Simultaneous Similarity Learning and Feature-Weight Learning for Document Clustering.
TextGraphs-6: Proceedings of TextGraphs-6: Graph-based Methods for Natural Language Processing(2011)
摘要
A key problem in document classification and clustering is learning the similarity between documents. Traditional approaches include estimating similarity between feature vectors of documents where the vectors are computed using TF-IDF in the bag-of-words model. However, these approaches do not work well when either similar documents do not use the same vocabulary or the feature vectors are not estimated correctly. In this paper, we represent documents and keywords using multiple layers of connected graphs. We pose the problem of simultaneously learning similarity between documents and keyword weights as an edge-weight regularization problem over the different layers of graphs. Unlike most feature weight learning algorithms, we propose an unsupervised algorithm in the proposed framework to simultaneously optimize similarity and the keyword weights. We extrinsically evaluate the performance of the proposed similarity measure on two different tasks, clustering and classification. The proposed similarity measure outperforms the similarity measure proposed by (Muthukrishnan et al., 2010), a state-of-the-art classification algorithm (Zhou and Burges, 2007) and three different baselines on a variety of standard, large data sets.
更多查看译文
关键词
proposed similarity measure,feature vector,keyword weight,optimize similarity,similarity measure,proposed framework,different baselines,different layer,different task,document classification,feature-weight learning,document clustering,simultaneous similarity
AI 理解论文
溯源树
样例
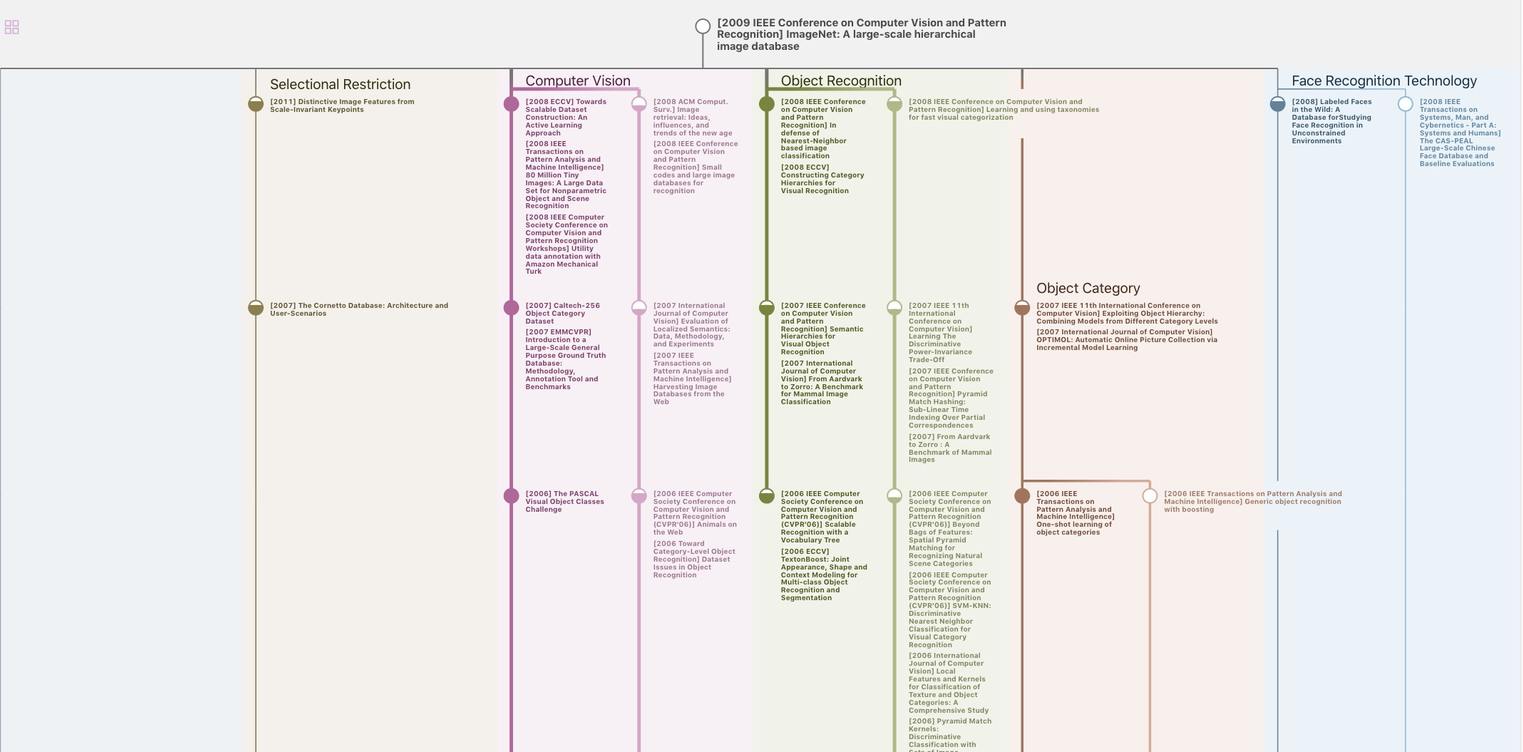
生成溯源树,研究论文发展脉络
Chat Paper
正在生成论文摘要