Trellis-Coded Quantization for End-to-End Learned Image Compression.
ICIP(2022)
Abstract
The performance of variational auto-encoders (VAE) for image compression has steadily grown in recent years, thus becoming competitive with advanced visual data compression technologies. These neural networks transform the source image into a latent space with a channel-wise representation. In most works, the latents are scalar quantized before being entropy coded. On the other hand, vector quantizers generally achieve denser packings of high-dimensional data regardless of the source distribution. Hence, low-complexity variants of these quantizers are implemented in the compression standards JPEG 2000 and Versatile Video Coding. In this paper we demonstrate coding gains by using trellis-coded quantization (TCQ) over scalar quantization. For the optimization of the networks with regard to TCQ, we employ a specific noisy representation of the features during the training stage. For variable-rate VAEs, we obtained 7.7% average BD-rate savings on the Kodak images by using TCQ over scalar quantization. When different networks per target bitrate are optimized, we report a relative coding gain of 2.4% due to TCQ.
MoreTranslated text
Key words
quantization,trellis-coded,end-to-end
AI Read Science
Must-Reading Tree
Example
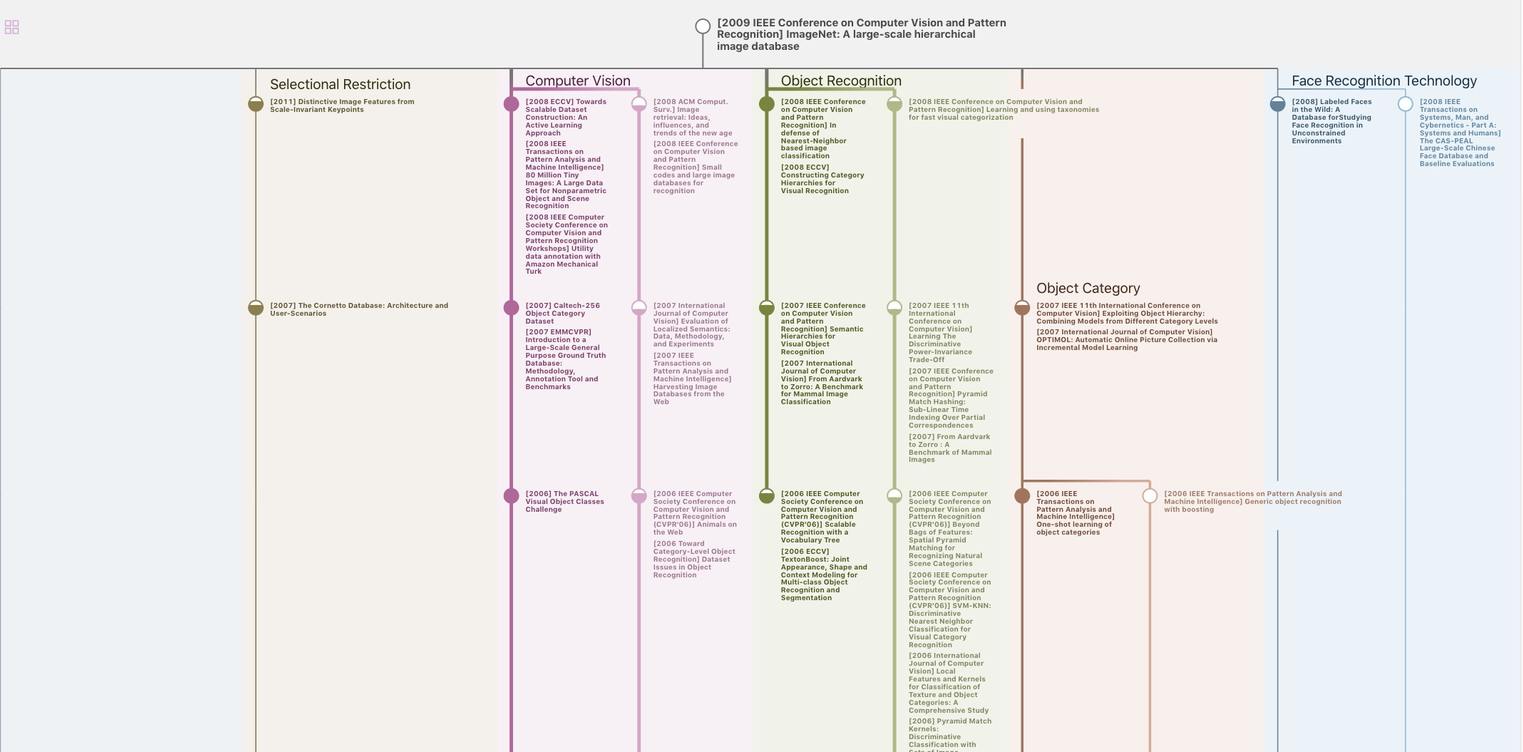
Generate MRT to find the research sequence of this paper
Chat Paper
Summary is being generated by the instructions you defined