Effects of Degradations on Deep Neural Network Architectures
arXiv: Computer Vision and Pattern Recognition(2018)
摘要
Deep convolutional neural networks (CNN) have massively influenced recent advances in large-scale image classification. More recently, a dynamic routing algorithm with capsules (groups of neurons) has shown state-of-the-art recognition performance. However, the behavior of such networks in the presence of a degrading signal (noise) is mostly unexplored. An analytical study on different network architectures toward noise robustness is essential for selecting the appropriate model in a specific application scenario. This paper presents an extensive performance analysis of six deep architectures for image classification on six most common image degradation models. In this study, we have compared VGG-16, VGG-19, ResNet-50, Inception-v3, MobileNet and CapsuleNet architectures on Gaussian white, Gaussian color, salt-and-pepper, Gaussian blur, motion blur and JPEG compression noise models.
更多查看译文
AI 理解论文
溯源树
样例
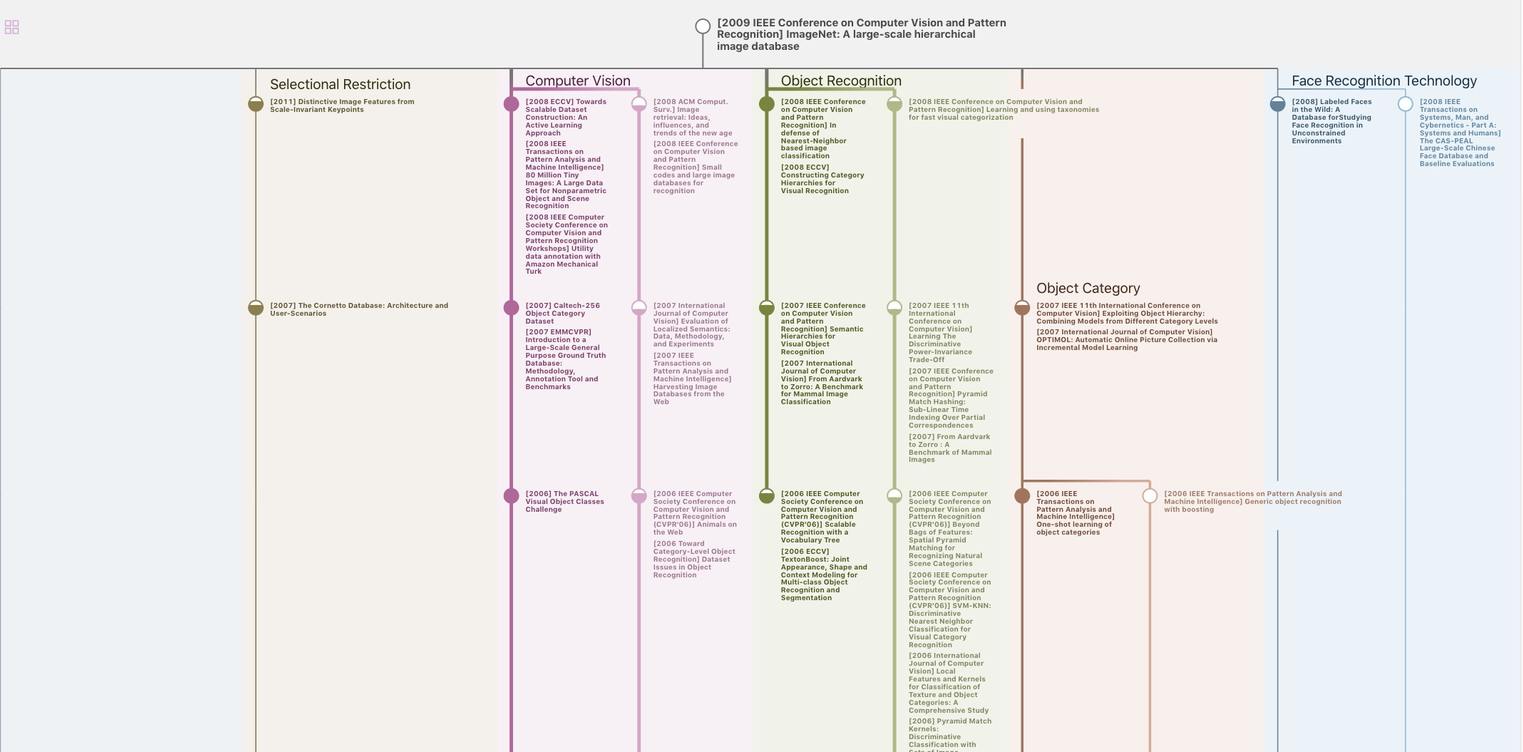
生成溯源树,研究论文发展脉络
Chat Paper
正在生成论文摘要