0, around 18:00, coincides with the period when the great majority of day_0 s have completed hydration (ovary stage 8) and finishes around 04:00 hours when the majority of POF degeneration reaches stage II. This oversampling is less intense than previously perceived by Santiago and Sanz (1992) and lower than that reported for most of the Engraulidae (see review in Ganias, 2008 , which suggest an incidence of day_0 of 3 times day_1 for Engraulidae and Cupleidae). However it is consistent with some sardine stocks for which oversampling is negligible, or does not exist, such as the Iberian Sardine ( García et al., 1992 ), California sardine ( Lo et al., 2005; Macewicz et al., 1996 ) and Australian sardine ( Ward et al., 2001 ). In the case of the Bay of Biscay anchovy, as with many other species, oversampling of day_0 is a result of the ephemeral spawning aggregation of fish, by which spawning takes place in differentiated spawning schools, with the most actively spawning females accompanied by a larger group of males ( Ganias, 2008 ). This aggregation is reflected in the skewed sex ratios of these schools, as the higher the fraction of actively spawning females, the lower the sex ratio in the sample ( r = −0.29, P < 0.001, Table 9 ), and in the clumped distribution of day_0 females, i.e. day_0 segregates from the rest of the spawning classes. However, contrary to other studies ( Alheit et al., 1984; Ganias, 2008 ), in the case of the Bay of Biscay anchovy, clumped distributions were also shown by all spawning classes all day round ( Table 7 ). This means that all of the schools sampled were structured according to the spawning condition of the anchovies, so random distributions of the remaining (other than day_0) spawning classes were not seen within these schools. This is probably a feature characteristic of populations with high spawning fraction, as this makes all (pre and post) spawning classes highly complementary; This leads to the strong negative correlations between daily spawning classes detected for all classes in our study ( Table 6 ). The exception between day_0 ( S (0) and %day_2+.Mixed was certainly due to the partial overlapping of these two classes (as many of the day_2+.Mixed were also day_0). The clumped distribution of the spawning classes explains the wide variances of the S estimators based on single daily spawning classes ( S (0) and S (1)). In addition, the parallel strong negative correlation resulting between day_0 and day_1 explains why the joint distribution of these two classes approached a uniform distribution (according to the standardized Morisita index of dispersion) as each one compensates for the deviations from a random distribution of the other. Similarly this also explains the strong reduction of variance resulting for the joint S (0+1) estimator. In fact, the estimate of variance of S (0 + 1) (0.052) matched perfectly with the one expected for an estimator of S based on a combination of two daily spawning classes ( Ganias et al., 2003 ) in which the negative covariance of the two daily classes is to be subtracted twice from the addition of the individual variances (resulting in a variance of 0.053). The low variance of S (1). Corr is harder to understand as it is based on a single spawning class. Therefore, this low variance must be due to the mathematical correction of the denominator (substitution of day_0 by day_1 females to account for the oversampling of day_0). Nevertheless S (0 + 1) was a slightly more precise estimator of spawning fraction than S (1). Corr . The negative correlations between S (0) and S (1) and between S (0) and %day_2 + .Pure peaked and became relatively similar around the spawning time. This implies that oversampling of day_0 was made at the expense of all of the remaining daily classes approximately in proportion to their abundances. This corresponds with the random type of oversampling of day_0, based on all of the other spawning classes, for which the unbiased estimator is only S (1). Corr . However, in this case S (0+1) was, in practical terms, almost unbiased, with very low relative bias estimates by surveys. For instance, the relative bias of S (0 + 1) for the whole set of historical samples was 1.5% of the true S ( Table 8 ); this means a very small absolute bias of just 0.006. Thus, from the pooled estimate of S (0 + 1) of 0.401, the unbiased estimate of S was 0.395, a value very close to that given by S (1). Corr , of 0.390, which implies a negligible difference considering the variability of the estimates. Such a small bias was due, as predicted by Fig. 4 , to the moderate oversampling of day_0 at peak spawning time and to the high value of S . In addition oversampling (and hence bias) was limited to 10 out of the 24 hours of the day. The high S necessarily implied that the oversampling of day_0 spawners was mostly reflected (in absolute terms) in the undersampling of day_1 spawners as they were the only two non-overlapping daily classes in the population; this largely compensated for their relative biases around spawning time and made the estimator S (0 + 1) almost entirely unbiased. Another way of looking at this is that the closer S is to 0.5 the closer S (0 + 1) is to becoming the only unbiased estimator in case of oversampling, as for S = 0.5 the only potential type of oversampling is that of repulsion. Therefore, we conclude that for species which have a high spawning fraction (usually above 0.33, as many small pelagic species – including scombroids – in temperate and tropical areas), little oversampling of day_0, and/or a strong negative correlation between day_0 and day_1, S (0 + 1) can be considered as a robust and simple estimator of S . This produced the most precise estimator of S with a negligible bias which can ultimately be corrected according to the formulae presented before. Note that in addition the S (0 + 1) is robust to overlapping of day_0 and day_1 classes, whenever those females are included in both spawning classes. On the other hand, S (1). Corr was unbiased, given the random type of oversampling, but a bit less precise and neglected a great part of the information from the samples (day_0 class) in its estimate, whilst S (0 + 1) makes direct use of all available information without any correction. Including day_0 (advanced maturity stages and recent POFs) and day_1 in the spawning fraction estimates is a practice not often followed, although several examples are found in the literature ( Dickerson et al., 1992; Lowerre-Barbieri et al., 1996; Macewicz et al., 1996; McBride et al., 2002; Roumillat and Brouwer, 2004; Schaefer, 1996; Taylor et al., 1998; Yamada et al., 1998; Ward et al., 2001 ). Given the benefits of the joint use of these two daily spawning classes for species of high spawning fractions, S (0 + 1) (corrected for bias if necessary) may be the preferred estimator to be used in this type of circumstance. The estimates of S produced by S (1). Corr and S (0 + 1) estimators were independent of sampling gear and of sampling time, except for the 06:00 hour class in the case of S (1). Corr , which showed a lower S . This exception might be related to an incorrect allocation to daily classes in the overlapping period of the POF stage V, in that hour class. Certainly, if recruitment of day 1 to stage V was more abrupt than proposed here in the overlapping 2-hour class range, the difference in S in the 06:00 hour class would be reduced. In addition, the S estimates showed a great stability over the years with only a slight sensitivity to the mean weight of mature females. This contrasts with the results of most of the applications on other Engraulidae and Clupeidae populations where the spawning fraction was shown to vary remarkably between years and areas ( Shelton et al., 1993; Somarakis et al., 2004; Stratoudakis et al., 2006 ), or as a function of female mean weight ( Claramunt et al., 2007; Ganias, 2008; Ganias et al., 2003 ). For example, Claramunt et al. (2007) showed an expected relative increase in spawning fraction for Engraulidae of about +50% for females increasing from 20 g to 30 g, whilst our relationship suggested a minor change of about 7.5%. This lack of sensitivity is congruent with the almost invariant S across the years, despite oscillations in the proportion of one-year old fish in the population throughout this period ( ICES, 2009 ). From an evolutionary point of view, this result can be expected for a short living species with very little survivorship at the age of 2 because in this case optimum spawning capacity should not be delayed later than one-year-old fish. All these results suggest that during the season of the DEPM applications in the Bay of Biscay (May/early June), the spawning fraction was rather constant, supporting the idea of the “biorhythm hypothesis” postulated by Hunter and Lo (1997) , which states that the spawning fraction could be a rather constant reproductive feature for mature females in similar habitat conditions. In any case, our results suggest that refinements in the ageing of POFs, either by a better comprehension of their degeneration over time or by separating the staging of POFs from their ageing process, reduces the variability of the different spawning fraction estimates between years and surveys. Finally, this study endorses other possibilities for estimating the spawning fraction, such as from a Bayesian perspective under the null hypothesis of a somewhat invariant S throughout time. Monitoring of the spawning populations of pelagic fish stocks through the DEPM requires precise biological knowledge of the reproductive biology and dynamics of the spawning of stocks for survey design and parameter estimation ( Lasker, 1985; Stratoudakis et al., 2006 ). This study exemplifies how good knowledge on the timing of final oocyte maturation and degeneration of POFs can result in improved assignation of actively spawning females to pre and post spawning classes and leads ultimately to a revision of the spawning fraction estimates for DEPM applications At the same time, this study demonstrates the fast spawning dynamics of this anchovy (high S , strong aggregative pattern of daily spawning classes and oversampling of active spawners); which has helped in the understanding of statistical properties of the S estimators (variance and bias) and selection of the optimal estimators ( S (1). Corr and S (0 + 1)). Finally the methods presented here have allowed an alternative estimation of S based upon the individual spawning frequencies of females, which can be of particular interest for species with fast spawning dynamics. Acknowledgments This paper is contribution no. 513 from AZTI-Tecnalia (Marine Research). This study was funded by the Department of Agriculture, Fishing and Food of the Basque Government , through projects on the application of the DEPM (1990–2009) and by the European Commission UNCOVER project (Project no. 022717 (SSP 8)). Financial support to the second author of this study was provided by a grant from the Agricultural and Fisheries Department of the Basque Country (2005–2007). We thank Inmaculada Martín and Udane Martinez, laboratory technicians from AZTI Foundation, for their collaboration in the histological examination of gonad samples and Jacques Massé (and Ifremer) for providing us with samples collected from pelagic trawling during their acoustic surveys undertaken in 1994, 1997–1998 and 2001–2004. We also thank Professor Michael Collins (NOCS, UK and AZTI-Tecnalia) and Dr. Paul de Bruyn for editorial comments and the English revision of the manuscript, as well as to the members of the ICES WGACEGG study group for helpful discussions. Dr. Susan Lowerre-Barbieri and Dr. Hilario Múrua and three anonymous reviewers are thanked for helpful comments for improving the paper, as well as Leire Ibaibarriaga for her help with statistical analysis. Appendix A The bias of the S estimators at a sampling time t It is shown here how the bias of the different S estimators from samples taken at a given time of the day (in Table 5 ) will depend upon the amount of oversampling of day_0 existing at that time, the type of this oversampling and on the actual value of the spawning fraction of the population S . Given a sample of size n taken at time t , let n ′ 0 , n ′ 1 and n ′ 2 + denote the observed number of individuals pertaining to day_0, day_1 and day_2+ in the sample, respectively, and n ˆ ′ 0 , n ˆ ′ 1 and n ˆ ′ 2 + the expected numbers of individuals of those spawning classes which should have been taken in the sample according the expected mean oversampling of the day_0 at that time. Furthermore, let n 0 , n 1 and n 2+ denote the expected numbers of individuals of those spawning classes which should have been taken in the sample if there was no oversampling of the day_0. And finally Let ϕ t denote the average oversampling (in absolute numbers) of day_0 individuals at sampling time t . From this it follows that the expected number of day_0 individuals in the sample ( n ˆ ' 0 ) is n 0 + ϕ t (with n 0 = S n ) and the actual observation n ' 0 will be a number from the binomial distribution B ( ( n ˆ ′ 0 / n ) , n ) . In all cases spawning classes should exclude from one another and add up to n so that n = ∑ i = 0 2 + n i = ∑ i = 0 2 + n ′ i , ∑ i = 0 2 + n ˆ ′ i , and day_0 and day_1 should be completely enumerated at the expense of overlapping with day_2+ (so day_2+ excludes those being at the same time day_0 or day_1). The expected bias, at time t , of the spawning fraction estimators considered in this paper will depend upon the oversampling type: (a) When the oversampling of day_0 has a negative random effect on all remaining daily spawning classes ( n 1 and n 2+ ) the undersampling of the latter classes will be proportional to their true relative abundance. In this way, the expected sampled day_1 individuals ( n ˆ ′ 1 ) at time t will be n ˆ ′ 1 = n 1 − ϕ t n 1 n 1 + n 2 + = n 1 − ϕ t n 1 n − n 0 = n 1 − ϕ t S 1 − S That last equality follows from the definition of S = n 0 / n = n 1 / n . For each sample estimator of S we will have the following expected estimate ( S ˆ ( * ) t ) and relative bias: S ˆ ( 0 ) t = n ˆ ′ 0 n = n 0 + ϕ t n = S + ϕ t n with relative bias: (A.1) R b i a s ( S ˆ ( 0 ) t ) = S ˆ ( 0 ) t S − 1 = ϕ t S · n = ϕ t n 0 S ˆ ( 0 ) t = n ˆ ′ 0 n = n 0 + ϕ t n = S + ϕ t n with relative bias: (A.2) R b i a s ( S ˆ ( 1 ) t ) = S ˆ ( 1 ) t S − 1 = − ϕ t S · n S 1 − S = − R b i a s ( S ˆ ( 0 ) t ) S 1 − S S ˆ ( 1 ) t . C o r r = n ˆ ′ 1 n − n ˆ ′ 0 + n ˆ ′ 1 = n 1 − ϕ t ( n 1 / ( n 1 + n 2 + ) ) n − n 0 + n 1 − ϕ t − ϕ t ( n 1 / ( n 1 + n 2 + ) ) As n 1 should equal n 0 they cancel each other out and this formula is reduced to: S ˆ ( 1 ) t . C o r r = n 1 − ϕ t ( S / ( 1 − S ) ) n − ϕ t ( 1 + ( S / ( 1 − S ) ) ) = n 1 − ϕ t ( S / ( 1 − S ) ) n − ϕ t ( 1 ( 1 − S ) ) = S so S ˆ ( 1 ) . C o r r is unbiased at any time, as expected. And: S ˆ ( 0 + 1 ) t = n ˆ ′ 0 + n ˆ ′ 1 2 n = n 0 + ϕ t + n 1 − ϕ t ( n 1 / ( n 1 + n 2 + ) ) 2 n because S = n 0 + n 1 /2 n , it follows that: S ˆ ( 0 + 1 ) t = S + ϕ t ( 1 − ( n 1 / ( n 1 + n 2 + ) ) ) 2 n = S + ϕ t ( 1 − ( S / ( 1 − S ) ) ) 2 n = S + ϕ t ( ( 1 − 2 S ) / ( 1 − S ) ) 2 n with relative bias: (A.3) R b i a s ( S ˆ ( 0 + 1 ) t ) = S ˆ ( 0 + 1 ) t S − 1 = ϕ t 2 S · n 1 − 2 S 1 − S = R b i a s ( S ˆ ( 0 ) t ) 1 − 2 S 2 · ( 1 − S ) (b) When the oversampling of day_0 has a negative effect only on n 1 (repulsion effect) the biases shown in Table 5 are derived as above just by taking into account that then oversampling of day_0 individuals and the undersampling of day_1 will be of equal magnitude, but opposite signs (( ± ϕ t )), whilst n ˆ ′ 2 + = n 2 + . References Alday et al., 2008 A. Alday A. Uriarte M. Santos I. Martin A.M. Martinez de Murguia L. Motos Degeneration of postovulatory follicles of the Bay of Biscay anchovy ( Engraulis encrasicolus L.) Sci. Mar. 72 2008 565 575 Alday et al., 2010 A. Alday M. Santos A. Uriarte I. Martin U. Martinez L. Motos Revision of criteria for the classification of Postovulatory Follicles degeneration, for the Bay of Biscay anchovy ( Engraulis encrasicolus L.) Rev. Invest. Mar. 17 2010 165 171 http://www.azti.es/rim/component/content/article/32.html Alheit et al., 1984 J. Alheit V.H. Alarcon B.J. Macewicz Spawning frequency and sex ratio in the Peruvian anchovy Engraulis ringens Calif. Coop. Oceanic Fish. Invest. Rep. 25 1984 43 52 Armstrong et al., 1988 M. Armstrong P. Shelton I. Hampton G. Jolly Y.C. Melo Egg production estimates of anchovy biomass in the Southern Benguela System Calif. Coop. Oceanic Fish. Invest. Rep. 29 1988 137 156 Claramunt et al., 2007 G. Claramunt R. Serra L.R. Castro L.A. Cubillos Is the spawning frequency dependent on female size? Empirical evidence in Sardinops sagax and Engraulis ringens off northern Chile Fish. Res. 85 2007 248 257 Clarke, 1987 T.A. Clarke Fecundity and spawning frequency of the Hawaiian anchovy or Nehu Encrasicholina purpurea Fish. Bull. 85 1987 127 138 Cubillos et al., 2007 L.A. Cubillos P. Ruiz G. Claramunt S. Gacitúa S. Núñez L.R. Castro K. Riquelme C. Alarcón C. Oyarzun A. Sepúlveda Spawning, daily egg production, and spawning stock biomass estimation for common sardine (Strangomera bentincki) and anchovy (Engraulis ringens) off central southern Chile in 2002 Fish. Res. 86 2007 228 240 Dickerson et al., 1992 T.L. Dickerson B.J. Macewicz J.R. Hunter Spawning frequency and batch fecundity of chub mackerel Scomber japonicus , during 1985 Calif. Coop. Oceanic Fish. Invest. Rep. 33 1992 130 140 Dimmlich et al., 2009 W.F. Dimmlich T.M. Ward W.G. Breed Spawning dynamics and biomass estimates of an anchovy Engraulis australis population in contrasting gulf and shelf environments J. Fish Biol. 75 2009 1560 1576 Fitzhugh and Hettler, 1995 R.G. Fitzhugh F.W. Hettler Temperature influence on postovulatory follicle degeneration in Atlantic menhaden, Brevoortia tyrannus Fish. Bull. 93 1995 568 572 Funamoto and Aoki, 2002 T. Funamoto I. Aoki Reproductive ecology of Japanese anchovy off the Pacific coast of eastern Honshu, Japan J. Fish Biol. 60 2002 154 169 Funamoto et al., 2004 T. Funamoto I. Aoki Y. Wada Reproductive characteristics of Japanese anchovy, Engraulis japonicus , in two bays of Japan Fish. Res. 70 2004 71 81 Ganias et al., 2007 K. Ganias C. Nunes Y. Stratoudakis Degeneration of postovulatory follicles in the Iberian sardine Sardina pilchardus : structural changes and factors affecting resorption Fish. Bull. 105 2007 131 139 Ganias et al., 2003 K. Ganias S. Somarakis A. Machias A. Theodorou Evaluation of spawning frequency in a Mediterranean sardine population ( Sardina pilchardus sardina ) Mar. Biol. 142 2003 1169 1179 Ganias, 2008 K. Ganias Ephemeral spawning aggregations in the Mediterranean sardine, Sardina pilchardus : a comparison with other multiple-spawning clupeoids Mar. Biol. 155 2008 293 301 Ganias, 2009 K. Ganias Linking sardine spawning dynamics to environmental variability Estuar. Coast. Shelf Sci. 84 2009 402 408 Ganias, In press Ganias, K. Thirty years of using the postovulatory follicles method: overview, problems and alternatives. Fish. Res., this volume, in press. García et al., 1992 A. García N. Pérez N.C.H. Lo A. Lago de Lanzós A. Solá The Egg Production Method applied to the spawning biomass estimation of sardine, Sardina pilchardus (Walb) on the North Atlantic Spanish coast Bol. Inst. Esp. Oceanogr. 8 1992 123 138 Goldberg et al., 1984 S.R. Goldberg V. Alarcon J. Alheit Postovulatory follicle histology of the Pacific sardine, Sardinops sagax , from Peru Fish. Bull. 82 1984 443 445 Hampton, 1996 I. Hampton Acoustic and egg-production estimates of South African anchovy biomass over a decade: comparisons, accuracy and utility ICES J. Mar. Sci. 53 1996 493 500 Hunter and Goldberg, 1980 J.R. Hunter S.R. Goldberg Spawning incidence and batch fecundity in northern anchovy, Engraulis mordax Fish. Bull. 77 1980 641 652 Hunter and Macewicz, 1985 J.R. Hunter B.J. Macewicz Measurement of spawning frequency in multiple spawning fishes R. Lasker An Egg Production Method for Estimating Spawning Biomass of Pelagic Fish: Application to the Northern Anchovy Engraulis mordax. NOAA Tech. Rep. NMFS 36 1985 79 93 Hunter and Lo, 1997 J.R. Hunter N.C.H. Lo The daily egg production method of biomass estimation: some problems and potential improvements Ozeanografika 2 1997 41 69 ICES, 2004 ICES, 2004. In: Stratoudakis, Y., Bernal, M., Uriarte, A. (Eds.), The DEPM Estimation of Spawning Stock Biomass for Sardine and Anchovy. ICES Coop. Res. Rep. No. 268, 91 pp. ICES, 2006 ICES, 2006. Report of the Working Group on Acoustic and Egg Surveys for Sardine and Anchovy in ICES areas VIII and IX, 24–28 October 2005, Vigo, Spain. ICES CM. 2006/LRC:01, 126 pp. ICES, 2007 ICES, 2007. Report of the Working Group on Acoustic and Egg Surveys for Sardine and Anchovy in ICES Areas VIII and IX (WGACEGG), 26–30 November 2007, Palma de Mallorca, Spain. ICES CM 2007/LRC:16, 167 pp. ICES, 2009 ICES, 2009. Report of the Working Group on Anchovy and Sardine (WGANSA), 15-20 June 2009, ICES Headquarters, Copenhagen. ICES CM 2009/ACOM:13, 354 pp. Krebs, 1999 Krebs, C.J., 1999. Ecological Methodology, Second ed. Addison-Wesley Educational Publishers, Inc. London (with corrections to the edition in: http://www.zoology.ubc.ca/∼krebs/books.html ). Lasker, 1985 Lasker, R., (Ed.), 1985. An Egg Production Method for Estimating Spawning Biomass of Pelagic Fish: Application to the Northern Anchovy, Engraulis mordax . NOAA Tech. Rep. NMFS 36. Lo et al., 2005 N. Lo B.J. Macewicz D.A. Griffith Spawning biomass of pacific sardine ( Sardinops sagax ), from 1994-2004 off California Calif. Coop. Oceanic Fish. Invest. Rep. 46 2005 96 112 Lowerre-Barbieri et al., 1996 S.K. Lowerre-Barbieri M.E. Chittenden Jr. L.R. Barbieri Variable spawning activity and annual fecundity of weakfish in Chesapeake Bay Trans. Am. Fish. Soc. 125 1996 532 545 Luo and Musick, 1991 J. Luo J. Musick Reproductive biology of the Bay Anchovy, Anchoa mitchilli , in Chespeake Bay Trans. Am. Fish. Soc. 120 1991 801–710 Maack and George, 1999 G. Maack M.R. George Contributions to the reproductive biology of Encrasicholina punctifer Fowler, 1938 (Engraulidae) from West Sumatra, Indonesia Fish. Res. 44 1999 113 120 Macewicz and Hunter, 1993 B. Macewicz J.R. Hunter Spawning frequency and batch fecundity of jack mackerel, Trachurus symmetricus , off California during 1991 Calif. Coop. Oceanic Fish. Invest. Rep. 34 1993 112 121 Macewicz et al., 1996 B.J. Macewicz J.J. Castro-Gonzalez C.E. Cotero-Altamirano J.R. Hunter Adult reproductive parameters of Pacific sardine (Sardinops sagax) during 1994 Calif. Coop. Oceanic Fish. Invest. Rep. 37 1996 140 151 McBride et al., 2002 R.S. McBride F.J. Stengard B. Mahmoudi Maturation and diel reproductive periodicity of round scad (Carangidae: Decapterus punctatus ) Mar. Biol. 140 2002 713 722 McCullagh and Nelder, 1989 P. McCullagh J.A. Nelder Generalized Linear Models, Monographs on Statistic and Applied Probability Second ed. 1989 Chapman & Hall London Motos, 1996 L. Motos Reproductive biology and fecundity of the Bay of Biscay anchovy population ( Engraulis encrasicolus L.) Sci. Mar. 60 Suppl. 2 1996 195 207 Motos et al., 2005 Motos, L., Uriarte, A., Prouzet, P., Santos, M., Alvarez, P., Sagarminaga, Y., 2005. Assessing the Bay of Biscay anchovy population by DEPM: a review 1989-2001. In: Castro, L.R., Freón, P., van der Lingen, C.D., Uriarte, A. (Eds.), Report of the SPACC Meeting on Small Pelagic Fish Spawning Habitat Dynamics and the Daily Egg Production Method (DEPM). GLOBEC Report 22, xiv, pp. 88–90. Pájaro et al., 2009 M. Pájaro G.J. Macchi E. Leonarduzzi J.E. Hansen Spawning biomass of Argentine anchovy ( Engraulis anchoita ) from 1996 to 2004 using the Daily Egg Production Method J. Mar. Biol. Assoc. U.K. 89 2009 829 837 Parker, 1980 K. Parker A direct method for estimating northern anchovy, Engraulis mordax , spawning biomass Fish. Bull. 78 1980 541 544 Pérez et al., 1992 N. Pérez I. Figueiredo B.J. Macewicz The spawning frequency of sardine, Sardina pilchardus (Walb.), off the Atlantic Iberian coast Bol. Inst. Esp. Oceanogr. 8 1992 175 189 Picquelle and Stauffer, 1985 S. Picquelle G. Stauffer Parameter estimation for an egg production method of anchovy biomass assessment R. Lasker An Egg Production Method for Estimating Spawning Biomass of Pelagic Fish: Application to the Northern Anchovy Engraulis mordax. NOAA Tech. Rep. NMFS 36 1985 7 16 Priede and Watson, 1993 I.G. Priede J.J. Watson An evaluation of the daily egg-production method for estimating biomass of Atlantic mackerel ( Scomber scombrus ) Bull. Mar. Sci. 53 1993 891 911 Rogers et al., 2003 P.J. Rogers T. Geddes T.M. Ward Blue sprat Spratelloides robustus (Clupeidae: Dussumieriinae): a temperate clupeoid with a tropical life history strategy? Mar. Biol. 142 2003 809 824 Roumillat and Brouwer, 2004 W.A. Roumillat M.C. Brouwer Reproductive dynamics of female spotted sea trout ( Cynoscion nebulosus ) in South Carolina Fish. Bull. 102 2004 473 487 Santiago and Sanz, 1992 J. Santiago A. Sanz Egg production estimates of the Bay of Biscay anchovy, Engraulis encrasicolus (L.), spawning stock in 1987 and 1988 Bol. Inst. Esp. Oceanogr. 8 1992 225 230 Sanz et al., 1992 A. Sanz L. Motos A. Uriarte Daily fecundity of the Bay of Biscay anchovy, Engraulis encrasicolus (L.), population in 1987 Bol. Inst. Esp. Oceanogr. 8 1992 203 214 Schaefer, 1996 K. Schaefer Spawning time, frequency and batch fecundity of yellowfin tuna, Thunnus albacares , near Clipperton Atoll in the eastern Pacific Ocean Fish. Bull. 94 1996 98 112 Shelton et al., 1993 P.A. Shelton M.J. Armstrong B.A. Roel An overview of the application of the daily egg production method in the assessment and management of anchovy in the Southeast Atlantic Bull. Mar. Sci. 53 1993 778 794 Somarakis et al., 2000 S. Somarakis A. Machias C. Koutsikopoulos E. Maraveya M. Giannoulaki N. Tsimenides Distribution of anchovy and its spawning grounds in the central Aegean and Ionian seas Proceedings of the Sixth Hellenic Symposium on Oceanography and Fisheries Vol. 2 (in Greek, with English abstract) 2000 94 97 Somarakis et al., 2004 S. Somarakis I. Palomera A. García L. Quintanilla C. Koutsikopoulos A. Uriarte L. Motos Daily egg production of anchovy in European waters ICES J. Mar. Sci. 61 2004 944 958 Somarakis, 2005 S. Somarakis Marked interannual differences in reproductive parameters and daily egg production of anchovy in the northern Aegean Sea Belg. J. Zool. 135 2005 247 252 Stratoudakis et al., 2006 Y. Stratoudakis M. Bernal K. Ganias A. Uriarte The daily egg production method (DEPM): recent advances, current applications and future challenges Fish Fish. 7 2006 35 57 Takasuka et al., 2005 A. Takasuka Y. Oozeki H. Kubota Y. Tsuruta T. Funamoto Temperature impacts on reproductive parameters for Japanese anchovy: comparison between inshore and offshore waters Fish. Res. 76 2005 475 482 Taylor et al., 1998 R.G. Taylor H.J. Grier J.A. Whittington Spawning rhythms of common snook in Florida J. Fish Biol. 53 1998 502 520 Wang and Houde, 1994 S.B. Wang E.D. Houde Energy storage and dynamics in bay anchovy Anchoa mitchilli Mar. Biol. 121 1994 219 227 Ward et al., 2001 M.T. Ward F. Hoedt M. McLeay F.D. Dimmlich M. Kinloch G. Jackson R. McGarvey P.J. Rogers K. Jones Effect of the 1995 and 1998 mass mortality events on the spawning biomass of sardine, Sardinops sagax , in South Australian Waters ICES J. Mar. Sci. 58 2001 865 875 Wright, 1992 P.J. Wright Ovarian development, spawning frequency and batch fecundity in Encrasicholina heteroloba (Ruppell, 1858) J. Fish Biol. 40 1992 833 844 Yamada et al., 1998 T. Yamada I. Aoki I. Mitani Spawning time, spawning frequency and fecundity of Japanese chub mackerel, Scomber japonicus , in the waters around the Izu Islands, Japan Fish. Res. 38 1998 83 89 Yoneda et al., 2002 M. Yoneda K. Futagawa M. Tokimura H. Horikawa S. Matsuura M. Matsuyama Reproductive cycle, spawning frequency and batch fecundity of the female whitefin jack, Kaiwarinus equula , in the East China Sea Fish. Res. 57 2002 297 309 Zeldis and Francis, 1998 J.R. Zeldis R.I.C.C. Francis A daily egg production method estimate of snapper biomass in Hauraki Gulf, New Zealand ICES J. Mar. Sci. 55 1998 522 534
MoreTranslated text
Key words
Spawning fraction,Post-ovulatory follicles,Final oocyte maturation,Reproduction,Anchovy
AI Read Science
Must-Reading Tree
Example
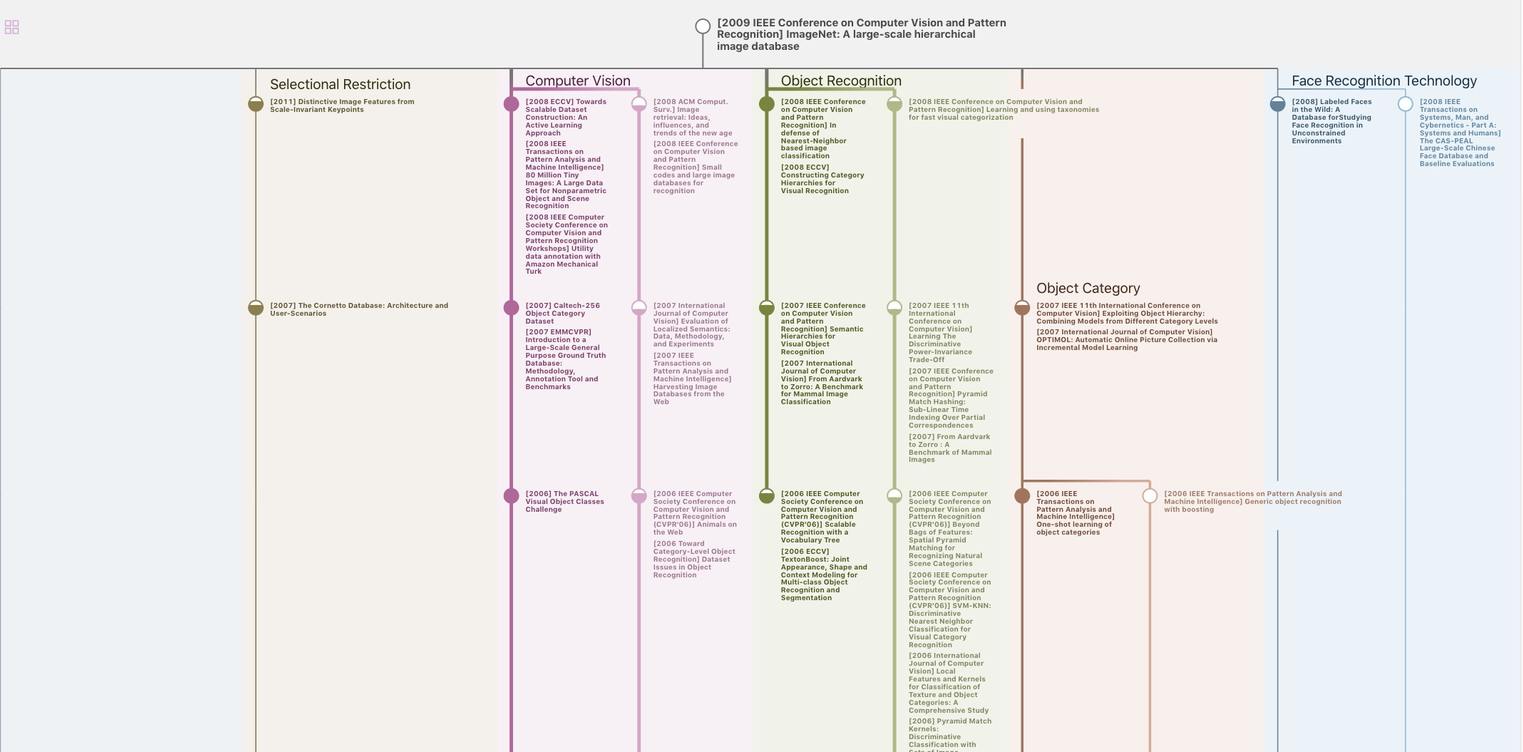
Generate MRT to find the research sequence of this paper
Chat Paper
Summary is being generated by the instructions you defined