Occupancy states forecasting with a hidden Markov model for incomplete data, exploiting daily periodicity
ENERGY AND BUILDINGS(2023)
摘要
To automatize HVAC energy savings in buildings, it is useful to forecast the occupants' behaviour. This article deals with such a forecasting problem by exploiting the daily periodicity of the input variables and the ability of the proposed model to learn from missing data. We propose a case study of occupancy behaviour, for which only a history of indirect information is available: CO2 and decibel levels. The unobserved classes are modeled by a Hidden Markov Model (HMM) which emits sensors' data and periodic variables. The fact that Baum-Welch's algorithm for HMMs is an instance of the EM algorithm allows the model to learn from incomplete data. In this case, the missing data are the class labels at every instant (past and future) and the sensors' data in the future, but the time periodic variables are known at every instant: this enables the model to classify both the past instants (history) and the future instants (forecasting). Our method is tested on simulated data sets with 5%, 15%, 25% and 50% missing data that provide respectively an average classification accuracy of 93%, 89%, 85% and 80%. Then, experiments on oneday and one-week forecasting scenarios achieved an average accuracy of 82% and 81%. The proposed method is also applied on a real-life data set which seems to provide promising results.(c) 2023 Elsevier B.V. All rights reserved.
更多查看译文
关键词
hidden markov model,forecasting,incomplete data
AI 理解论文
溯源树
样例
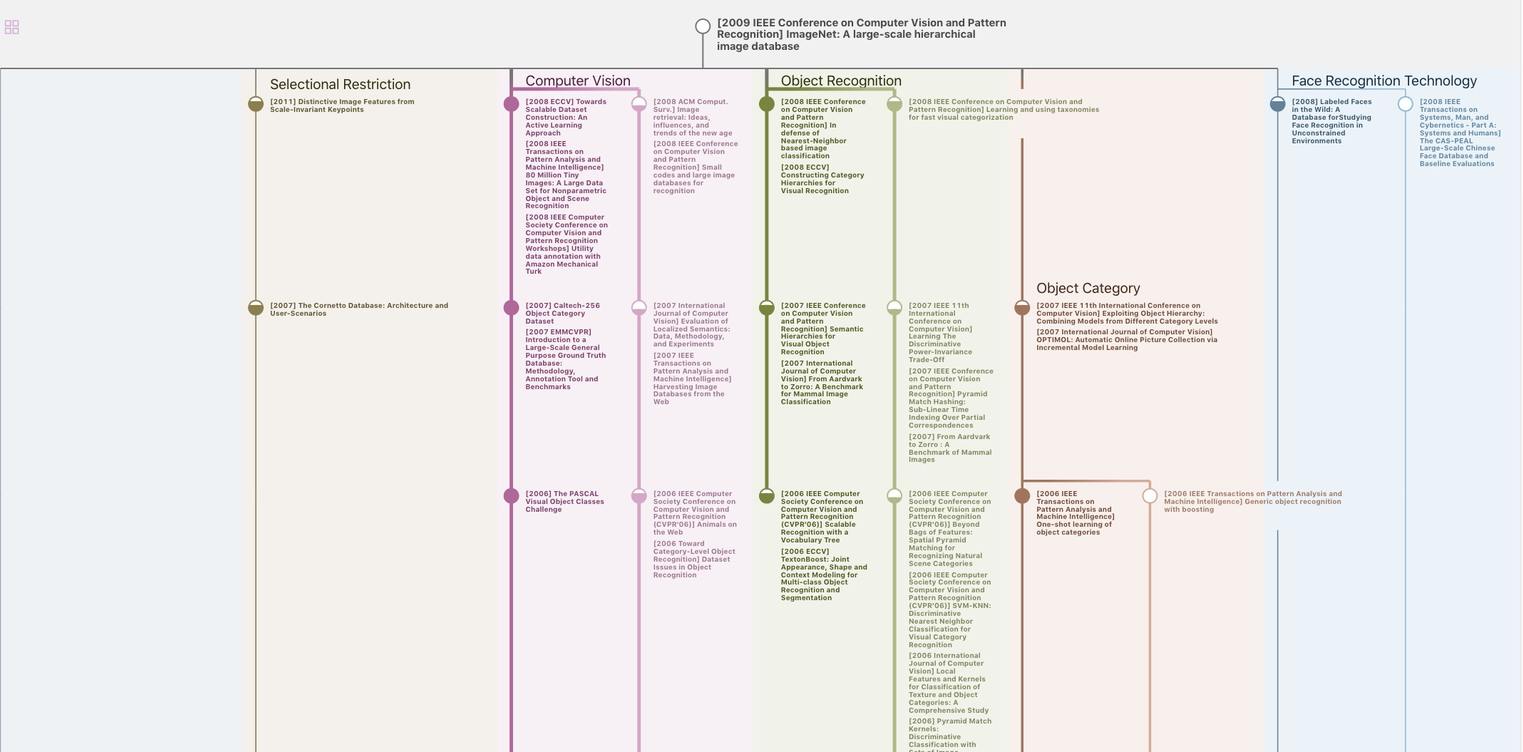
生成溯源树,研究论文发展脉络
Chat Paper
正在生成论文摘要