Applying the FAIR4Health Solution to Identify Multimorbidity Patterns and their Association with Mortality through a Frequent Pattern Growth Association Algorithm: Validation Study. (Preprint)
crossref(2021)
摘要
BACKGROUND Chronic diseases are responsible for most health problems in older people. We know that chronic conditions tend to cluster in the form of patterns, also known as multimorbidity patterns. However, health systems and professionals are generally organized and trained to respond to specific diseases independently, negatively impacting patients and health systems. Different initiatives are trying to respond to these problems. In this context, the current availability of electronic health records and other types of health research data represents an excellent research opportunity. However, there are also some relevant limitations and challenges related to a current lack of tools that allow us to access, harmonize, integrate and reuse datasets technically, legally, ethically, and respectfully to patients and society. In this sense, the FAIR (Findability, Accessibility, Interoperability, and Reusability) principles can help us to guide scientific data management and stewardship and drive scientific discovery to a new paradigm. FAIR4Health is a European Commission supported project that applies FAIR principles on publicly-funded research datasets. OBJECTIVE To present the FAIR4Health pathfinder case study designed to validate and evaluate the FAIR4Health solution with the aim of identifying multimorbidity patterns and their association with mortality in older adults from different health organizations databases of four European countries. METHODS To apply the FAIR principles in five European cohorts from different healthcare settings (i.e., primary care, hospitals, and nursing homes) and institutions (i.e., University of Geneva from Switzerland, Università Cattolica del Sacro Cuore from Italy, University of Porto from Portugal, Instituto Aragonés de Ciencias de la Salud from Spain, and Andalusian Health Service also from Spain), a multicentric retrospective observational study (N = 11,034) was performed. In FAIR4Health, a workflow was designed to implement the FAIR principles on health datasets, and two tools were developed, a Data Curation Tool to transform the raw datasets into FAIR datasets and a Data Privacy Tool to preserve data privacy. On top of these, the FAIR4Health Platform was implemented to provide an interface for researchers, and enable the usage of federated machine learning algorithms on FAIR datasets. In this study, we applied a federated frequent pattern growth association algorithm to identify the most frequent disease patterns among a set of variables. RESULTS We applied the FAIR principles in the health research datasets from different organizations, and we were able to reuse and integrate heterogeneous datasets, increasing the variability of data compared to the studies not applying those principles. We identified and described high-frequent multimorbidity patterns consistent with the literature and observed a strong association with polypharmacy and mortality. CONCLUSIONS Our results highlight the importance of implementing the FAIR data policy to overcome the difficulties in data management and accelerate responsible health research with patients and society.
更多查看译文
AI 理解论文
溯源树
样例
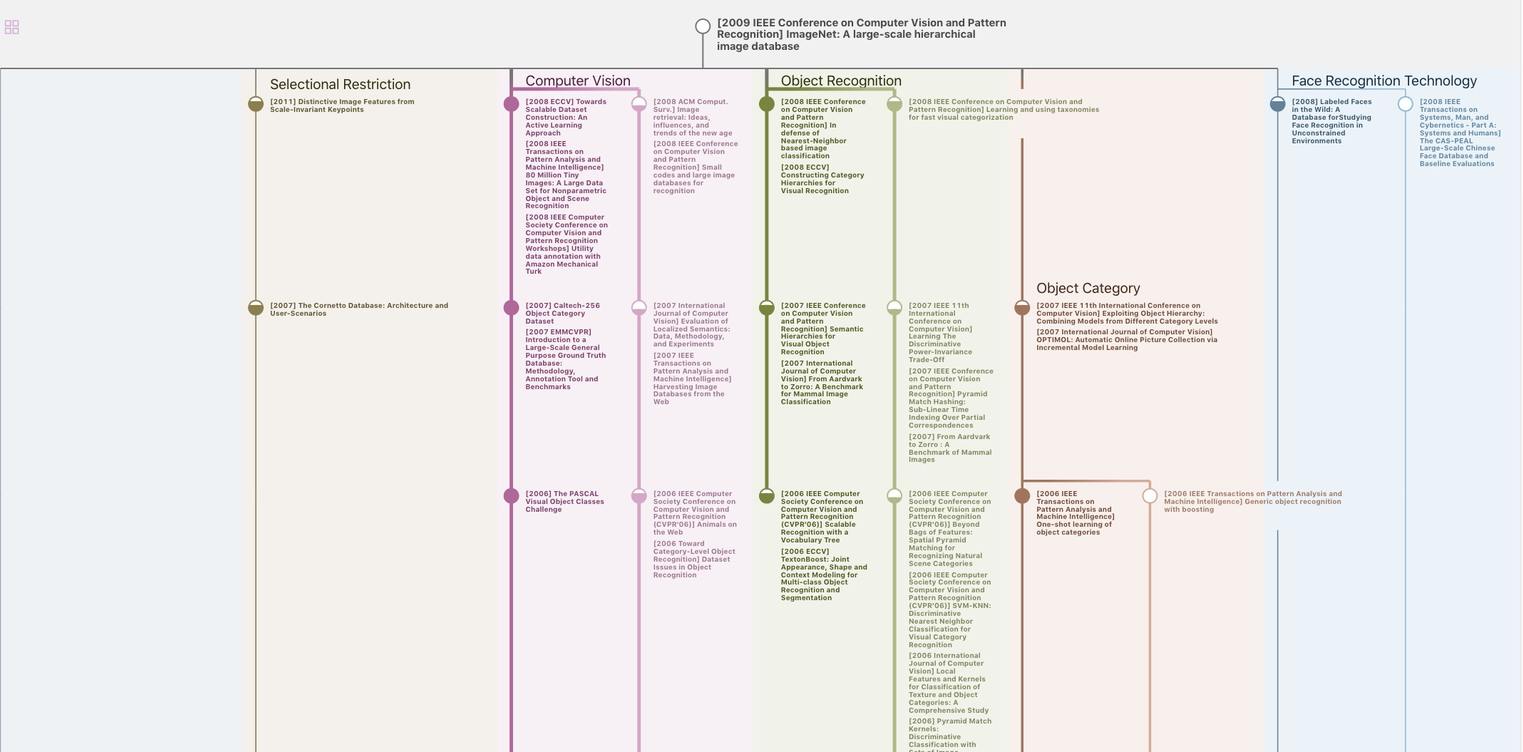
生成溯源树,研究论文发展脉络
Chat Paper
正在生成论文摘要