Towards total scene understanding: Classification, annotation and segmentation in an automatic framework
CVPR(2009)
摘要
Given an image, we propose a hierarchical generative model that classifies the overall scene, recognizes and segments each object component, as well as annotates the image with a list of tags. To our knowledge, this is the first model that performs all three tasks in one coherent framework. For instance, a scene of a `polo game' consists of several visual objects such as `human', `horse', `grass', etc. In addition, it can be further annotated with a list of more abstract (e.g. `dusk') or visually less salient (e.g. `saddle') tags. Our generative model jointly explains images through a visual model and a textual model. Visually relevant objects are represented by regions and patches, while visually irrelevant textual annotations are influenced directly by the overall scene class. We propose a fully automatic learning framework that is able to learn robust scene models from noisy Web data such as images and user tags from Flickr.com. We demonstrate the effectiveness of our framework by automatically classifying, annotating and segmenting images from eight classes depicting sport scenes. In all three tasks, our model significantly outperforms state-of-the-art algorithms.
更多查看译文
关键词
visual model,automatic framework,image segmentation,image annotation,sport scene,visual object,object component recognition,total scene understanding,image classification,polo game,object recognition,textual model,noise measurement,data mining,layout,switches,visualization
AI 理解论文
溯源树
样例
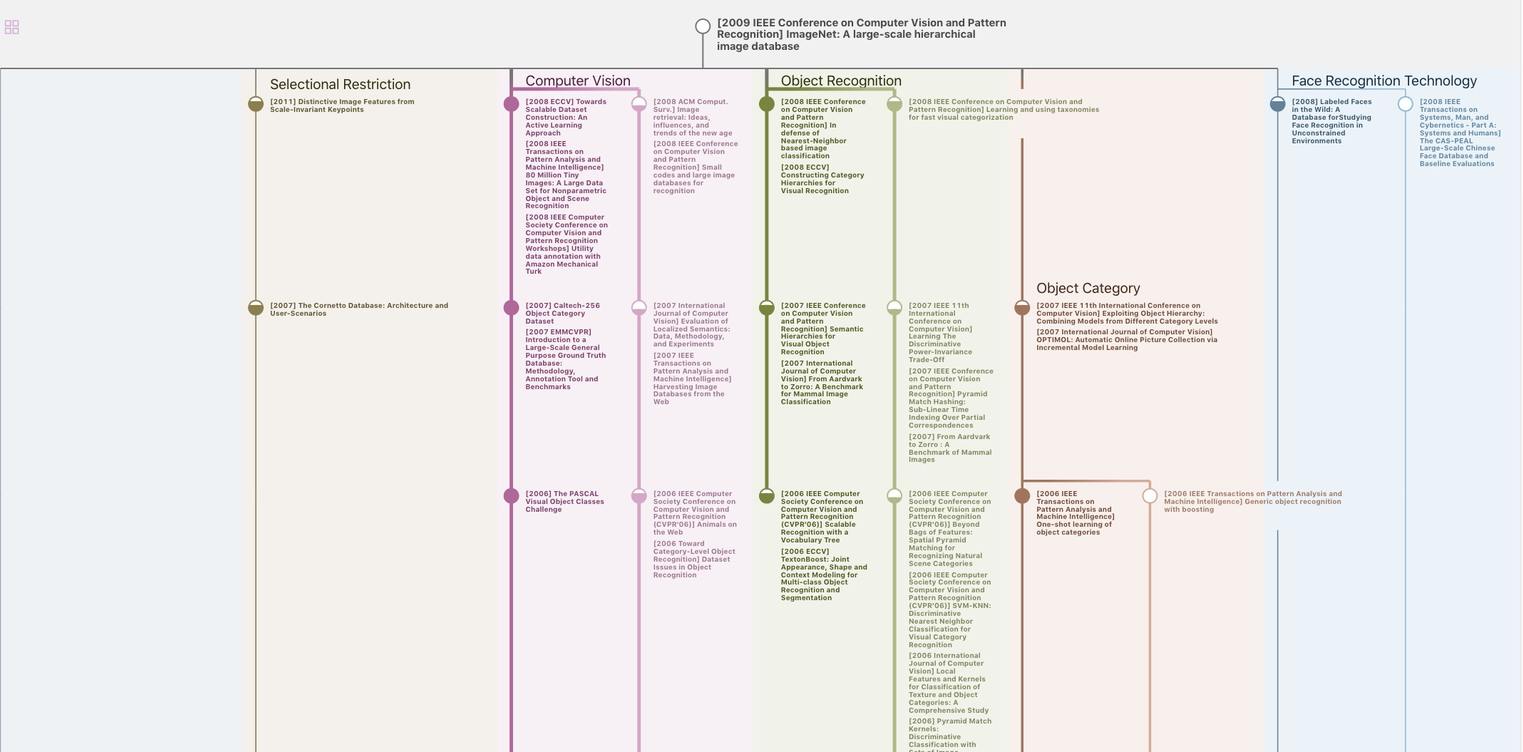
生成溯源树,研究论文发展脉络
Chat Paper
正在生成论文摘要