Continuous Time Dynamic Topic Models
Uncertainty in Artificial Intelligence(2012)
摘要
In this paper, we develop the continuous time dynamic topic model (cDTM). The cDTM is a dynamic topic model that uses Brownian motion to model the latent topics through a sequential collection of documents, where a \topic" is a pattern of word use that we expect to evolve over the course of the col- lection. We derive an ecient variational approximate inference algorithm that takes advantage of the sparsity of observations in text, a property that lets us easily han- dle many time points. In contrast to the cDTM, the original discrete-time dynamic topic model (dDTM) requires that time be discretized. Moreover, the complexity of vari- ational inference for the dDTM grows quickly as time granularity increases, a drawback which limits ne-grained discretization. We demonstrate the cDTM on two news corpora, reporting both predictive perplexity and the novel task of time stamp prediction.
更多查看译文
关键词
discrete time,brownian motion,col
AI 理解论文
溯源树
样例
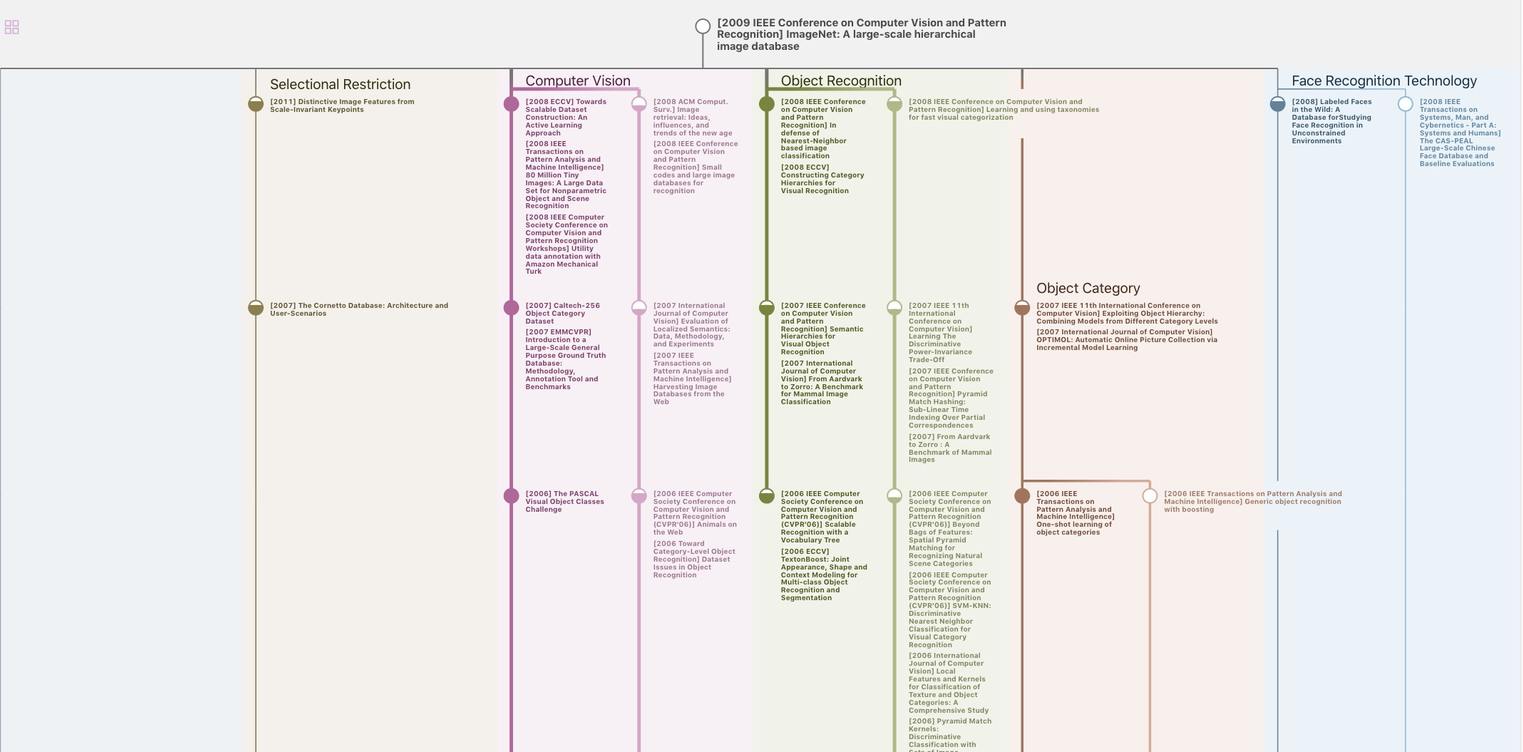
生成溯源树,研究论文发展脉络
Chat Paper
正在生成论文摘要