Application of a Gaussian, Missing-Data Model to Product Recommendation.
IEEE Signal Process. Lett.(2010)
Abstract
A Gaussian, missing-data model is applied to predict product ratings. Vectors of product ratings from users are assumed to be independent and identically distributed. Two approaches for parameter estimation in this model are studied: Little and Rubin's expectation-maximization algorithm and McMichael's modified stochastic gradient descent approach. The resulting estimates are used in minimum mean squared error prediction of product ratings using the conditional mean. On a large dataset, performance using McMichael's approach is better than reported performance of the popular matrix factorization approach.
MoreTranslated text
Key words
data models,recommender system,gaussian model,gradient descent,matrix decomposition,expectation maximization,conditional mean,covariance matrix,predictive models,maximum likelihood,independent and identically distributed,minimum mean square error,expectation maximization algorithm,matrix factorization,stochastic processes,gaussian processes,maximum likelihood estimation,recommender systems,parameter estimation,sparse matrices,stochastic gradient descent,missing data
AI Read Science
Must-Reading Tree
Example
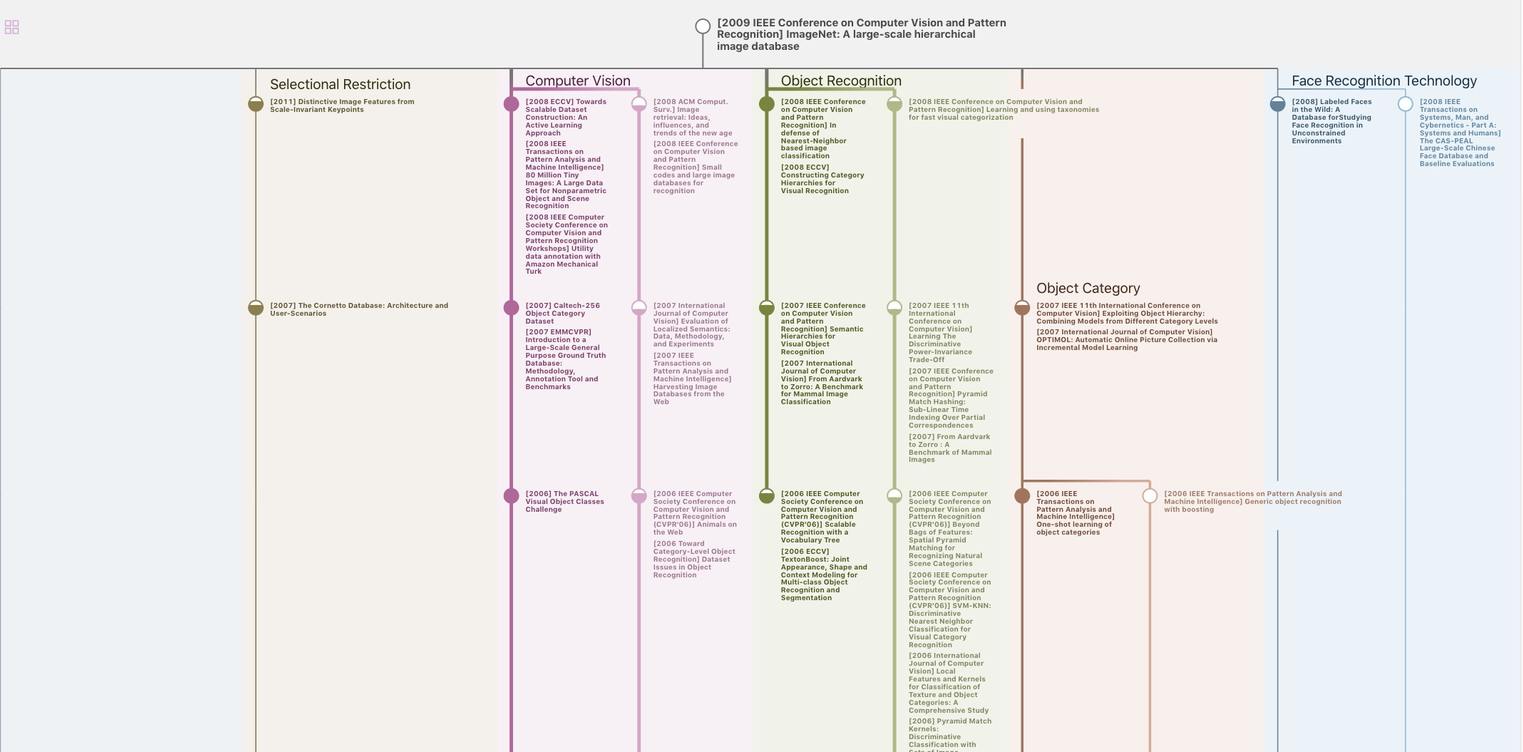
Generate MRT to find the research sequence of this paper
Chat Paper
Summary is being generated by the instructions you defined