A Triplet-Branch Convolutional Neural Network for Part-Based Gait Recognition.
Comput. Syst. Sci. Eng.(2023)
摘要
Intelligent vision-based surveillance systems are designed to deal with the gigantic volume of videos captured in a particular environment to perform the interpretation of scenes in form of detection, tracking, monitoring, behavioral analysis, and retrievals. In addition to that, another evolving way of surveillance systems in a particular environment is human gait-based surveillance. In the existing research, several methodological frameworks are designed to use deep learning and traditional methods, nevertheless, the accuracies of these methods drop substantially when they are subjected to covariate conditions. These covariate variables disrupt the gait features and hence the recognition of subjects becomes difficult. To handle these issues, a region-based triplet-branch Convolutional Neural Network (CNN) is proposed in this research that is focused on different parts of the human Gait Energy Image (GEI) including the head, legs, and body separately to classify the subjects, and later on, the final identification of subjects is decided by probability-based majority voting criteria. Moreover, to enhance the feature extraction and draw the discriminative features, we have added soft attention layers on each branch to generate the soft attention maps. The proposed model is validated on the CASIA-B database and findings indicate that part-based learning through triplet-branch CNN shows good performance of 72.98% under covariate conditions as well as also outperforms single-branch CNN models.
更多查看译文
关键词
recognition,neural network,triplet-branch,part-based
AI 理解论文
溯源树
样例
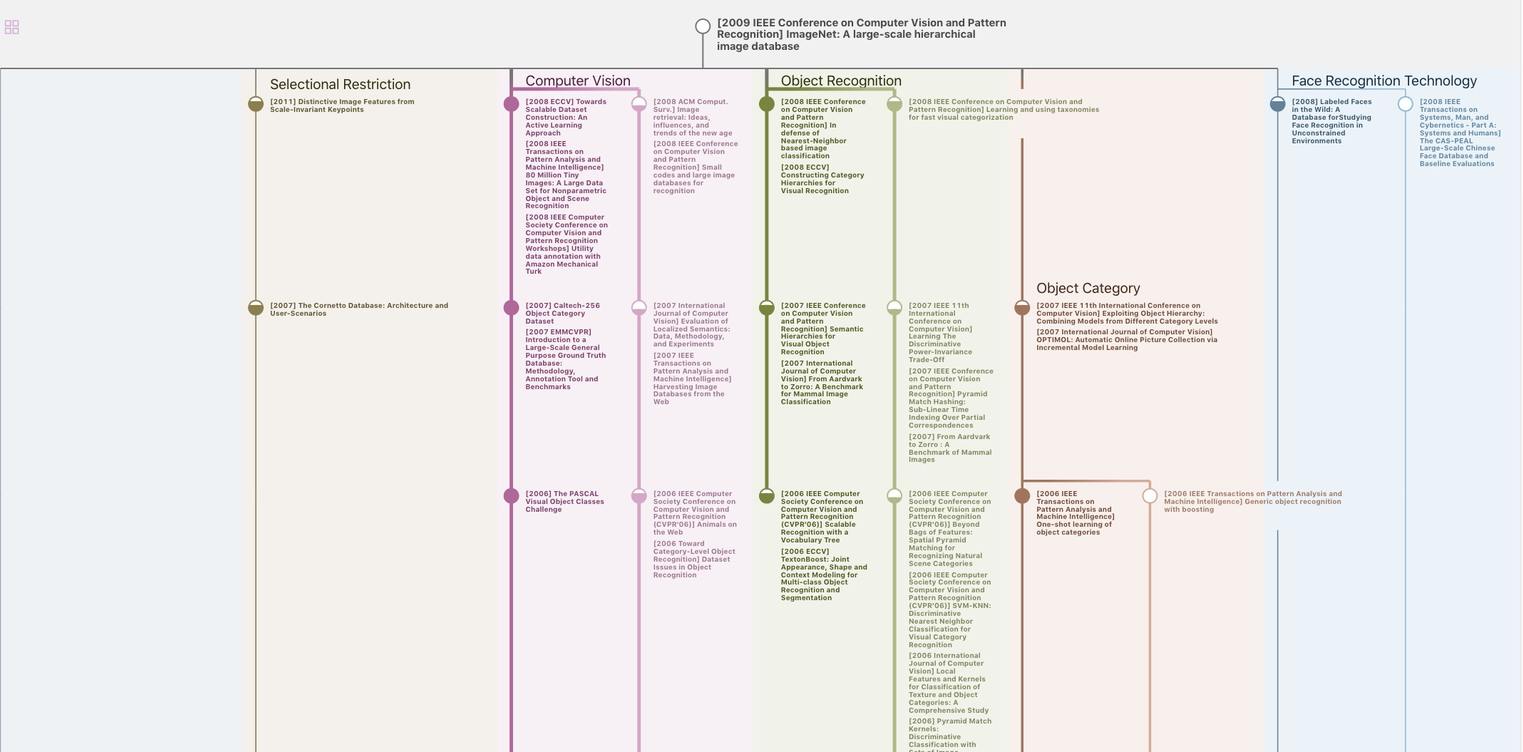
生成溯源树,研究论文发展脉络
Chat Paper
正在生成论文摘要