Pareto Rank Learning in Multi-objective Evolutionary Algorithms
IEEE Congress on Evolutionary Computation(2012)
摘要
In this paper, the interest is on cases where assessing the goodness of a solution for the problem is costly or hazardous to construct or extremely computationally intensive to compute. We label such category of problems as “expensive” in the present study. In the context of multi-objective evolutionary optimizations, the challenge amplifies, since multiple criteria assessments, each defined by an “expensive” objective is necessary and it is desirable to obtain the Pareto-optimal solution set under a limited resource budget. To address this issue, we propose a Pareto Rank Learning scheme that predicts the Pareto front rank of the offspring in MOEAs, in place of the “expensive” objectives when assessing the population of solutions. Experimental study on 19 standard multi-objective benchmark test problems concludes that Pareto rank learning enhanced MOEA led to significant speedup over the state-of-the-art NSGA-II, MOEA/D and SPEA2.
更多查看译文
关键词
evolutionary computation,pareto rank learning,multi-objective evolutionary algorithms,spea2,learning (artificial intelligence),expensive objective,moea,pareto rank learning scheme,multiple criteria assessments,multiobjective benchmark test problems,moea-d,pareto optimisation,nsga-ii,expensive problems,pareto-optimal solution,multiobjective evolutionary optimization algorithms,offspring pareto front rank,optimization,databases,learning artificial intelligence,support vector machines,predictive models,vectors
AI 理解论文
溯源树
样例
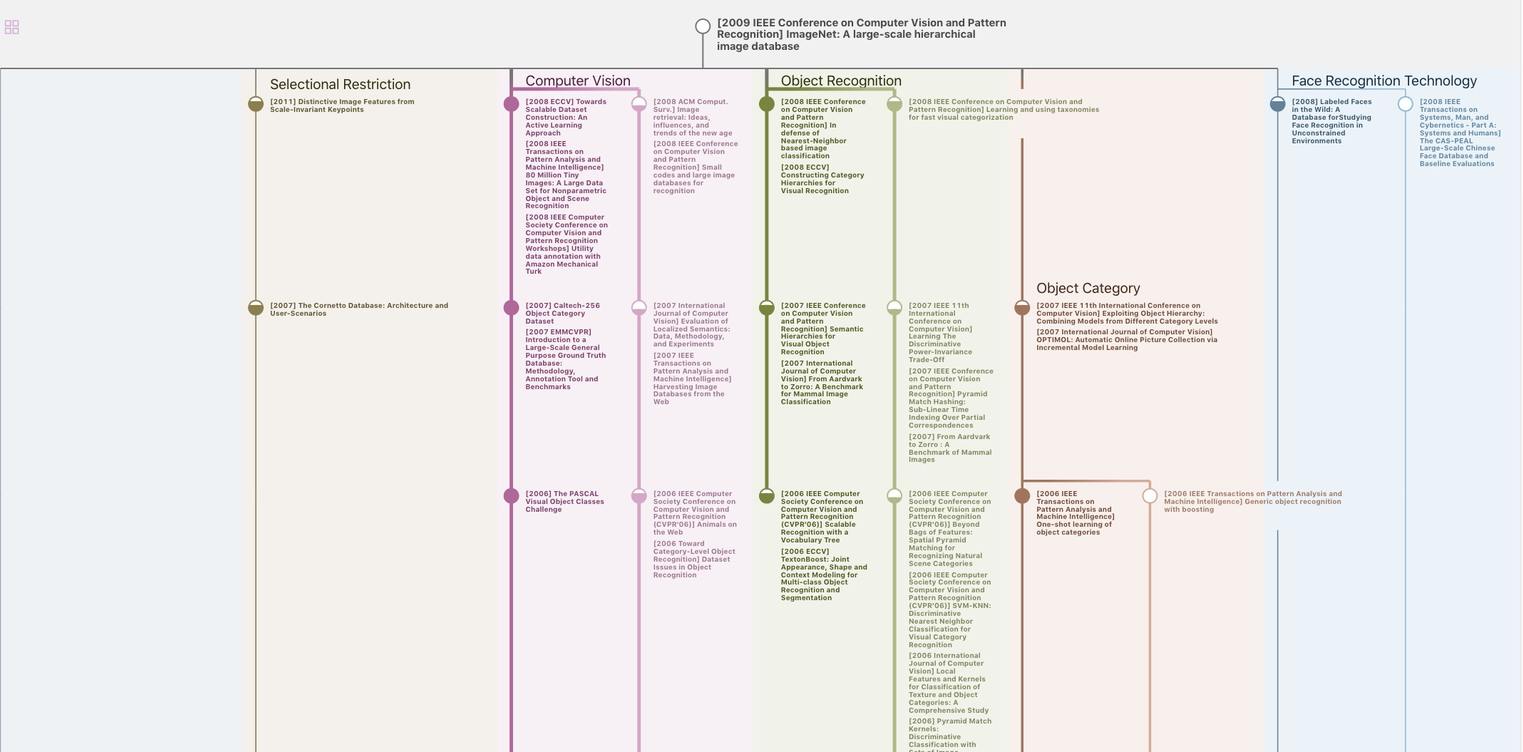
生成溯源树,研究论文发展脉络
Chat Paper
正在生成论文摘要