An efficient point sampling algorithm for train bottom key component detection
TWELFTH INTERNATIONAL CONFERENCE ON INFORMATION OPTICS AND PHOTONICS (CIOP 2021)(2021)
摘要
With the increasing resolution of 3D laser scanners, the number of points in 3D point cloud becoming huge. For example,the number of points in the point cloud that collected form key components of train bottom usually reaches hundreds of thousands to millions. Generally, we would down sample the point cloud before the recognition, registration and reconstruction, which means using a simplified point set with less amount of points to represent the raw data. The Farthest Point Sampling algorithm (FPS) has been proven to be able to traverse the entire point cloud well by uniform sampling. However, it takes a long time for iterative calculation so that the calculation efficiency of this sampling algorithm performed not very well when the amount of points is large, whether it is used for deep learning or using handcraft algorithms for processing, it takes too much time in the sampling process. Therefore, this paper purposed a faster algorithm based on FPS that dividing the point cloud into several grids by octree, then allocating the number of sampling points in each grid according to the density of the points in the grids. The experimental results show that its running time is greatly reduced , and the calculation efficiency is significantly improved. Additionally, the results of the sampling points could also traverse the raw data and retain enough features for meanwhile. It can be practically applied to process the Traction rod device, Nut, Bolt and Wheelset or the other 3D point cloud of key components of train bottom definitely , making detection more efficient.
更多查看译文
关键词
Point cloud,key components of train bottom,Farthest Point Sampling,octree
AI 理解论文
溯源树
样例
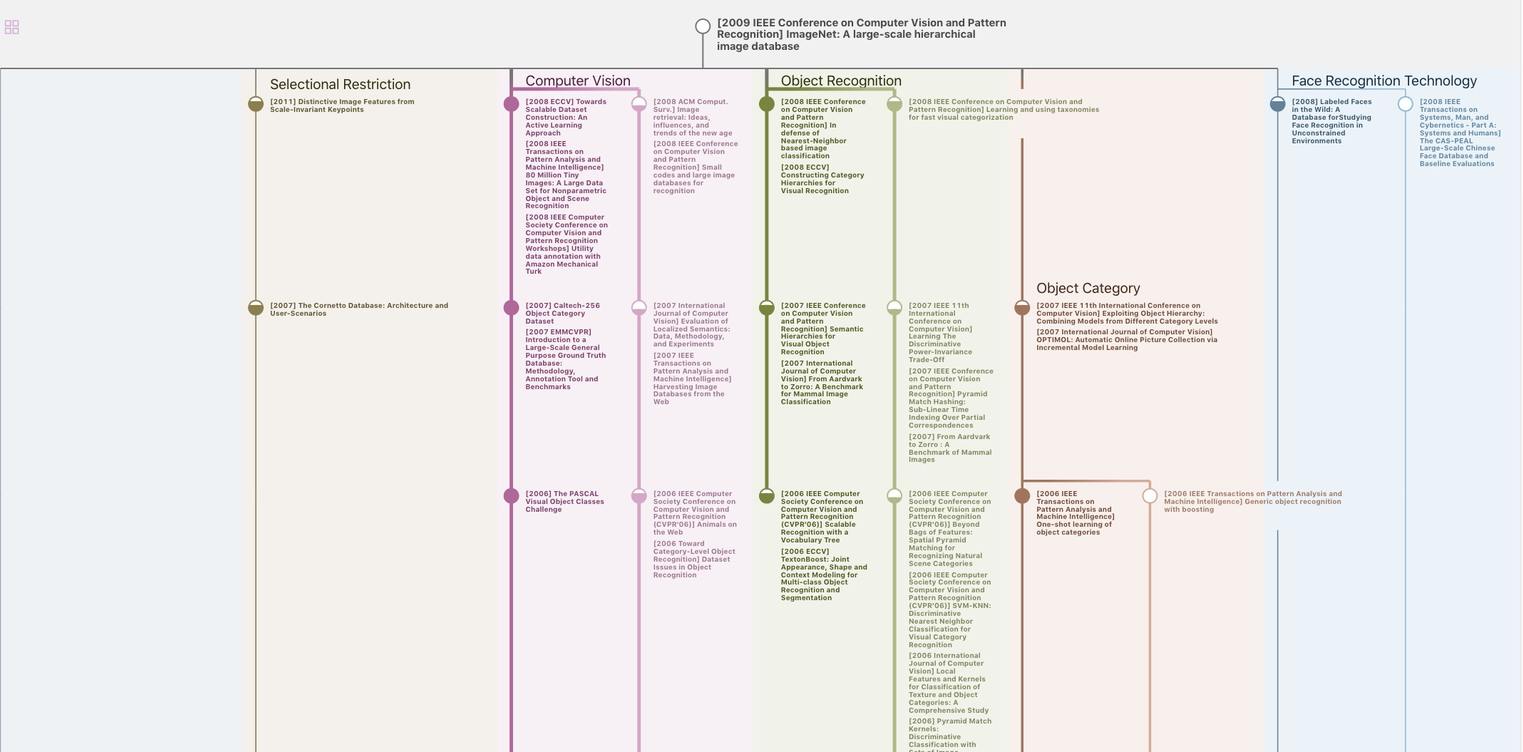
生成溯源树,研究论文发展脉络
Chat Paper
正在生成论文摘要