Efficient and effective (k, TP)-core-based community search over attributed heterogeneous information networks
INFORMATION SCIENCES(2024)
Abstract
Given a heterogeneous information network (HIN) G and a query node q, community search (CS) over an HIN identifies a cohesive subgraph from G that contains q. Although HINs with attributes on nodes (called AHINs) are prevalent today, the CS over AHINs (CS-AHIN) is ignored in the literature. Though we can convert an AHIN to an attributed homogeneous graph given a meta -path, then apply the CS approaches for attributed homogeneous graphs to solve CSAHIN, it is problematic for two reasons. (1) Complete graph conversion is time-consuming and unnecessary, because the search only involves the query node's neighborhood, not the entire graph. (2) Existing attribute cohesiveness metrics are not strict enough to reflect substantial similarities among the community's pairwise nodes. To resolve this, we define the CS-AHIN problem atop a strict attribute cohesiveness metric that supports textual and numerical attributes simultaneously. We show the problem is NP-hard. To address it, we propose an exact baseline to return the global optimal result. Then, we propose three heuristic algorithms using a general greedy search framework to speed up the efficiency. Moreover, we present a cohesiveness-aware proximity graph-based index to boost the performance. Comprehensive experimental studies on various real-world datasets demonstrate our method's superiority.
MoreTranslated text
Key words
Community search,Heterogeneous information network,(k P)-core,Proximity graph
AI Read Science
Must-Reading Tree
Example
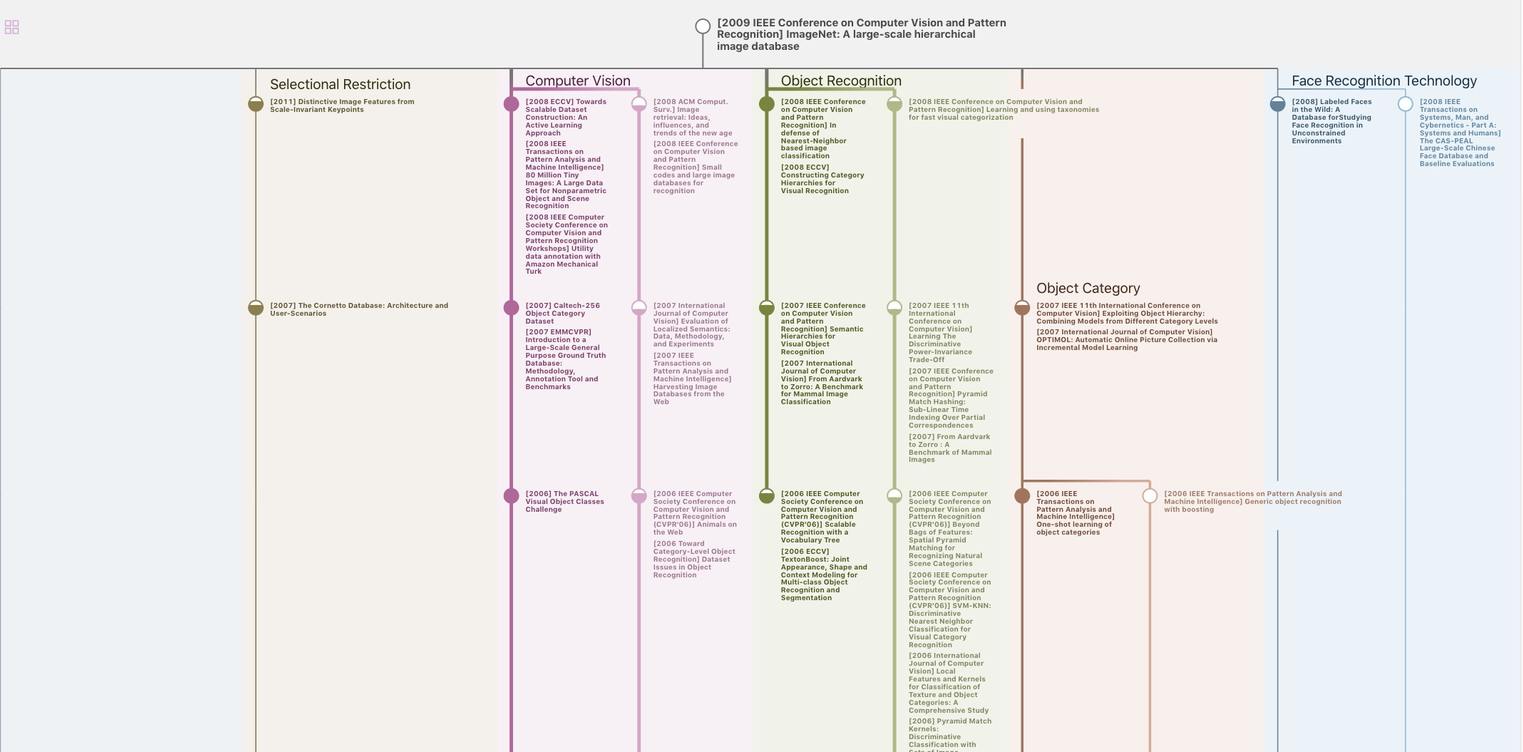
Generate MRT to find the research sequence of this paper
Chat Paper
Summary is being generated by the instructions you defined