Pose Invariant Deformable Shape Priors Using L1 Higher Order Sparse Graphs
ISVC (1)(2013)
摘要
In this paper we propose a novel method for knowledge-based segmentation. We adopt a point distribution graphical model formulation which encodes pose invariant shape priors through L 1 sparse higher order cliques. Local shape deformation properties of the model can be captured and learned in an optimal manner from a training set using dual decomposition. These higher order shape terms are combined with conventional visual ones aiming at maximizing the posterior segmentation likelihood. The considered graphical model is optimized using dual decomposition and is used towards 2D computer vision and 3D object segmentation medical imaging with promising results.
更多查看译文
关键词
Markov Random Fields, Active Shape Model, Dissimilarity Function, Shape Prior, Dual Decomposition
AI 理解论文
溯源树
样例
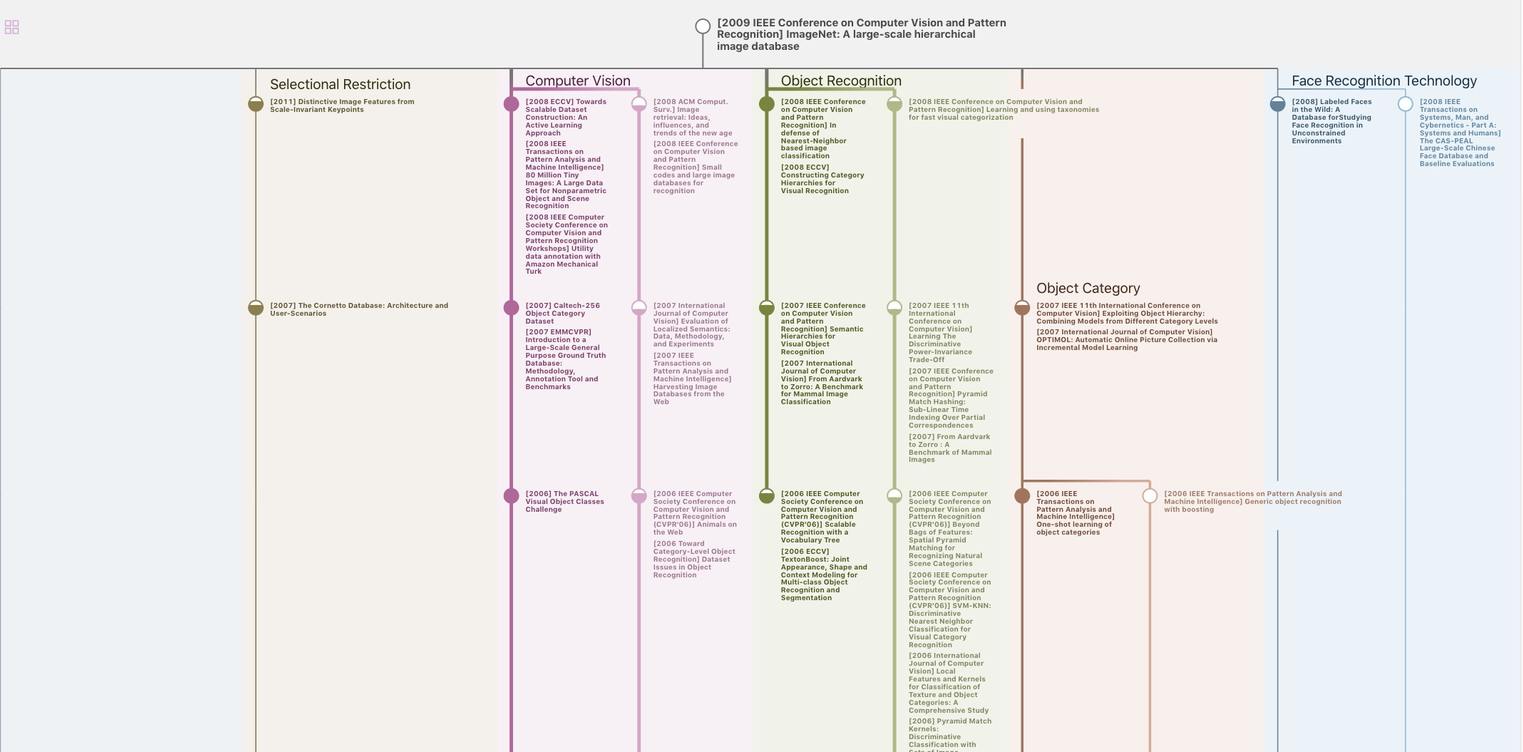
生成溯源树,研究论文发展脉络
Chat Paper
正在生成论文摘要