Multi-Dimensional Feature Scoring For Gene Expression Data
msra(2006)
摘要
Motivation: The analysis of gene expression data presents resear- chers with the problem of finding optimal subsets of genes to focus on. This is a computational and statistical challenge, mostly due to the high-dimensionality of the data and the small amounts of samp- les. Hence, an initial process of gene (feature) selection is usually performed. Results: This paper discusses several methods that perform feature scoring and selection. It focuses on a comparison between common one-dimensional methods (scoring each gene using only its expres- sion values) and our proposed multi-dimensional method (scoring each gene using also its correlation with other genes), based on linear discriminant analysis (LDA). We present several techniques of regularizing the multi-dimensional LDA, aiming to solve the inherent problems of high-dimensional feature space. We compare the performance of these methods using simulati- ons and real data, and specifically address how several parameters (such as sample size and dimensionality) affect the methods. The results show that the multi-dimensional methods outperform the one- dimensional methods, and we discuss the scenarios in which it is more appropriate to use them.
更多查看译文
关键词
sample size,feature selection,feature space
AI 理解论文
溯源树
样例
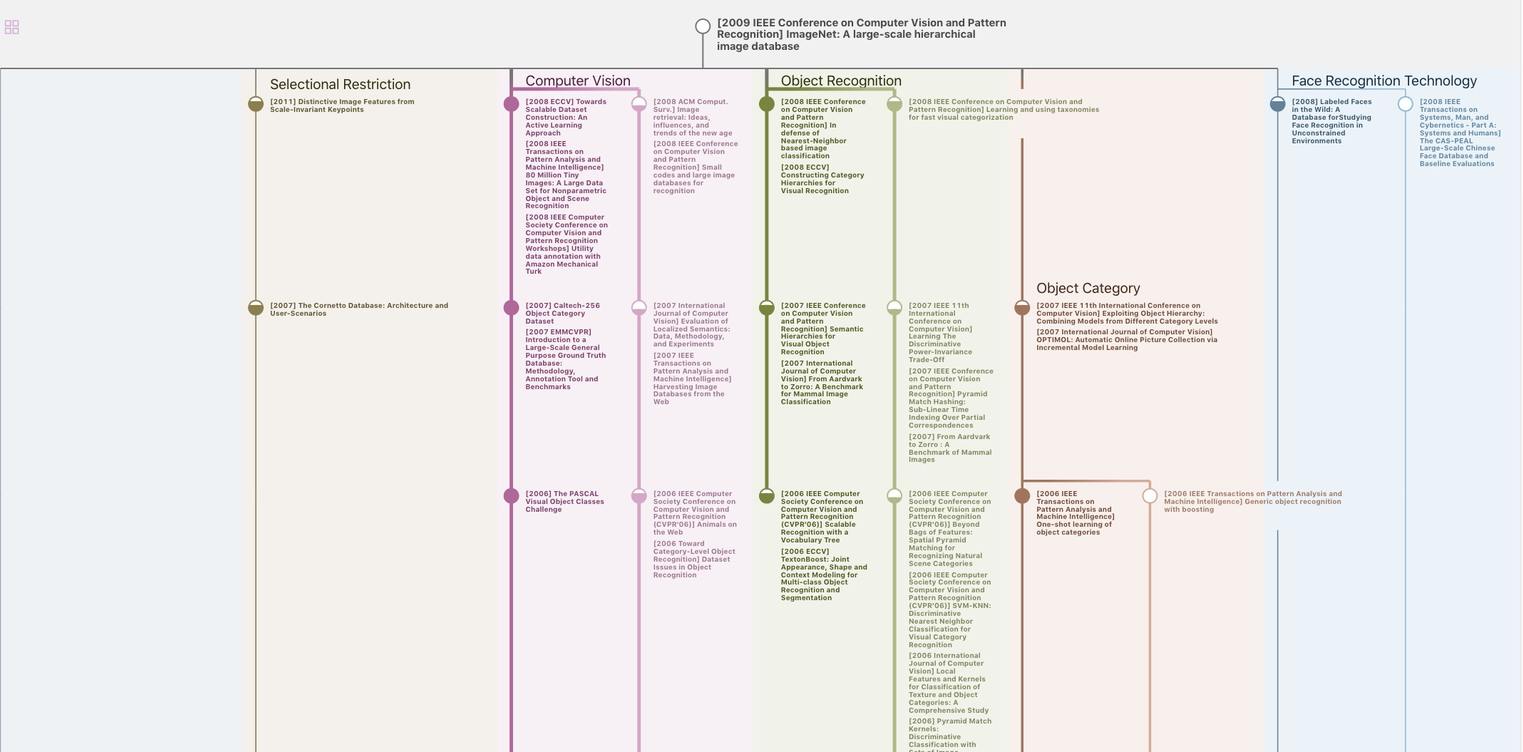
生成溯源树,研究论文发展脉络
Chat Paper
正在生成论文摘要