Fast And Effective Retraining On Contrastive Vocal Characteristics With Bidirectional Long Short-Term Memory Nets
INTERSPEECH 2006 AND 9TH INTERNATIONAL CONFERENCE ON SPOKEN LANGUAGE PROCESSING, VOLS 1-5(2006)
摘要
We apply Long Short-Term Memory (LSTM) recurrent neural networks to a large corpus of unprompted speech - the German part of the VERBMOBIL corpus. By training first on a fraction of the data, then retraining on another fraction, we both reduce time costs and significantly improve recognition rates. Contrastive retraining on the initial vowel cluster fraction of the data according to the Psycho-Computational Model of Sound Acquisition (PCMSA) shows higher frame by frame correctness due to more sparseness and the articulatory position of the sounds. For comparison we show recognition rates of Hidden Markov Models (HMMs) on the same corpus, and provide a promising extrapolation for HMM-LSTM hybrids.
更多查看译文
关键词
Bidirectional Long Short-Term Memory,recurrent neural networks,retraining on data fractions,Psycho-Computational Model of Sound Acquisition
AI 理解论文
溯源树
样例
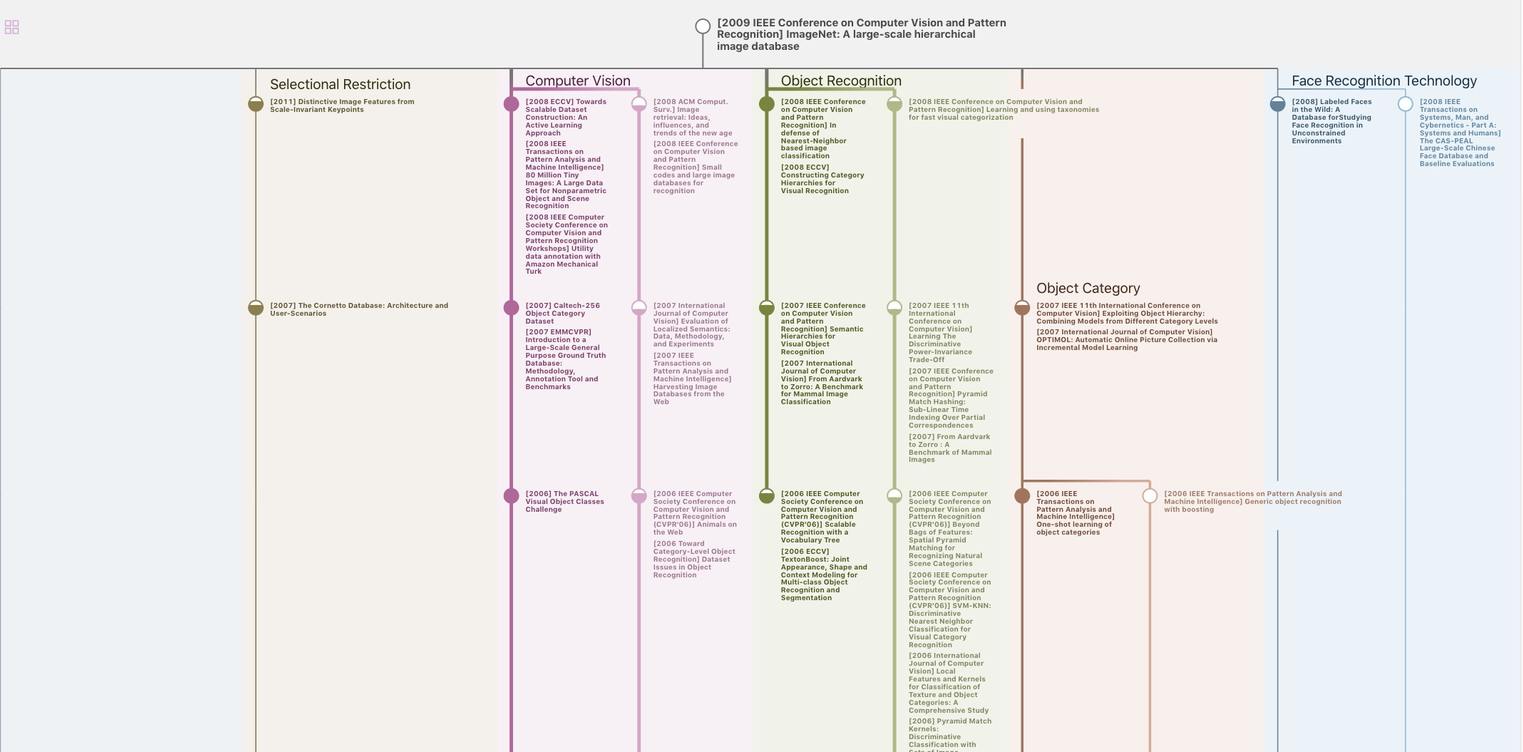
生成溯源树,研究论文发展脉络
Chat Paper
正在生成论文摘要