Bayesian calibration of mathematical models: Optimization of model structure and examination of the role of process error covariance.
Ecological Informatics(2013)
摘要
The integration of Bayesian inference techniques with mathematical modeling offers a promising means to improve ecological forecasts and management actions over space and time, while accounting for the uncertainty underlying model predictions. In this study, we address two important questions related to the ramifications of the statistical assumptions typically made about the model structural error and the prospect of Bayesian calibration to guide the optimization of model complexity. Regarding the former issue, we examine statistical formulations that whether postulate conditional independence or explicitly accommodate the covariance among the error terms for various model endpoints. Our analysis evaluates the differences in the posterior parameter patterns and predictive performance of a limiting nutrient (phosphate)–phytoplankton–zooplankton–detritus (particulate phosphorus) model calibrated with three alternative statistical configurations. The lessons learned from this exercise are combined with those from a second comparative analysis that aims to optimize model structure. In particular, we selected three formulas of the zooplankton mortality term (linear, hyperbolic, sigmoidal) and examine their capacity to determine the posterior parameterization as well as the reproduction of the observed ecosystem patterns. Our analysis suggests that the statistical characterization of the model error as well as the mathematical representation of specific ecological processes can be influential to the inference drawn by a modeling exercise. Our findings could be useful when selecting the most suitable statistical framework for model calibration and/or making informative decisions about model structure optimization. In the absence of adequate prior knowledge, we also advocate the use of Bayesian model averaging for obtaining weighted averages of the forecasts from different model structures and/or statistical descriptions of the process error terms.
更多查看译文
关键词
Process-based modeling,Eutrophication,Bayesian inference,Process error covariance,Model averaging,Model complexity optimization
AI 理解论文
溯源树
样例
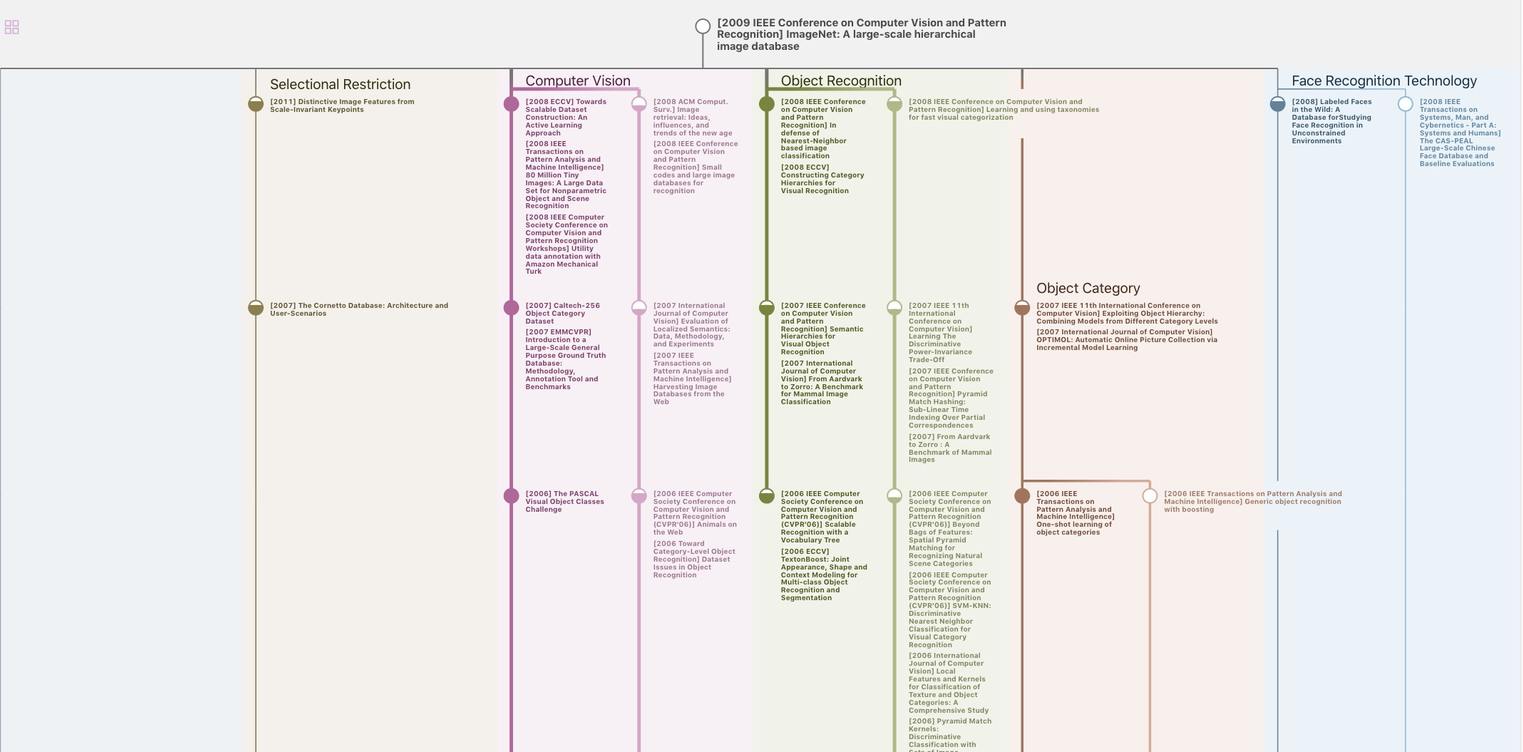
生成溯源树,研究论文发展脉络
Chat Paper
正在生成论文摘要