Reverse engineering of gene regulation models from multi-condition experiments
Computational Intelligence in Bioinformatics and Computational Biology(2013)
摘要
Reverse-engineering of quantitative, dynamic gene-regulatory network (GRN) models from time-series gene expression data is becoming important as such data are increasingly generated for research and other purposes. A key problem in the reverse-engineering process is the under-determined nature of these data. Because of this, the reverse-engineered GRN models often lack robustness and perform poorly when used to simulate system responses to new conditions. In this study, we present a novel method capable of inferring robust GRN models from multi-condition GRN experiments. This study uses two important computational intelligence methods: artificial neural networks and particle swarm optimization.
更多查看译文
关键词
biological techniques,biology computing,genetics,neural nets,particle swarm optimisation,reverse engineering,artificial neural network,computational intelligence method,data generation,data under-determined nature,dynamic GRN model,gene regulation model,model performance,model robustness,multicondition GRN experiment,multicondition experiment,particle swarm optimization,quantitative gene-regulatory network model,reverse engineering,reverse-engineered GRN model,robust GRN model inferring,system response simulation,time-series gene expression data,Gene regulatory networks,machine learning,multi-model fusion,optimization,reverse-engineering
AI 理解论文
溯源树
样例
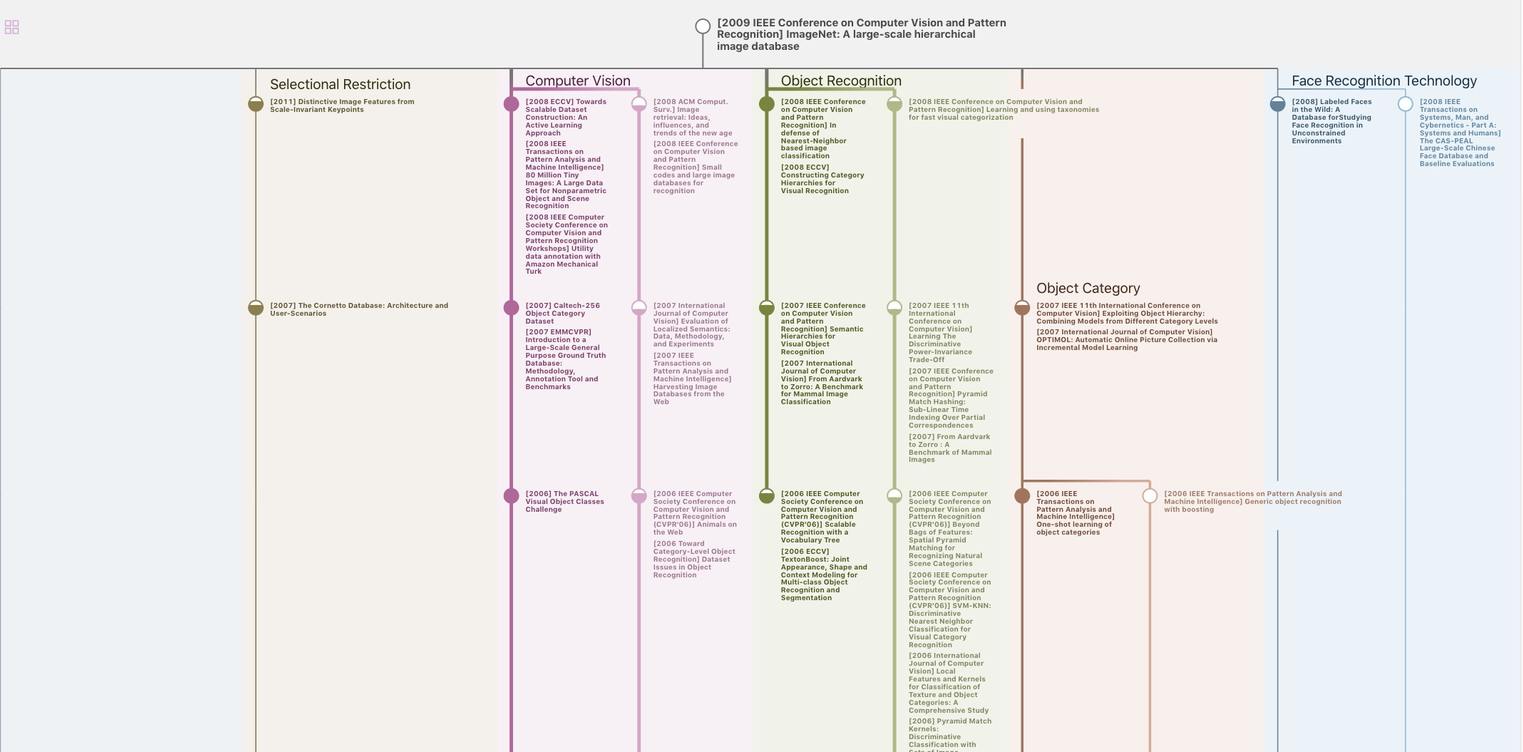
生成溯源树,研究论文发展脉络
Chat Paper
正在生成论文摘要