Improving Web Search Relevance with Semantic Features.
EMNLP '09: Proceedings of the 2009 Conference on Empirical Methods in Natural Language Processing: Volume 2 - Volume 2(2009)
摘要
Most existing information retrieval (IR) systems do not take much advantage of natural language processing (NLP) techniques due to the complexity and limited observed effectiveness of applying NLP to IR. In this paper, we demonstrate that substantial gains can be obtained over a strong baseline using NLP techniques, if properly handled. We propose a framework for deriving semantic text matching features from named entities identified in Web queries; we then utilize these features in a supervised machine-learned ranking approach, applying a set of emerging machine learning techniques. Our approach is especially useful for queries that contain multiple types of concepts. Comparing to a major commercial Web search engine, we observe a substantial 4% DCG5 gain over the affected queries.
更多查看译文
关键词
NLP technique,Web query,major commercial Web search,substantial gain,supervised machine-learned ranking approach,DCG5 gain,affected query,existing information retrieval,limited observed effectiveness,multiple type,Improving web search relevance,semantic feature
AI 理解论文
溯源树
样例
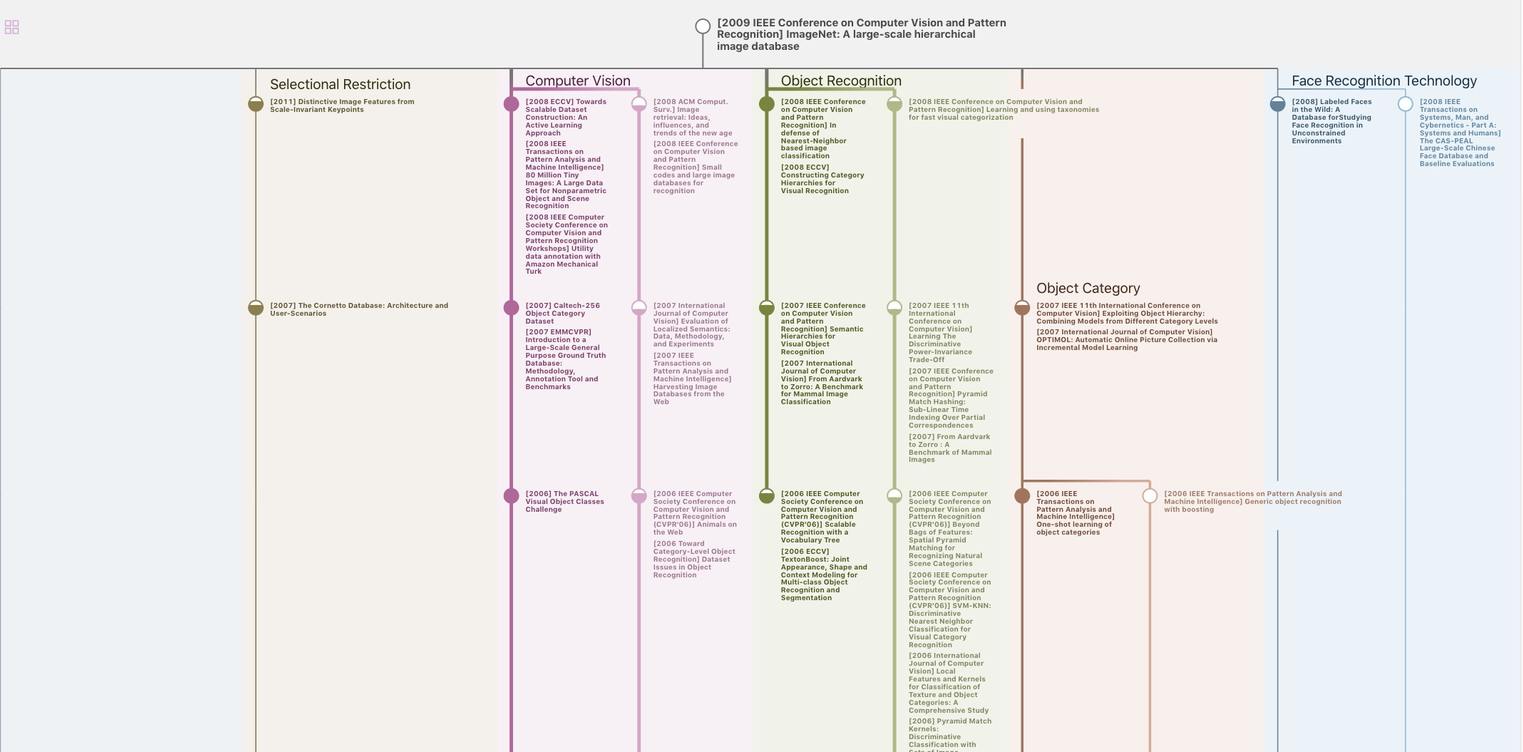
生成溯源树,研究论文发展脉络
Chat Paper
正在生成论文摘要