Named Entity Recognition in Tweets: An Experimental Study.
EMNLP '11: Proceedings of the Conference on Empirical Methods in Natural Language Processing(2011)
摘要
People tweet more than 100 Million times daily, yielding a noisy, informal, but sometimes informative corpus of 140-character messages that mirrors the zeitgeist in an unprecedented manner. The performance of standard NLP tools is severely degraded on tweets. This paper addresses this issue by re-building the NLP pipeline beginning with part-of-speech tagging, through chunking, to named-entity recognition. Our novel T-ner system doubles F 1 score compared with the Stanford NER system. T-ner leverages the redundancy inherent in tweets to achieve this performance, using LabeledLDA to exploit Freebase dictionaries as a source of distant supervision. LabeledLDA outperforms co-training, increasing F 1 by 25% over ten common entity types. Our NLP tools are available at: http://github.com/aritter/twitter_nlp
更多查看译文
关键词
NLP pipeline beginning,NLP tool,standard NLP tool,F1 score,Stanford NER system,novel T-ner system,140-character message,Freebase dictionary,common entity type,distant supervision,entity recognition,experimental study
AI 理解论文
溯源树
样例
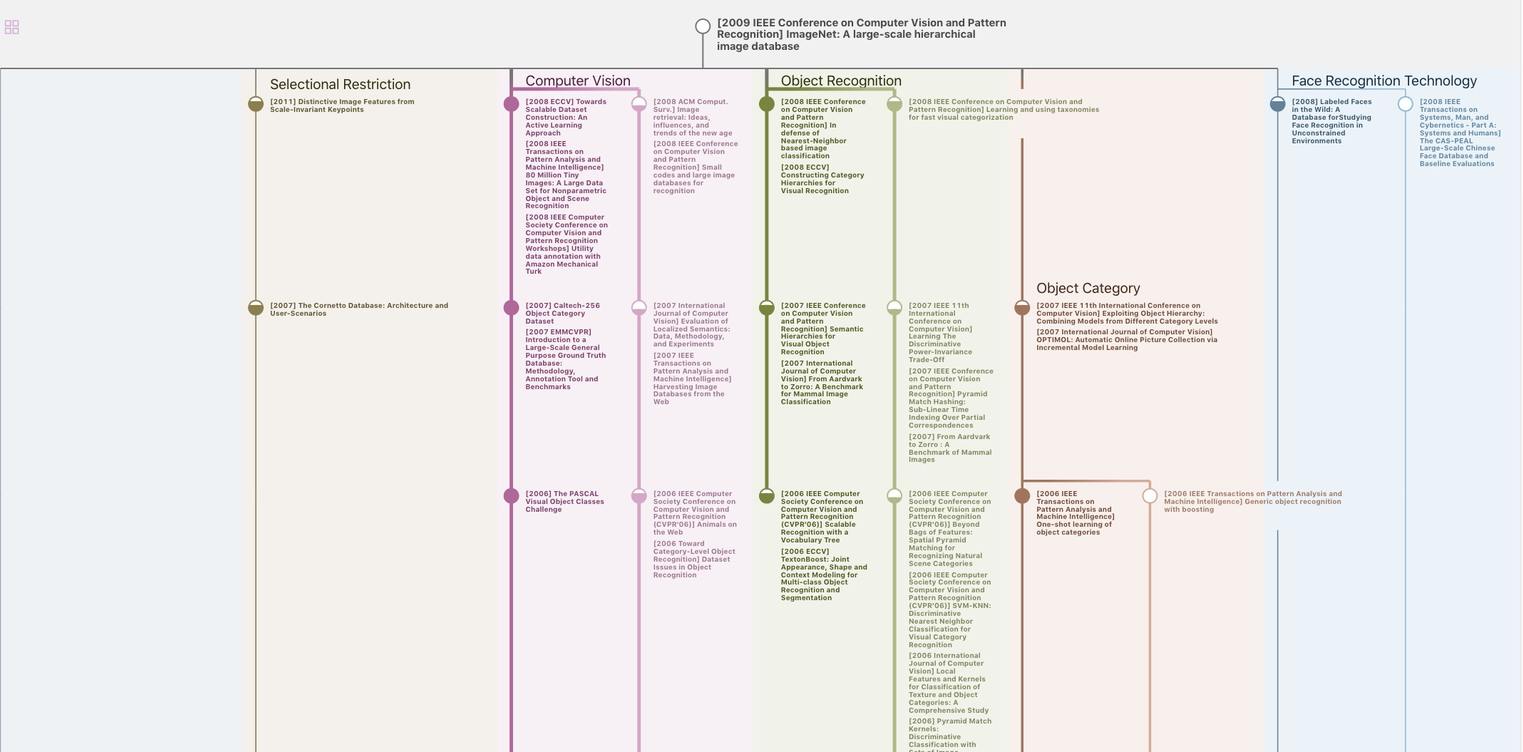
生成溯源树,研究论文发展脉络
Chat Paper
正在生成论文摘要