Deep Compression For Dense Point Cloud Maps
IEEE ROBOTICS AND AUTOMATION LETTERS(2021)
摘要
Many modern robotics applications rely on 3D maps of the environment. Due to the large memory requirements of dense 3D maps, compression techniques are often necessary to store or transmit 3D maps efficiently. In this work, we investigate the problem of compressing dense 3D point cloud maps such as those obtained from an autonomous vehicle in large outdoor environments. We tackle the problem by learning a set of local feature descriptors from which the point cloud can be reconstructed efficiently and effectively. We propose a novel deep convolutional autoencoder architecture that directly operates on the points themselves so that we avoid voxelization. Additionally, we propose a deconvolution operator to upsample point clouds, which allows us to decompress to an arbitrary density. Our experiments show that our learned compression achieves better reconstructions at the same bit rate compared to other state-of-the-art compression algorithms. We furthermore demonstrate that our approach generalizes well to different LiDAR sensors. For example, networks learned on maps generated from KITTI point clouds still achieve state-of-the-art compression results for maps generated from nuScences point clouds.
更多查看译文
关键词
Deep learning methods, mapping
AI 理解论文
溯源树
样例
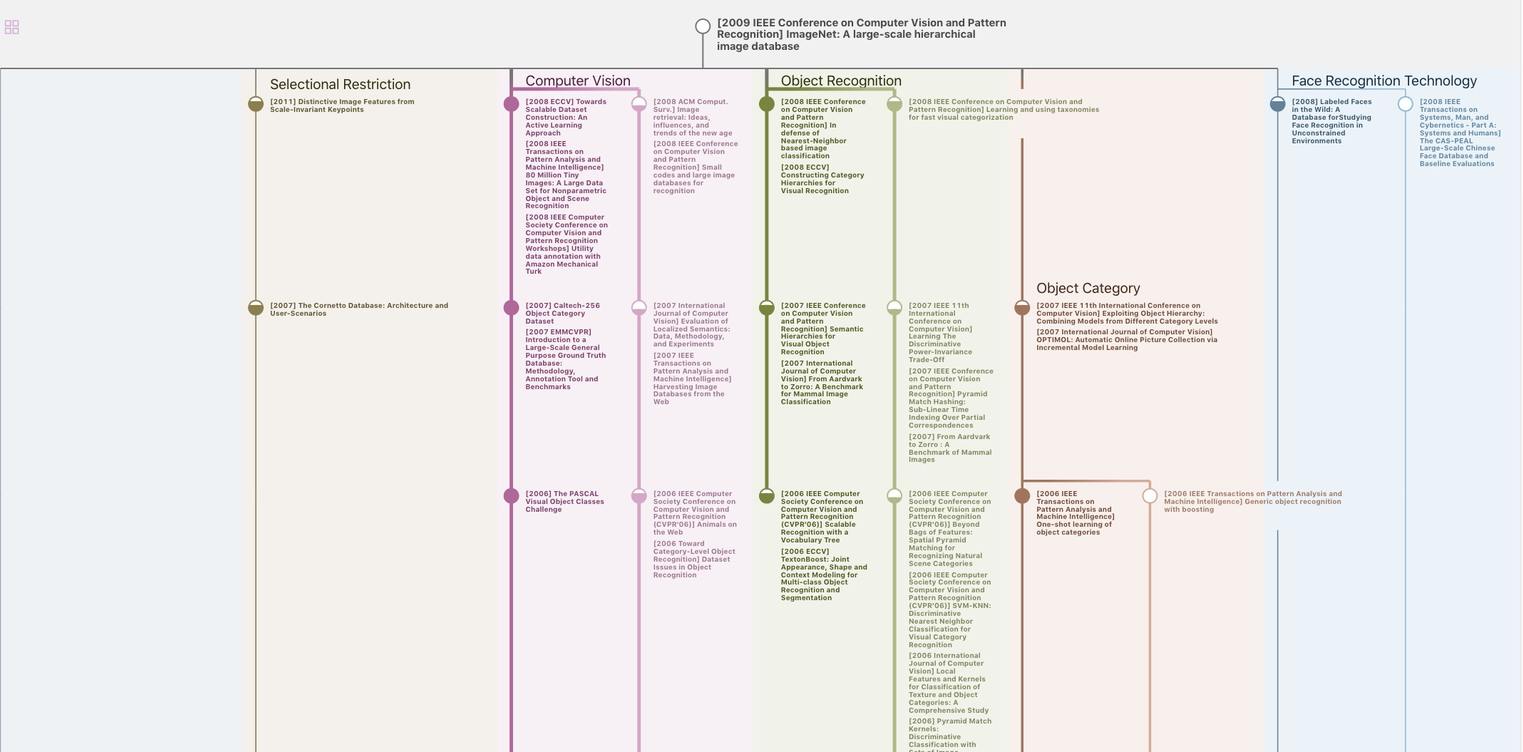
生成溯源树,研究论文发展脉络
Chat Paper
正在生成论文摘要