Practical large-scale spatio-temporal modeling of particulate matter concentrations
ANNALS OF APPLIED STATISTICS(2009)
摘要
The last two decades have seen intense scientific and regulatory interest in the health effects of particulate matter (PM). Influential epidemiological studies that characterize chronic exposure of individuals rely on monitoring data that are sparse in space and time, so they often assign the same exposure to participants in large geographic areas and across time. We estimate monthly PM during 1988-2002 in a large spatial domain for use in studying health effects in the Nurses' Health Study. We develop a conceptually simple spatio-temporal model that uses a rich set of covariates. The model is used to estimate concentrations of PM10 for the full time period and PM2.5 for a subset of the period. For the earlier part of the period, 1988-1998, few PM2.5 monitors were operating, so we develop a simple extension to the model that represents PM2.5 conditionally on PM10 model predictions. In the epidemiological analysis, model predictions of PM10 are more strongly associated with health effects than when using simpler approaches to estimate exposure. Our modeling approach supports the application in estimating both fine-scale and large-scale spatial heterogeneity and capturing space-time interaction through the use of monthly-varying spatial surfaces. At the same time, the model is computationally feasible, implementable with standard software, and readily understandable to the scientific audience. Despite simplifying assumptions, the model has good predictive performance and uncertainty characterization.
更多查看译文
关键词
Additive model,air pollution,epidemiology,geoadditive model,smoothing,kriging,backfitting,stochastic EM
AI 理解论文
溯源树
样例
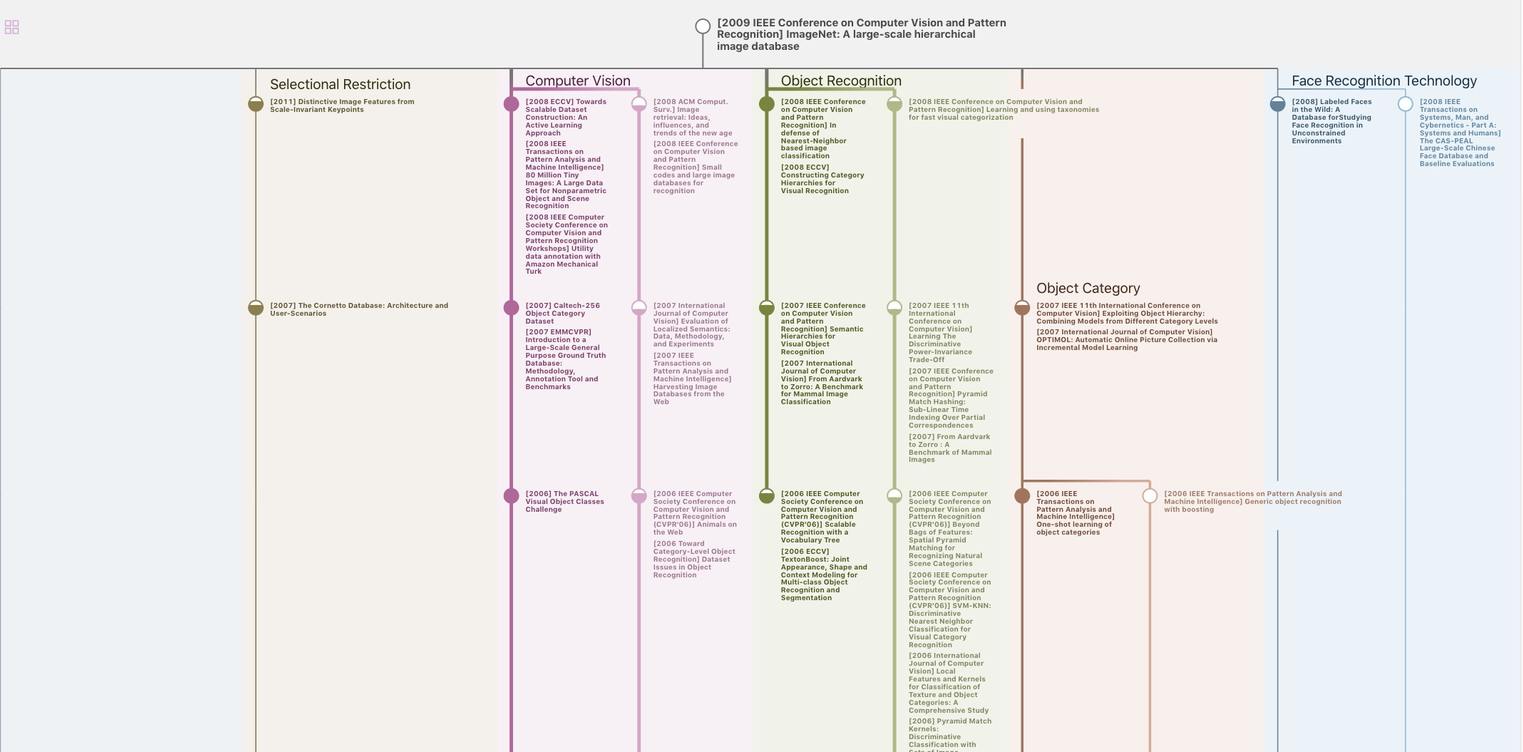
生成溯源树,研究论文发展脉络
Chat Paper
正在生成论文摘要