Unsupervised Methods To Identify Cellular Signaling Networks From Perturbation Data
BIOINFORMATICS: CONCEPTS, METHODOLOGIES, TOOLS, AND APPLICATIONS(2013)
摘要
The inference of cellular architectures from detailed time-series measurements of intracellular variables is an active area of research. High throughput measurements of responses to cellular perturbations are usually analyzed using a variety of machine learning methods that typically only work within one type of measurement. Here, summaries of some recent research attempts are presented-these studies have expanded the scope of the problem by systematically integrating measurements across multiple layers of regulation including second messengers, protein phosphorylation markers, transcript levels, and functional phenotypes into signaling vectors or signatures of signal transduction. Data analyses through simple unsupervised methods provide rich insight into the biology of the underlying network, and in some cases reconstruction of key architectures of the underlying network from perturbation data. The methodological advantages provided by these efforts are examined using data from a publicly available database of responses to systematic perturbations of cellular signaling networks generated by the Alliance for Cellular Signaling (AfCS).
更多查看译文
AI 理解论文
溯源树
样例
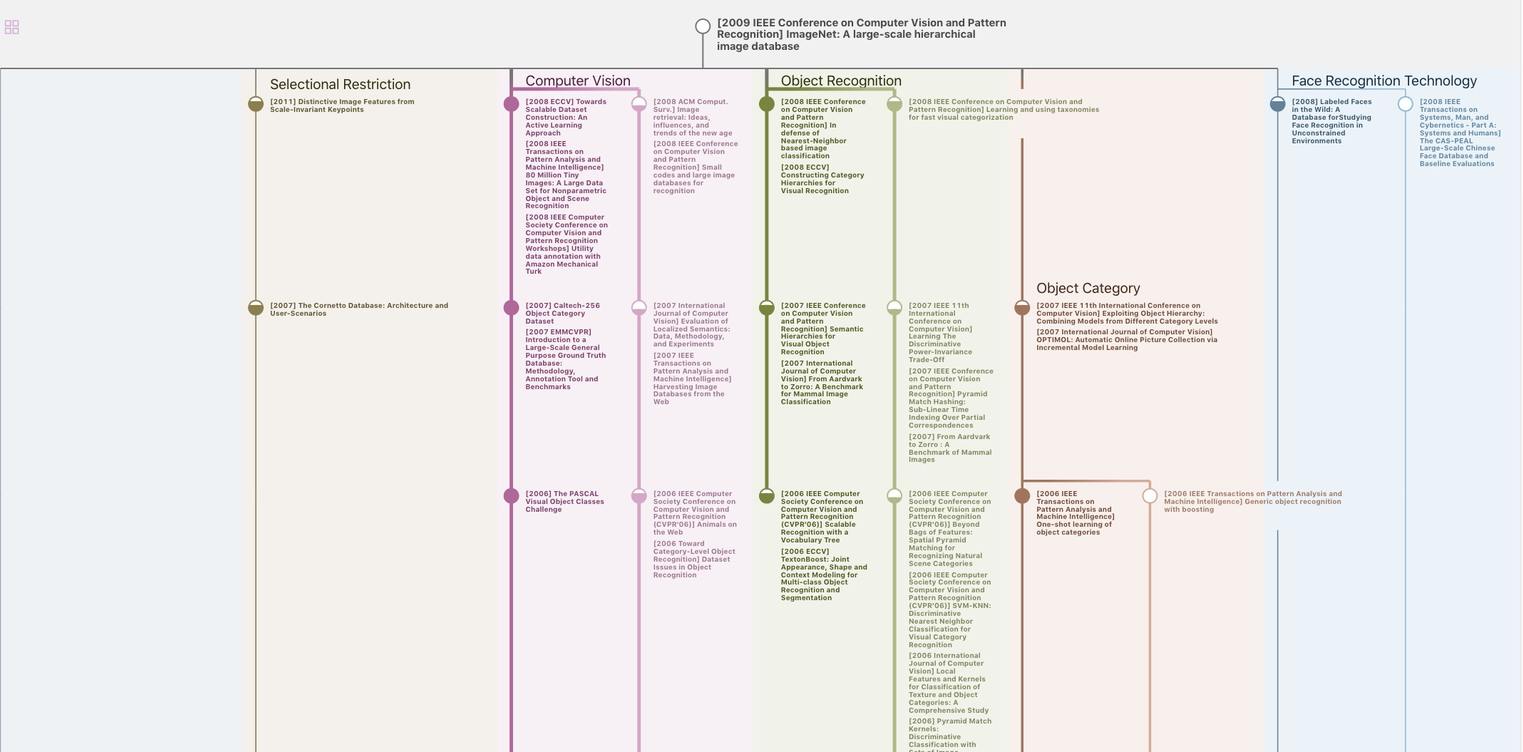
生成溯源树,研究论文发展脉络
Chat Paper
正在生成论文摘要