Quantitative Uncertainty-Based Incremental Localization And Anchor Selection In Wireless Sensor Networks
MSWiM '11: The 14th ACM International Conference on Modeling, Analysis and Simulation of Wireless and Mobile Systems Miami Florida USA October, 2011(2011)
摘要
Previous localization solutions in wireless sensor networks mainly focus on using various techniques to estimate node positions. In this paper, we argue that quantifying the uncertainty of these estimates is equally important in practice. By using the quantitative uncertainty of measurements and estimates, we can derive more accurate estimates by better fusing the measurements, provide confidence information for confidence-based applications, and know how to select the best anchor nodes so as to minimize the total mean square errors of the whole network. This paper quantifies the estimation uncertainty as an error covariance matrix, and presents an efficient incremental centralized algorithm-INOVA and a decentralized algorithm-OSE-COV for calculating the error covariance matrix. Furthermore, we present how to use the error covariance matrix to infer the confidence region of each node's estimate, and provide an optimal strategy for the anchor selection problem. Extensive simulation results show that INOVA significantly improves the computation efficiency when the network changes dynamically; the confidence region inference is accurate when the measurement number to node number ratio is more than 2; and the optimal anchor selection strategy reduces the total mean square error by four times as much as the variation-based algorithm in best case.
更多查看译文
关键词
wireless sensor network,localization,uncertainty
AI 理解论文
溯源树
样例
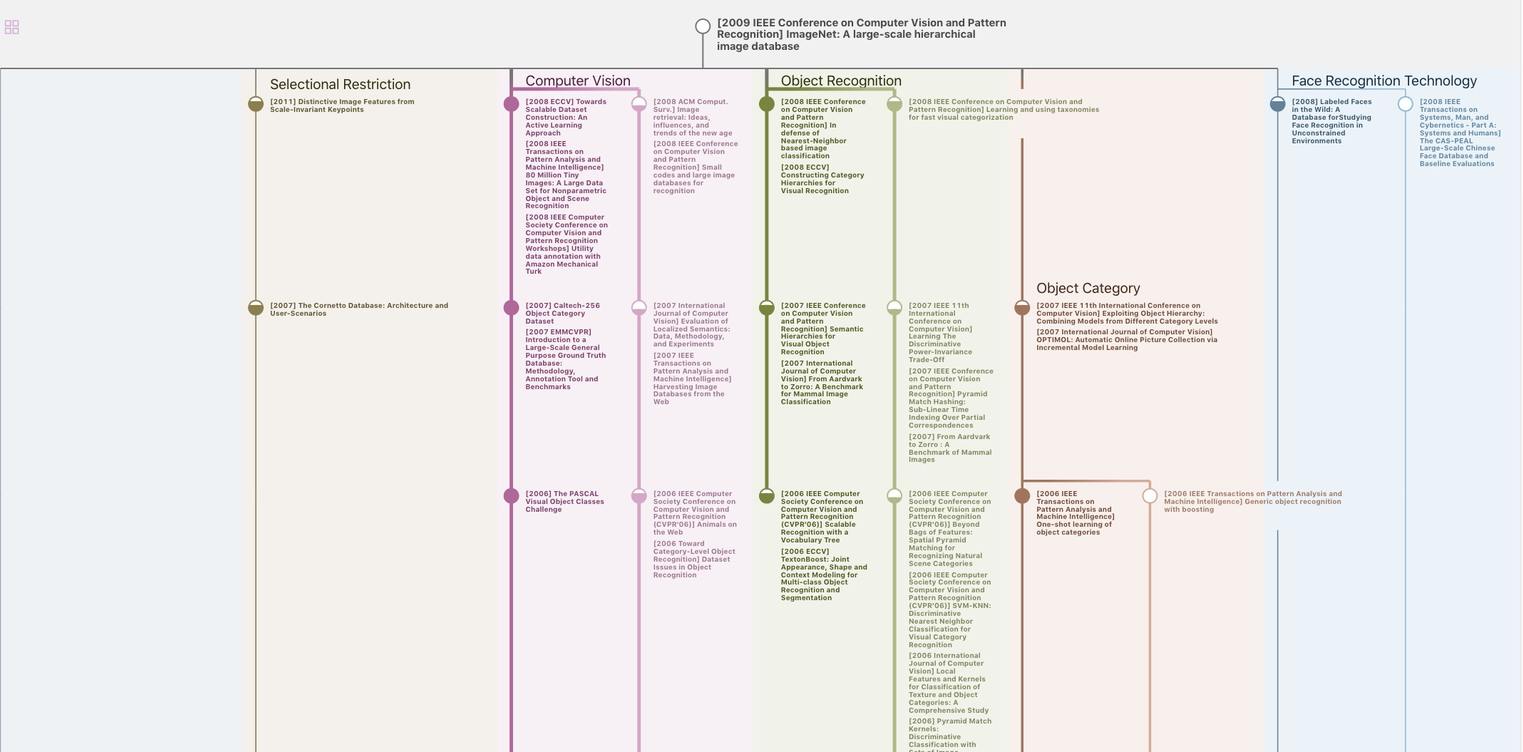
生成溯源树,研究论文发展脉络
Chat Paper
正在生成论文摘要