A Powerful and Precise Feature-level Filter using Group Knockoffs
arxiv(2024)
摘要
Selecting important features that have substantial effects on the response
with provable type-I error rate control is a fundamental concern in statistics,
with wide-ranging practical applications. Existing knockoff filters, although
shown to provide theoretical guarantee on false discovery rate (FDR) control,
often struggle to strike a balance between high power and precision in
pinpointing important features when there exist large groups of strongly
correlated features. To address this challenge, we develop a new filter using
group knockoffs to achieve both powerful and precise selection of important
features. Via experiments of simulated data and analysis of a real Alzheimer's
disease genetic dataset, it is found that the proposed filter can not only
control the proportion of false discoveries but also identify important
features with comparable power and greater precision than the existing group
knockoffs filter.
更多查看译文
AI 理解论文
溯源树
样例
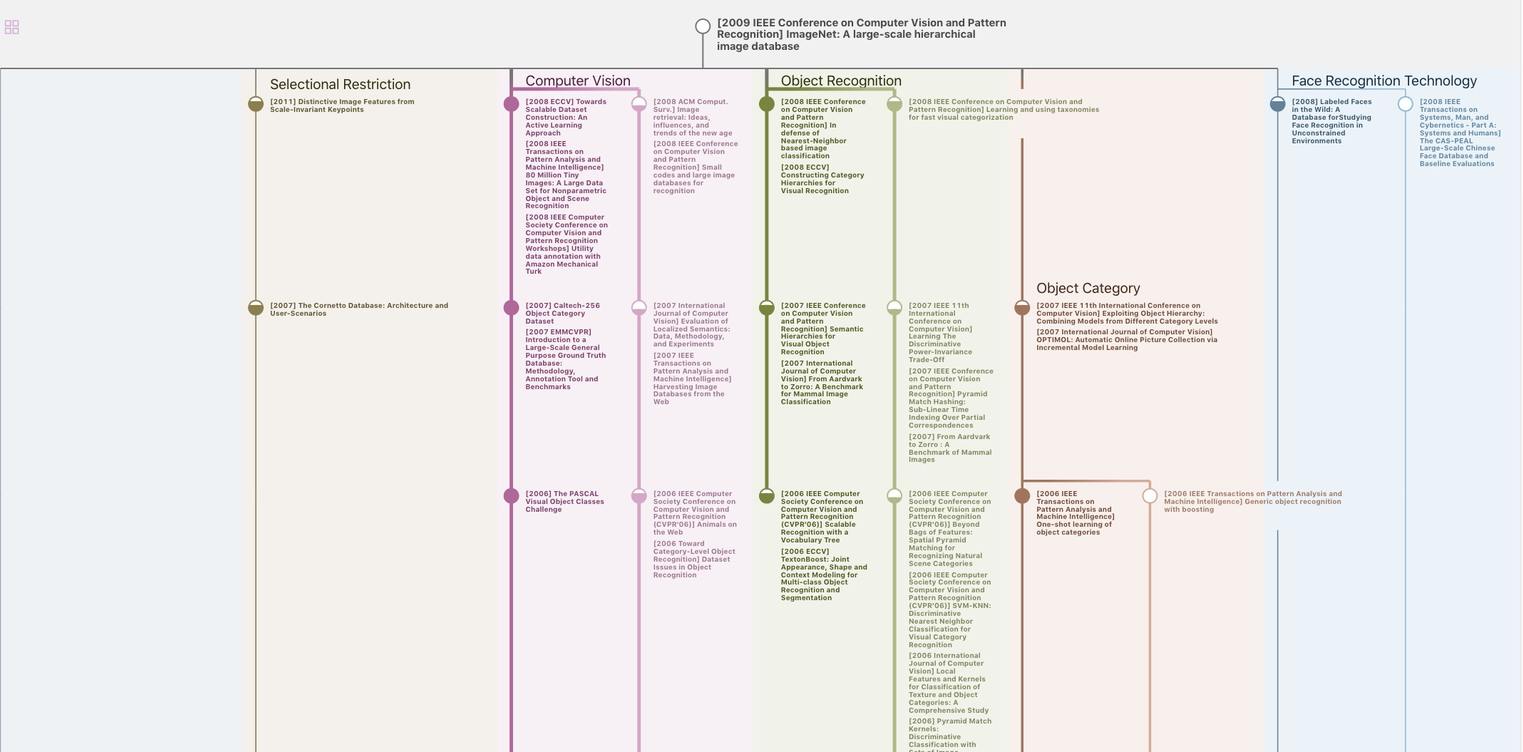
生成溯源树,研究论文发展脉络
Chat Paper
正在生成论文摘要