SU-HH-AUD C-03: Machine Learning Tools for Predicting Clinical Complications in a Multi-Plan IMRT Framework
MEDICAL PHYSICS(2008)
摘要
Purpose: To apply machine learning algorithms to predict clinical outcomes (saliva flow rate, rectal bleeding, EUD, NTCP and PUC) for treatment plans taking into account dose and dose‐volume information. Method and Materials: One hundred twenty‐five (125) plans were generated for a representative head and neck case by varying the input dose‐volume constraints on the OARs (left parotid, right parotid and cord). The method that we used to predict continuous saliva flow rate from the treatment input constraint settings is a sequential minimal optimization algorithm for training a support vector regression model. Two hundred fifty‐six (256) plans were generated for the whole pelvis case by varying the input dose‐volume constraints on the rectum, bladder and bowel. We use the threshold of 66%/50Gy in conjunction with binary classification to predict rectal bleeding. The binary classification method employed is an optimized decision tree. We also calculated the EUD, NTCP, TCP and PUC for both 125 head and neck cases and 256 pelvic cases. Both Lyman‐Kutcher‐Burman (LKB) and Kallam and Agren‐Cronqvist's NTCP models were used. Both continuous and categorical values of these biological measures were predicted by modeling the planning surface using quadratic models and solving with linear programming with three different objective functions: minimizing maximum relative error, absolute error and sum of absolute errors. Results: We repeated the 10‐fold cross‐validation process 10 times and obtained a mean absolute error of 0.00078 with a 95% confidence interval [0.000769, 0.000792] for normalized saliva flow rate. We achieved an average correct prediction percentage of 97.35% and a 95% confidence interval for the correct prediction percentage as [96.89%, 97.81%]. Categorical correct prediction percentages for EUD, NTCP and PUC ranged from 75% to 96%. Conclusion: Our results indicate that machine learning techniques provide invaluable tools for the prediction of treatment complications from input constraints in a multi‐plan environment.
更多查看译文
关键词
machine learning
AI 理解论文
溯源树
样例
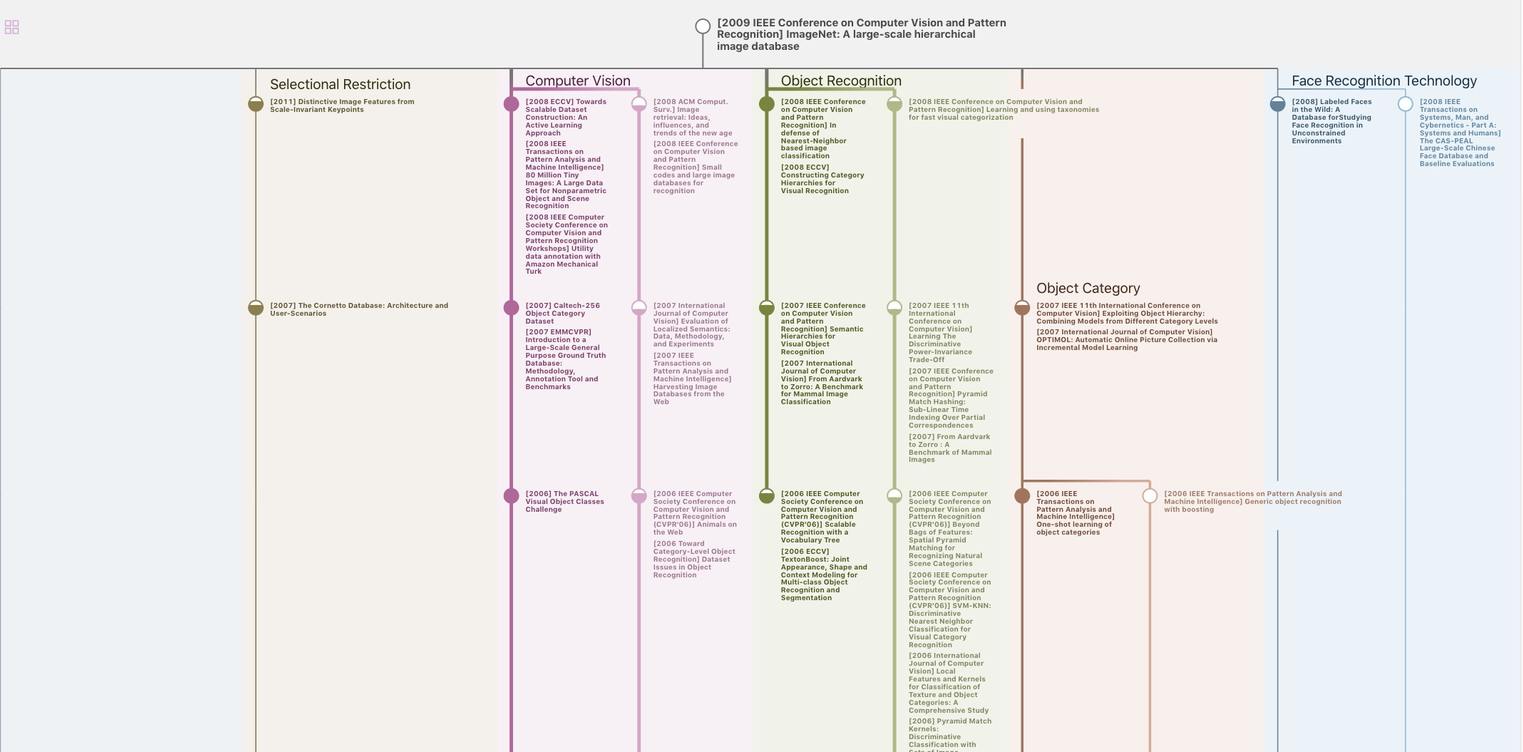
生成溯源树,研究论文发展脉络
Chat Paper
正在生成论文摘要