Prostate And Bladder Segmentation Using A Statistically Trainable Model
INTERNATIONAL JOURNAL OF RADIATION ONCOLOGY BIOLOGY PHYSICS(2007)
摘要
Statistically trainable deformable-shape models (SDSMs) of anatomic objects show promise for segmentation by automatically deforming in a target image to closely match target anatomy. The purpose was to establish benchmarks for comparison with clinical studies for a particular class of SDSMs called m-reps in segmenting the prostate and bladder for IGRT/ART. Approximately 190 CT treatment images for 13 patients undergoing IGRT for prostate cancer were manually contoured by an expert. M-rep models were fit to the contours and statistically analyzed to compute a probability distribution yielding a mean and day-to-day position and shape variability for each patient's bladder and prostate. Each fitted m-rep also was registered with its corresponding image to collect, in m-rep coordinates, histograms of image intensities in predefined local regions inside and around the prostate and bladder. The histograms were processed to yield probability distributions on anatomy-relative image intensity patterns. In a leave-one-image-out experiment, mean m-rep models were applied to each treatment image for each set of patient images, where the target image was excluded from training data. The prostate m-rep was initialized in each target image via an automatically computed transformation matrix that registered the target image with the first treatment image. Due to its wide shape variability the bladder m-rep was initialized via three manually drawn contours near the base, middle and superior sections. The deformations were automatically driven by an algorithm that optimized an objective function with terms for the geometric and intensity probability distributions described above. These studies included global and regional deformations for the prostate, and global, regional and local deformations for the bladder. For the prostate the median volume overlap between m-reps and the human expert was 93% for both the prostate and bladder. The median of the average surface separation between m-rep and human segmentations over all cases was 1.1 mm for the prostate, and 1.3 mm for the bladder. This level of agreement is at voxel scale and is better than two human experts would agree. M-rep optimization mimics contouring by a human expert against which m-reps have been trained. Moreover, assuming intra-observer variabilities are similar across experts, special properties of m-reps allow the position and shape variabilities to apply to a mean model estimated by another expert, e.g., by manual contouring the planning image, without additional training. To encourage open comparison and publication of segmentation results, images and associated data sets used in this study are available to other investigators.
更多查看译文
关键词
bladder segmentation,prostate,model
AI 理解论文
溯源树
样例
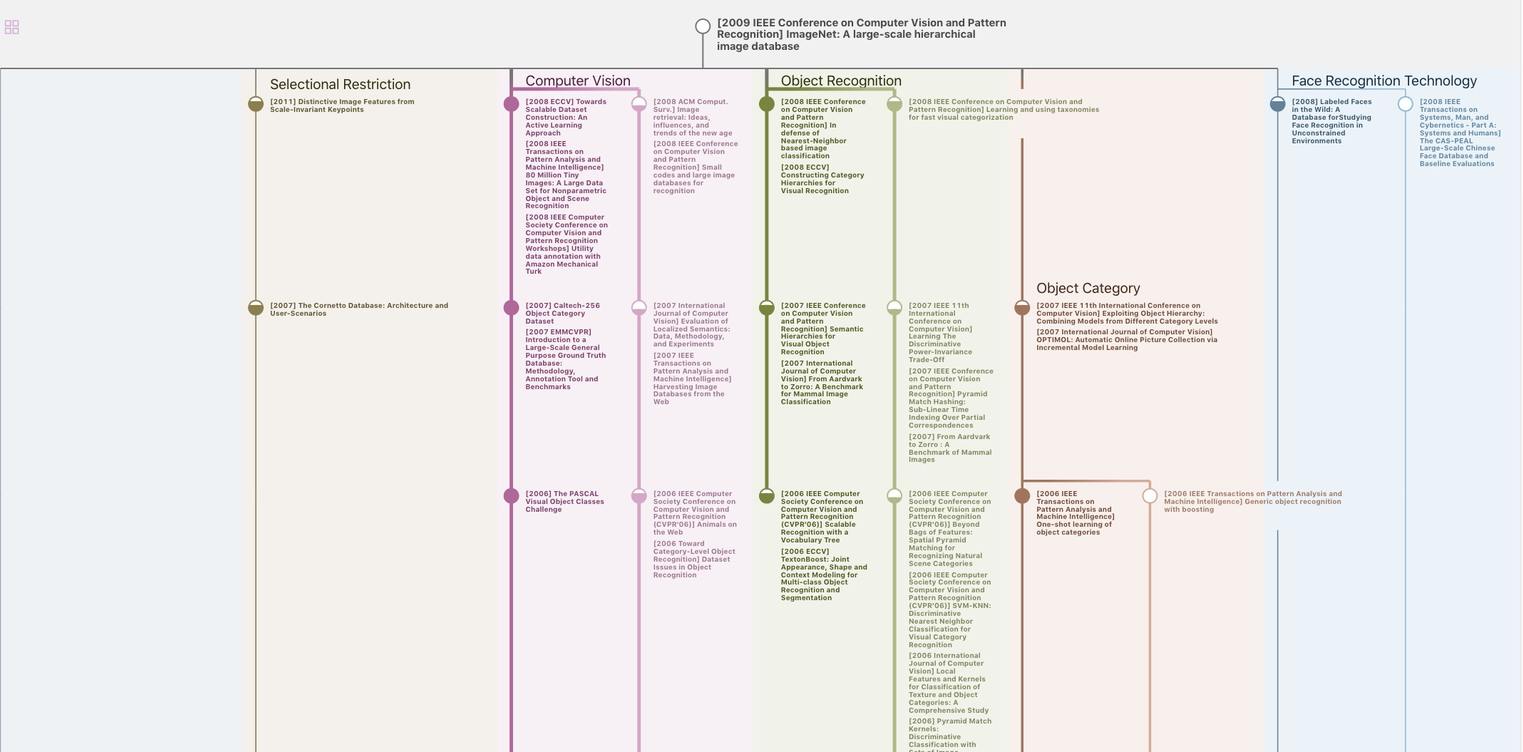
生成溯源树,研究论文发展脉络
Chat Paper
正在生成论文摘要