Metric Learning for Estimating Psychological Similarities
ACM TIST(2012)
摘要
An important problem in cognitive psychology is to quantify the perceived similarities between stimuli. Previous work attempted to address this problem with multidimensional scaling (MDS) and its variants. However, there are several shortcomings of the MDS approaches. We propose Yada, a novel general metric-learning procedure based on two-alternative forced-choice behavioral experiments. Our method learns forward and backward nonlinear mappings between an objective space in which the stimuli are defined by the standard feature vector representation and a subjective space in which the distance between a pair of stimuli corresponds to their perceived similarity. We conduct experiments on both synthetic and real human behavioral datasets to assess the effectiveness of Yada. The results show that Yada outperforms several standard embedding and metric-learning algorithms, both in terms of likelihood and recovery error.
更多查看译文
关键词
standard embedding,estimating psychological similarities,important problem,stimuli corresponds,objective space,metric-learning algorithm,mds approach,subjective space,standard feature vector representation,metric learning,real human behavioral datasets,novel general metric-learning procedure,feature vector,embedding,human behavior,cognitive psychology,multidimensional scaling
AI 理解论文
溯源树
样例
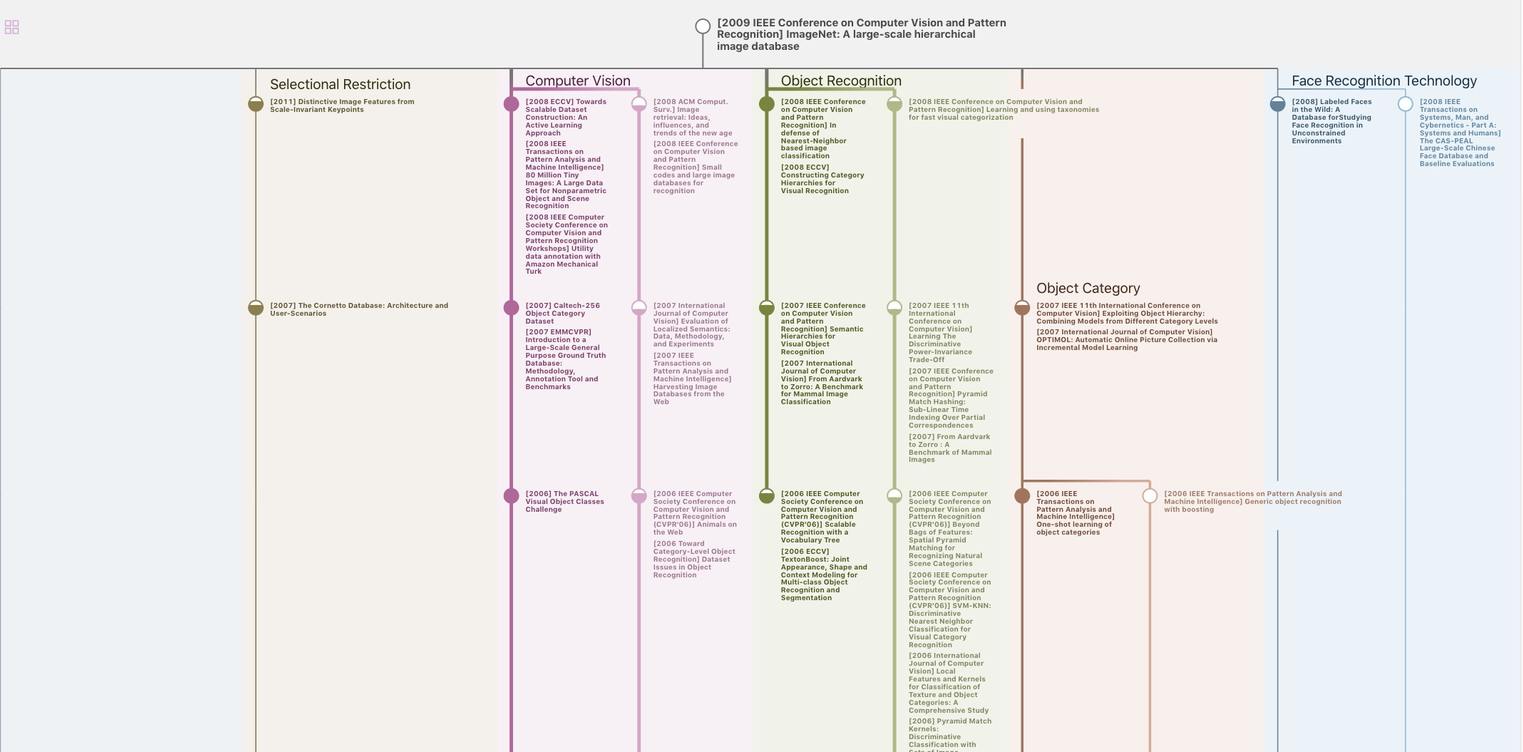
生成溯源树,研究论文发展脉络
Chat Paper
正在生成论文摘要