Using simulation and deep learning to derive synthetic high resolution daily fire danger maps
Advances in Forest Fire Research 2022(2022)
Abstract
Diagnostic of wildfire danger for the next day typically relies on computation of meteorological indices, usually derived from weather forecast. These indices may represent the danger of ignition, potential rate of spread or droughts, and while it takes into account the vegetation state it do not represent exactly how it will burn, as this is dependent of the fuel distribution and landscape at a scale much finer than weather model allows. On the other hand, overall landscape danger is more and more often evaluated statistically using mass simulation, with a very large number of fire simulated over a variety of weather situation to estimate effective fire area burned (in Ha), reaching a very fine scale at a cost of long computational time. To overcome this computational problem and pinpoint where and when the most critical situation of next day may occurs, We have recently introduced a method, DeepFire, based on deep learning emulation of wildfire simulation to compute these next day fire size distribution an very high temporal and spatial resolution. Nevertheless these simulation comes at a cost, the amount of generated data is large and providing an poses the challenge of creating a synthesis that may actually be helpful in operations. In this study, several approaches are proposed to analyse results and provide fire danger maps and ratings using this new simulation-based prediction system, it is applied to forecasts for 13 relatively large fires that occurred in Corsica and compared to corresponding forecasts using another fire danger index used in operational conditions.
MoreTranslated text
AI Read Science
Must-Reading Tree
Example
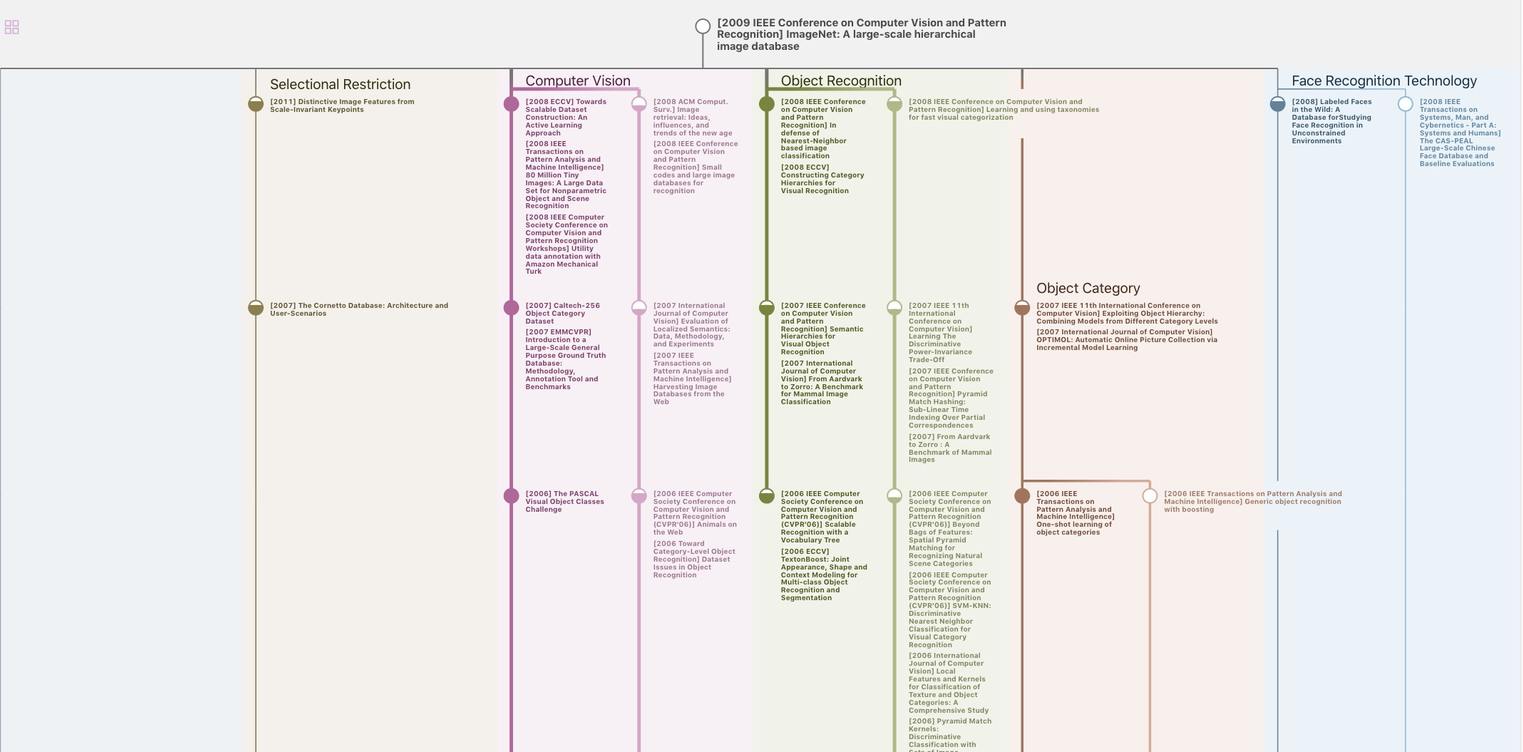
Generate MRT to find the research sequence of this paper
Chat Paper
Summary is being generated by the instructions you defined