CLiMF: Collaborative Less-Is-More Filtering.
IJCAI '13: Proceedings of the Twenty-Third international joint conference on Artificial Intelligence(2013)
摘要
In this paper we tackle the problem of recommendation in the scenarios with binary relevance data, when only a few ( k ) items are recommended to individual users. Past work on Collaborative Filtering (CF) has either not addressed the ranking problem for binary relevance datasets, or not specifically focused on improving top- k recommendations. To solve the problem we propose a new CF approach, Collaborative Less-is-More Filtering (CLiMF). In CLiMF the model parameters are learned by directly maximizing the Mean Reciprocal Rank (MRR), which is a well-known information retrieval metric for capturing the performance of top-k recommendations. We achieve linear computational complexity by introducing a lower bound of the smoothed reciprocal rank metric. Experiments on two social network datasets show that CLiMF significantly outperforms a naive baseline and two state-of-the-art CF methods.
更多查看译文
关键词
top-k recommendation,new CF approach,ranking problem,state-of-the-art CF method,Collaborative Filtering,Collaborative Less-is-More Filtering,binary relevance data,binary relevance datasets,social network datasets,Mean Reciprocal Rank,collaborative less-is-more
AI 理解论文
溯源树
样例
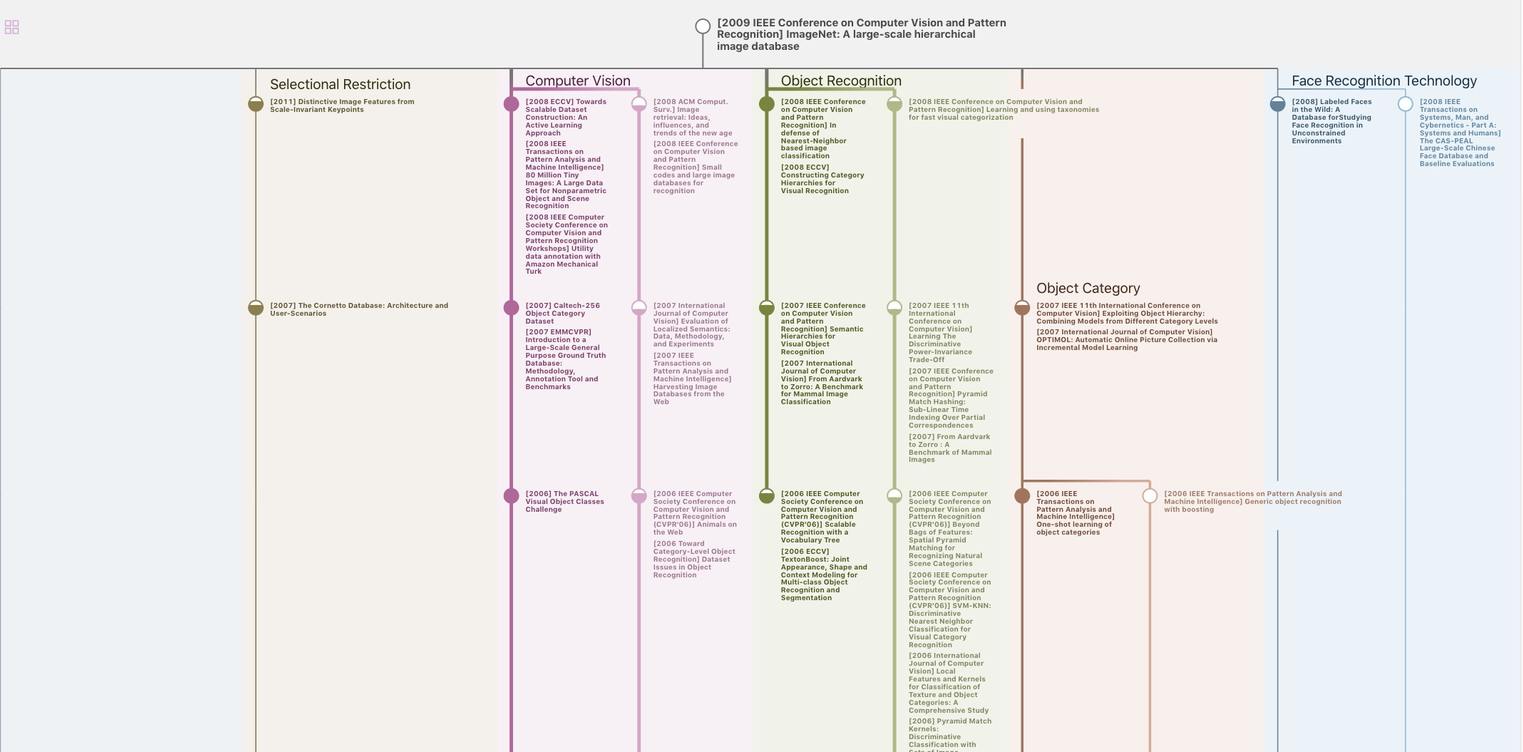
生成溯源树,研究论文发展脉络
Chat Paper
正在生成论文摘要