Clustering, coding, and the concept of similarity
Annals of Mathematics and Artificial Intelligence(2024)
摘要
This paper develops a theory of clustering and coding that combines a geometric model with a probabilistic model in a principled way. The geometric model is a Riemannian manifold with a Riemannian metric, g_ij(x) , which we interpret as a measure of dissimilarity. The probabilistic model consists of a stochastic process with an invariant probability measure that matches the density of the sample input data. The link between the two models is a potential function, U(x) , and its gradient, ∇ U(x) . We use the gradient to define the dissimilarity metric, which guarantees that our measure of dissimilarity will depend on the probability measure. Finally, we use the dissimilarity metric to define a coordinate system on the embedded Riemannian manifold, which gives us a low-dimensional encoding of our original data.
更多查看译文
关键词
Clustering,Prototype coding,Manifold learning,Dimensionality reduction,Dissimilarity metric,58J65,60H10,60J60
AI 理解论文
溯源树
样例
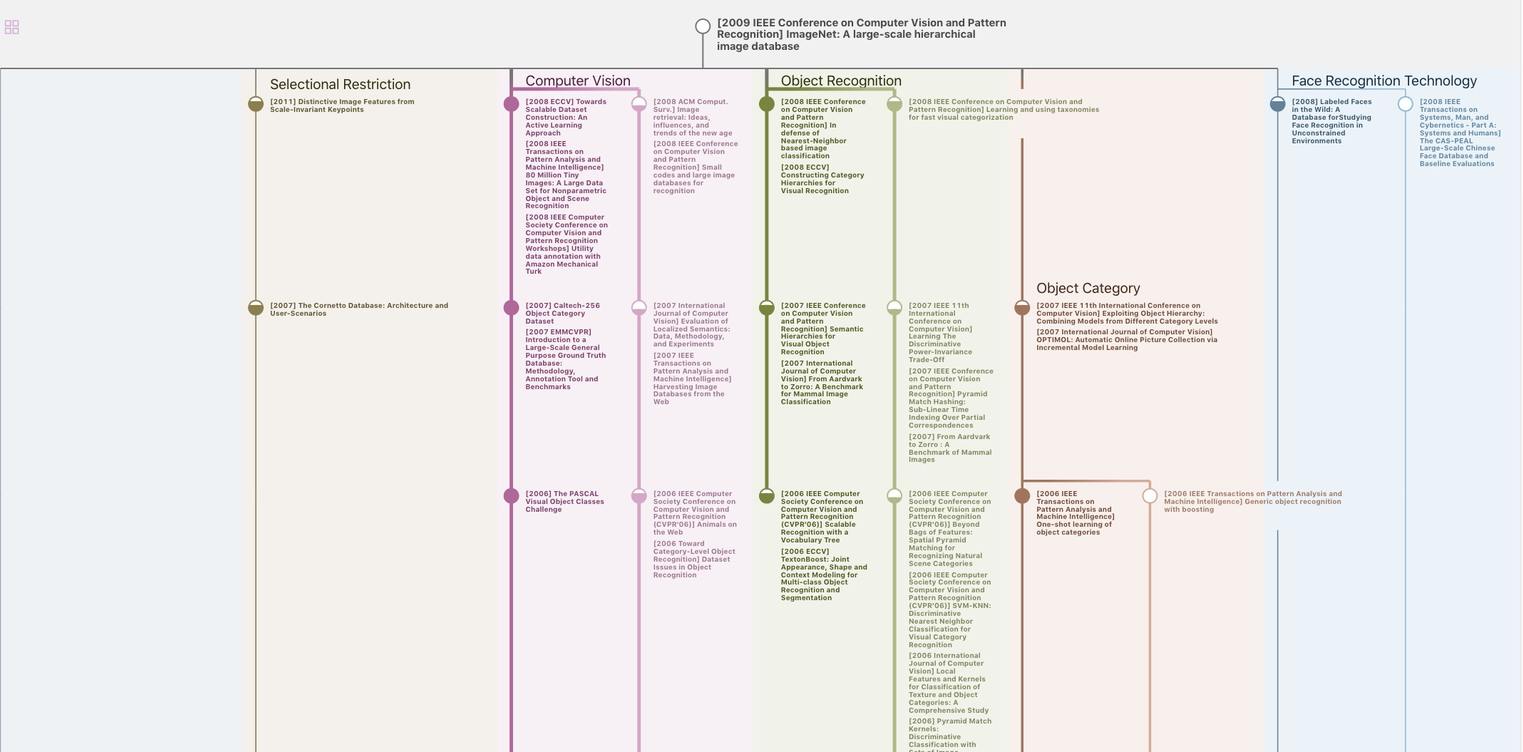
生成溯源树,研究论文发展脉络
Chat Paper
正在生成论文摘要