An EM Algorithm for Joint Feature Selection and
msra
摘要
The problems of accurate classifier design and fea- ture (predictor variable) selection have typically been considered in isolation of one another, with an independent feature selection phase often preceding the training of a classifier. However, the process of feature selection is most effective when it is undertaken with an eye towards retaining those features that are most relevant to the classification task at hand. This paper introduces a novel Bayesian algorithm that simultaneously learns the optimal nonlinear kernel classifier and the subset of predictor variables that contain complete discriminatory information for the classification. This simultaneous learning task is accomplished by an ex- pectation maximization (EM) algorithm that optimizes a single likelihood function which rewards both good classification perfor- mance and sparse feature utilization. This algorithm constitutes a Bayesian extension of the current state-of-the-art Support Vector Machine and Relevance Vector Machine. Experimental evidence for the superior classification accuracy of this learning algorithm is presented on benchmark datasets. In addition, on high dimensional biological datasets, it is shown that the subset of predictor variables that are identified by the feature selection contains several genes whose products are already used to diagnose the same disease in current clinical practice.
更多查看译文
AI 理解论文
溯源树
样例
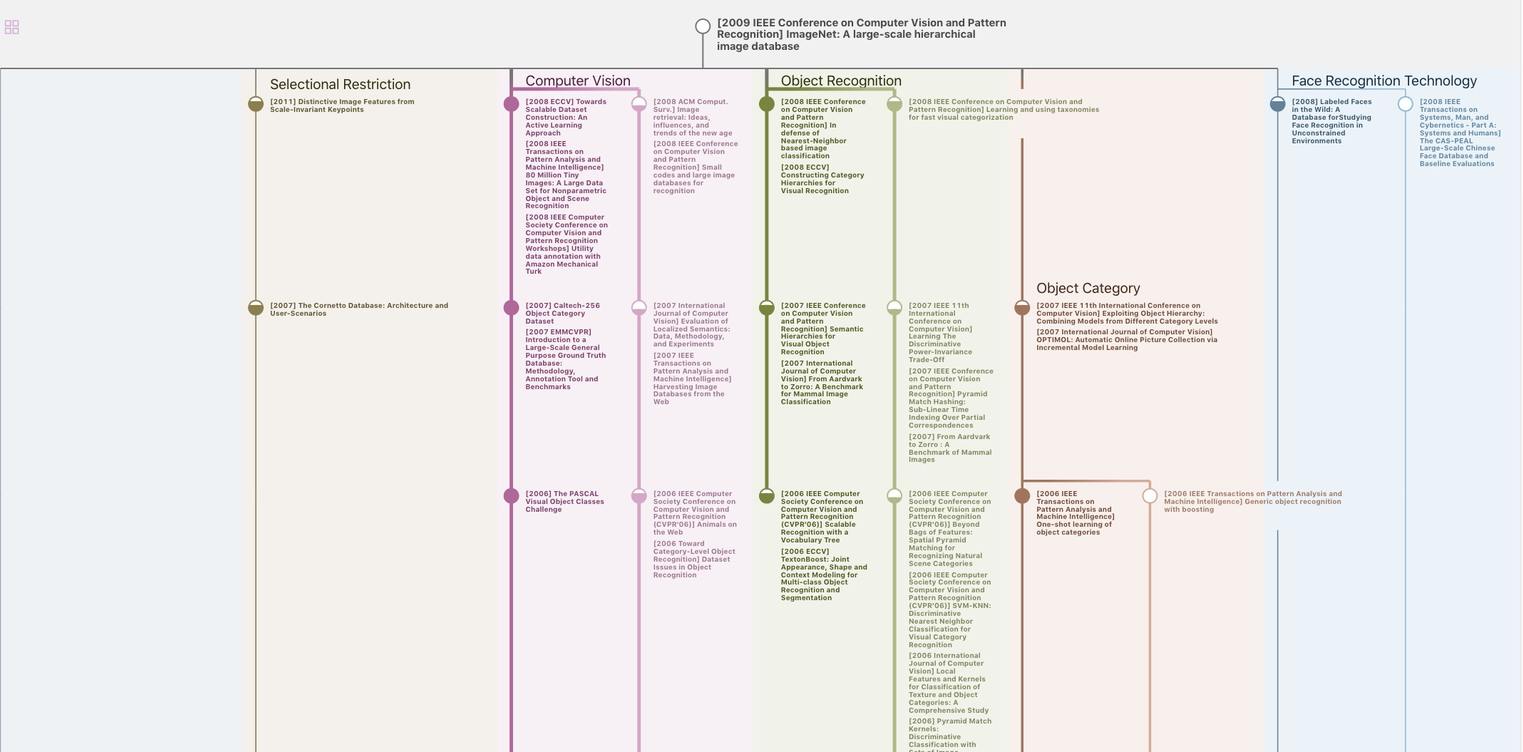
生成溯源树,研究论文发展脉络
Chat Paper
正在生成论文摘要