High-Accuracy Object Recognition with a New Convolutional Net Architecture and Learning Algorithm
msra(2014)
摘要
abstract describes a modified ConvNet architecture with a ne w unsupervised/supervised train- ing procedure that can reach 67.2% accuracy on Caltech-101. This work,explores several architectural designs and training methods,and studies their effect on the accuracy for object recognition. The convolutional network under consideration takes a 143x143 grayscale image as input. The preprocessing includes removing,mean,and performing a local contrast normalization (dividing each pixel by the standard deviation of its neighbors). The first stage has 64 filters of size 9x9, followe d by a subsampling layer with 5x5 stride, and 10x10 averaging window. The second stage has 256 feature map, each with 16 filters connected to a random,subset of first-layer feature ma,ps. The subsampling,layer has a stride of 4x4 and a 6x6 averaging window. Hence, the input to the last layer has 256 feature maps of size 4x4 (4096 dimensions). Figure 1 shows the outline of a convolutional net, and figure 2 shows the best sequence of transformations at each st age of the network. The results are shown in table . The most surprising result is that simply adding an absolute value after the hyperbolic tangent (tanh) non-linearity pr actically doubles the recognition rate from 26% to 58% with purely supervised training. We conjecture that the advantage of a rectifying non-linearity is to remove redundant informati on (the polarity of features), and at the same time, to avoids cancellations of neighboring opposite filter responses in the subsampling layers. Adding a local contrast normalization step after ea ch feature extraction layer [4] further improves the accuracy to 60%. The second interesting result is that pre-training each sta ge one after the other using a new unsupervised method, and adjusting the resulting network using supervised gradient descent bumps,up the accuracy to 67.2%. The procedure is reminiscent of several recent proposal for “deep learning” [2, 3]. Our layer-wise unsupervised training method is called Predictive Sparse
更多查看译文
AI 理解论文
溯源树
样例
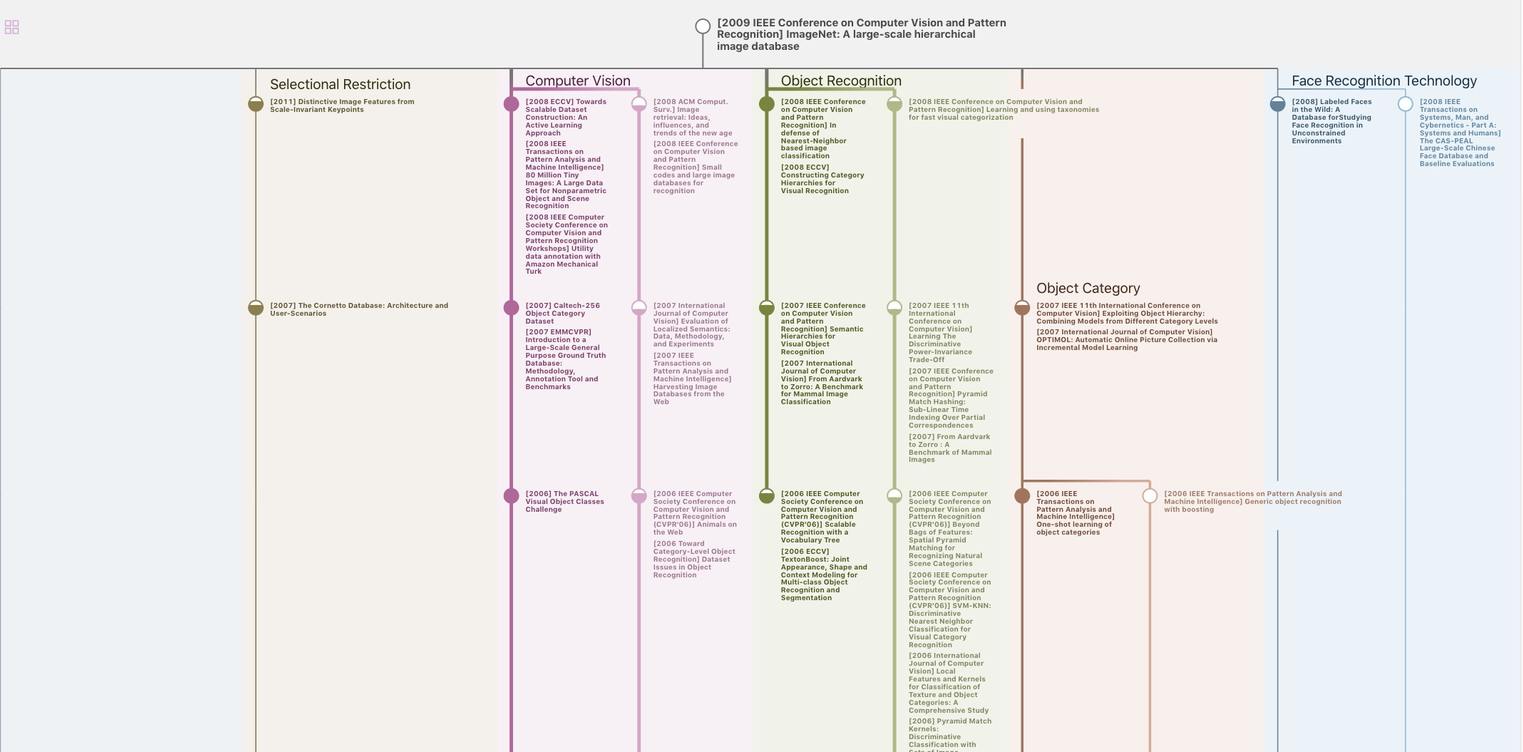
生成溯源树,研究论文发展脉络
Chat Paper
正在生成论文摘要