Divide and Conquer Kernel Ridge Regression: A Distributed Algorithm with Minimax Optimal Rates
JOURNAL OF MACHINE LEARNING RESEARCH(2015)
摘要
We study a decomposition-based scalable approach to kernel ridge regression, and show that it achieves minimax optimal convergence rates under relatively mild conditions. The method is simple to describe: it randomly partitions a dataset of size N into m subsets of equal size, computes an independent kernel ridge regression estimator for each subset using a careful choice of the regularization parameter, then averages the local solutions into a global predictor. This partitioning leads to a substantial reduction in computation time versus the standard approach of performing kernel ridge regression on all N samples. Our two main theorems establish that despite the computational speed-up, statistical optimality is retained: as long as m is not too large, the partition-based estimator achieves the statistical minimax rate over all estimators using the set of N samples. As concrete examples, our theory guarantees that the number of subsets m may grow nearly linearly for finite-rank or Gaussian kernels and polynomially in N for Sobolev spaces, which in turn allows for substantial reductions in computational cost. We conclude with experiments on both simulated data and a music-prediction task that complement our theoretical results, exhibiting the computational and statistical benefits of our approach.
更多查看译文
关键词
kernel ridge regression,divide and conquer,computation complexity
AI 理解论文
溯源树
样例
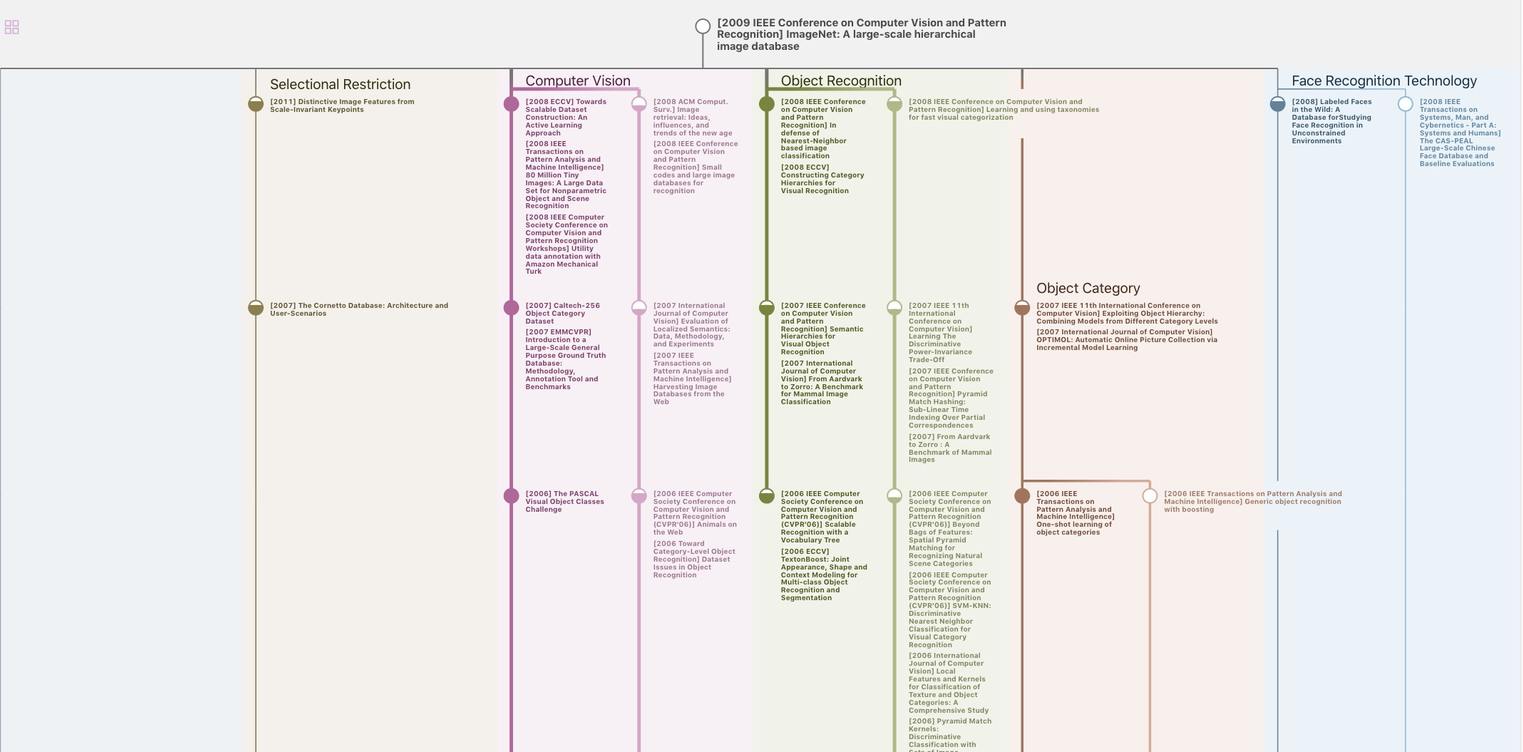
生成溯源树,研究论文发展脉络
Chat Paper
正在生成论文摘要