Modeling and forecasting climate variables using a physical-statistical approach
JOURNAL OF GEOPHYSICAL RESEARCH-ATMOSPHERES(2010)
摘要
In climatology it is common for studies to use either process models derived from physical principles or empirical models, which are rarely combined in any formal way. In part, this is because it is difficult to develop process models for climate variables such as monthly or seasonal rainfall that may be thought of as outputs from complex physical processes. Models for these so-called climate outputs therefore typically use empirical methods, often incorporating modeled data as predictors. Our application is concerned with using simplified models of the El Nino-Southern Oscillation to drive forecasts of climate outputs such as monthly rainfall in southeast Australia. We develop a method to couple an empirical model with a process model in a sequential formulation familiar in data assimilation. This allows us to model climate outputs directly, and it offers potential for building new seasonal forecasting approaches drawing on the strengths of both empirical and physical modeling. It is also easy to update the model as more data become available.
更多查看译文
关键词
process model,physical-statistical model,bayesian hierarchical modeling,el niño-southern oscillation,adaptive metropolis algorithm,particle filter,statistical model,empirical method,global change,bayesian hierarchical model,data assimilation,physical model,data analysis,el nino southern oscillation,seasonality,metropolis algorithm,development process,empirical model
AI 理解论文
溯源树
样例
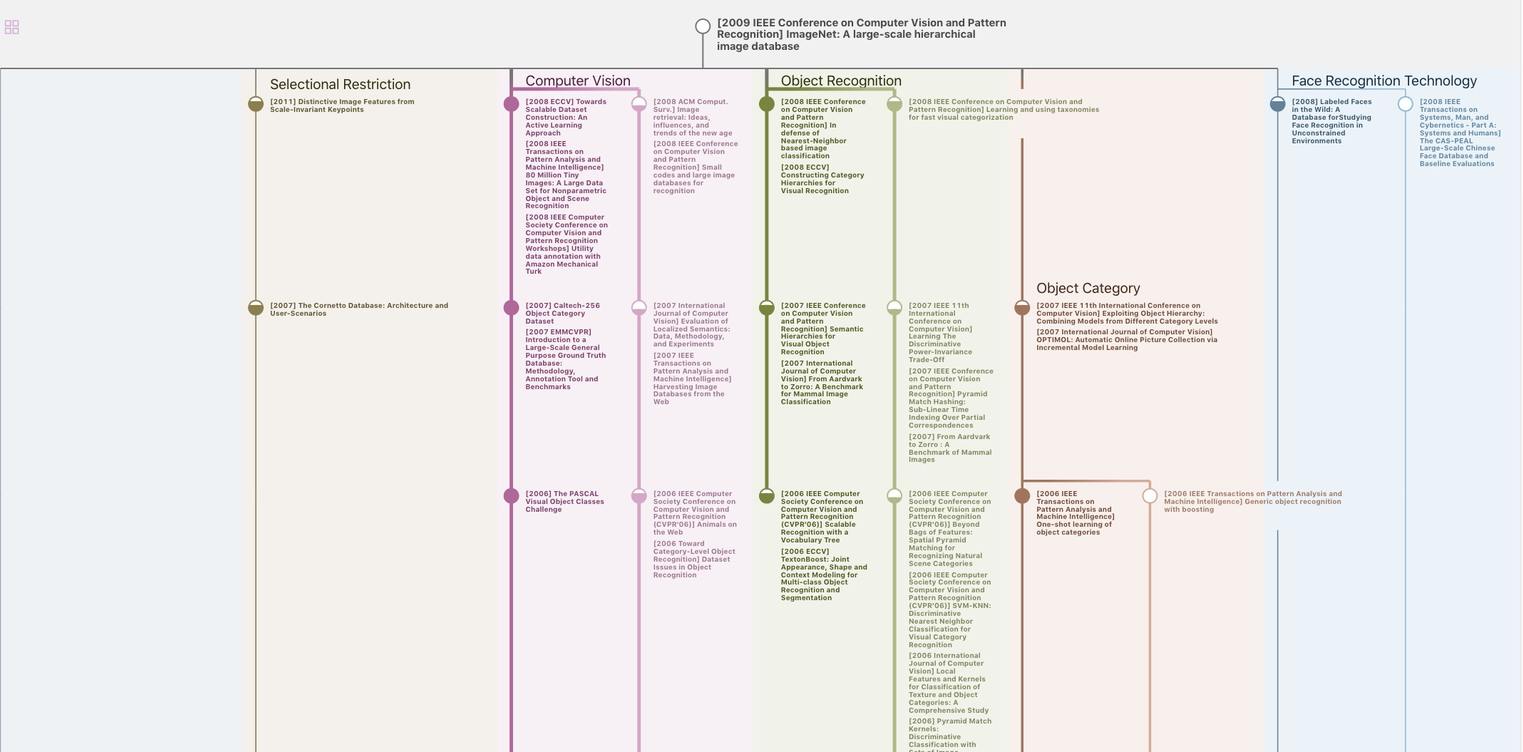
生成溯源树,研究论文发展脉络
Chat Paper
正在生成论文摘要