The Secret Life of Motion Covariates
Neuroimage(2009)
摘要
Abstract Motion covariates are often added to subject-level design matrices to reduce the effects of subject motion. This has been dem-onstrated to increase t-statistics despite the lack of a clear model between these covariates and EPI signal magnitude. Three studies were analyzed with a variety of choices for motion covariates. Results demonstrate that in addition to removing varianc e due to motion, the extra “secret” dimensions in the motion covariates remove signal and noise components unrelated to motion. Although not shown to be harmful at the group level, their lack of specificity and efficacy calls their use into question. Introduction Rigid body motion introduces error in EPI time-series both through misalignment of anatomical structures and through dis-ruption of the spin-history steady-state. Six degree-of-freedom realignment algorithms such as those in AFNI, SPM, and FSL accurately compensate for anatomical misalignment across frames but spin-history effects are more problematic. Motion es-sentially redefines slice boundaries such that the new boundaries contain spins formerly in neigboring slices at different stag es of T1 relaxation. The relatively large differences in the magnitude between adjacent slices leads to relatively large signal changes even for small motions. Steady-state is restored after several seconds (depending on the flip angle), so each discrete motion causes an excursion in signal magnitude lasting beyond the frame in which it occursJ. Three approaches have been used to compensate for spin-history effects: 1. Omitting subjects with excessive motion from the analysis, 2. Omitting frames following a large motion from the analysis, and 3) Incorporating a regressor into the general linear model to model signal excursions introduced by motion (Jiang 1995,Friston 1996, Johnstone 2006). The first two are straightforward and effective if motion is infrequent. The third approach of adding regressors to the design matrixe is widely used. The most frequent choices are the rotation and translation parameters of the rigid body transformation. This has sever al shortcomings. First, the regressors do not model the relaxation effects, so they cannot match the temporal dynamics of the signal. Second, the signs of the angles and translations differ randomly from the sign of moton-induced signal changes. Third , they comprise a 6-dimensional subspace when only one is required. And finally, the motion covariates best represent in-plane motion that has little effect on the signal while poorly representing through-slice motion (due to thick slices). The initial goal of this research was to develop improved regressors at both the volume and slice levels. The underlying i dea is that the large signal excursion caused by motion can also be thought of as an excellent signal for estimating its magnitude of its effect, and that this signal can be formed into a more effective regressor if it is decoupled from task-related and phys iologi-cal noise effects. The result of testing these regressors has been to show that motion regressors are ineffective in general.
更多查看译文
关键词
motion,secret life
AI 理解论文
溯源树
样例
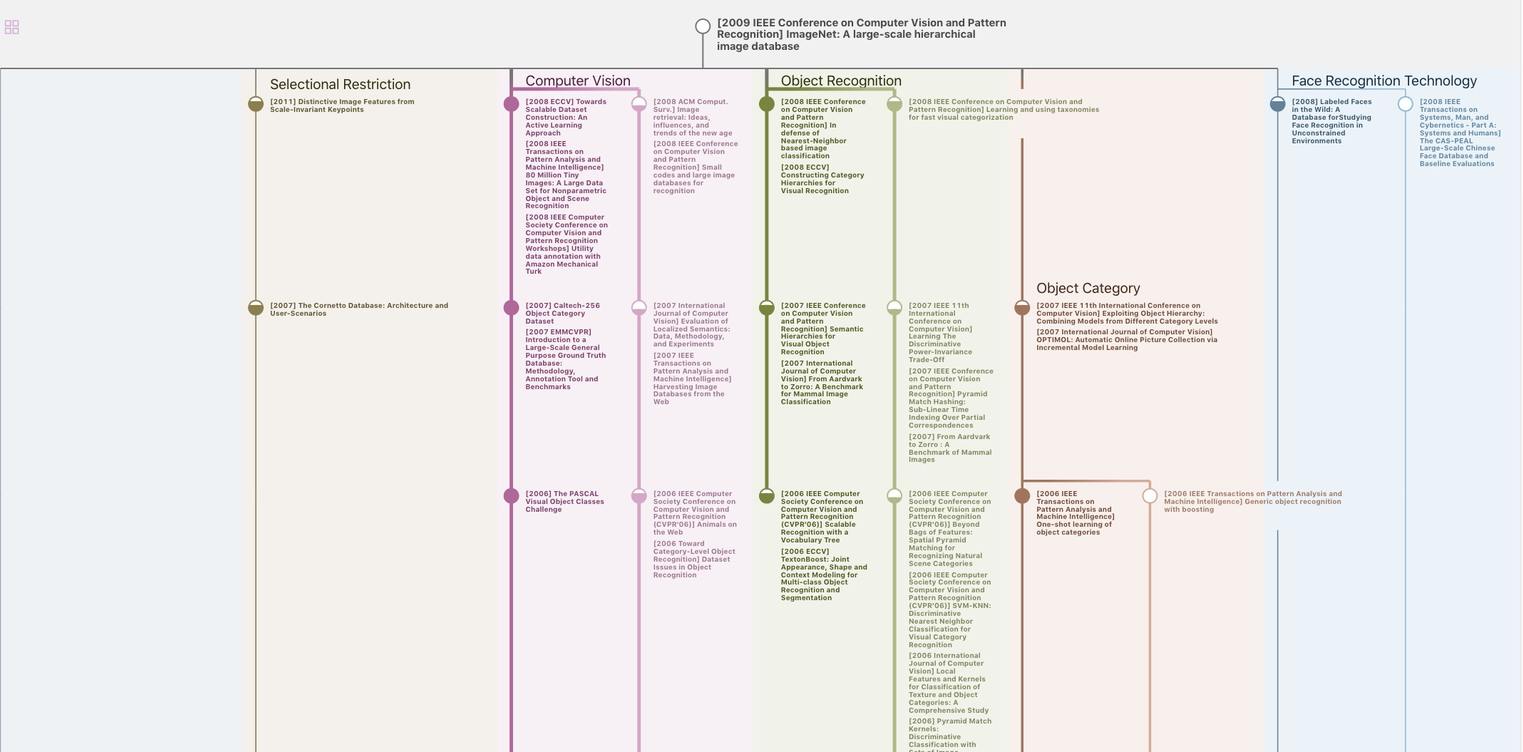
生成溯源树,研究论文发展脉络
Chat Paper
正在生成论文摘要