Predicting water quality in municipal water management systems using a hybrid deep learning model
Engineering Applications of Artificial Intelligence(2024)
摘要
Increasing municipal waste generation puts more and more municipal water resources at high risk. Accurate prediction of water quality becomes critical for effective protection of the water resources. Due to the nonlinear and non-stationary characteristics of water quality data of the municipal water resources, it is challenging to achieve high prediction accuracy, especially for medium-term and long-term predictions. To address this issue, we propose a novel hybrid deep learning model to predict water quality multiple steps ahead. The proposed model adopts the encoder–decoder structure in the form of two long short-term memory (LSTM) networks, integrated with the attention mechanism and a convolutional neural network (CNN). The model extracts the complex correlation between multiple water quality features through the CNN, and uses the two LSTM networks to transfer historical information to predictions, with an attention layer assigning different weights to the different parts of the historical information. Using three years of water quality data collected from an urban river, we experimentally show that the proposed model outperforms the baseline models by 11%–34% in root mean squared error (RMSE) when predicting dissolved oxygen multiple steps ahead, and by 1%–7% when predicting total phosphorus. Similar improvement has also been found in Nash–Sutcliffeefficiency (NSE) and mean absolute error (MAE). The proposed model is a feasible solution for multi-step medium-term water quality prediction.
更多查看译文
关键词
Water quality,Multi-step prediction,Encoder–decoder structure,Long short-term memory,Convolutional neural network,Attention mechanism
AI 理解论文
溯源树
样例
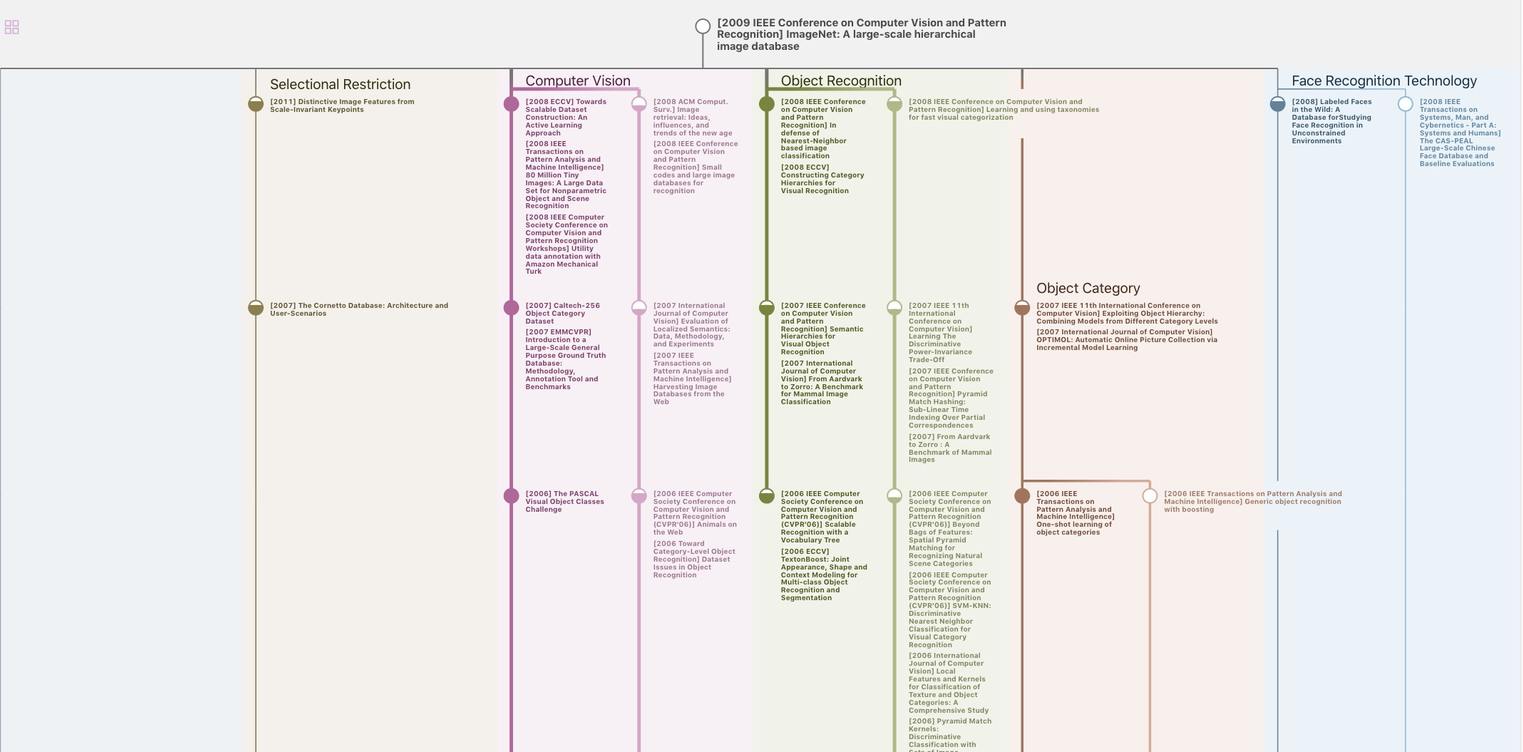
生成溯源树,研究论文发展脉络
Chat Paper
正在生成论文摘要