Computational investigation of signaling regimens using proteomics data
Computational investigation of signaling regimens using proteomics data(2012)
摘要
We present computational methods addressing three key challenges in the quest to construct a more complete picture of protein signaling pathways, namely, confident identification of proteins in a sample, functional classification of large-scale proteomics data, and characterization of the dynamic conformational changes in protein structures.First, we develop a probabilistic protocol for identification of short peptide fragments characterized by tandem mass-spectromety (MS/MS). A machine learning procedure for correctly matching peptides with mass spectra was constructed. Further, we demonstrated how the developed model can be represented as an interpretable tree of rules, thereby effectively removing the 'black-box' notion often associated with machine learning classifiers, making the underlying model clearer to end-users. Finally, using a probabilistic framework, a method for protein identification based on the peptide predictions was proposed and tested.Second, a genome-wide functional classification protocol for identifying dual specificity membrane- and protein-binding domains was developed. Experimental characterization of 90 PDZ domains demonstrating that 40% had submicromolar membrane affinity was used for building a model utilized to predict the membrane binding properties of 2000 PDZ domains from 20 species. We demonstrate that reversible membrane binding is a key component in spatially regulation protein interaction networks and further propose a mechanistic classification of dual-specificity binding. As an extension to the PDZ domain models, we build a knowledge-mining procedure for learning the general mechanisms of membrane-binding, using C1, C2, and PH domains as test-beds. We demonstrate how this method was able to uncover properties of each family known to be important in membrane-binding. Last, we present a method for modeling the changes in single molecule dynamics induced by a signaling event as a discrete state Markov Chain model. Specifically, we use the partial unfolding of so-called mechanical proteins by way of steered molecular dynamics to demonstrate how the protein energy landscape is altered when different external mechanical forces are applied. By probing the protein structure with a range of forces, we show that the transitions pathways taking the protein structure from folded to partially unfolded vary significantly depending on the external input. The constructed model is instrumental in explaining experimental single molecule studies of the unfolding of the protein domain I27, as well as the changes in mechanical properties of a number of I27 mutant structures.
更多查看译文
关键词
Markov Chain model,protein energy landscape,developed model,spatially regulation protein interaction,so-called mechanical protein,PDZ domain model,protein structure,protein domain I27,computational investigation,proteomics data,underlying model,protein identification
AI 理解论文
溯源树
样例
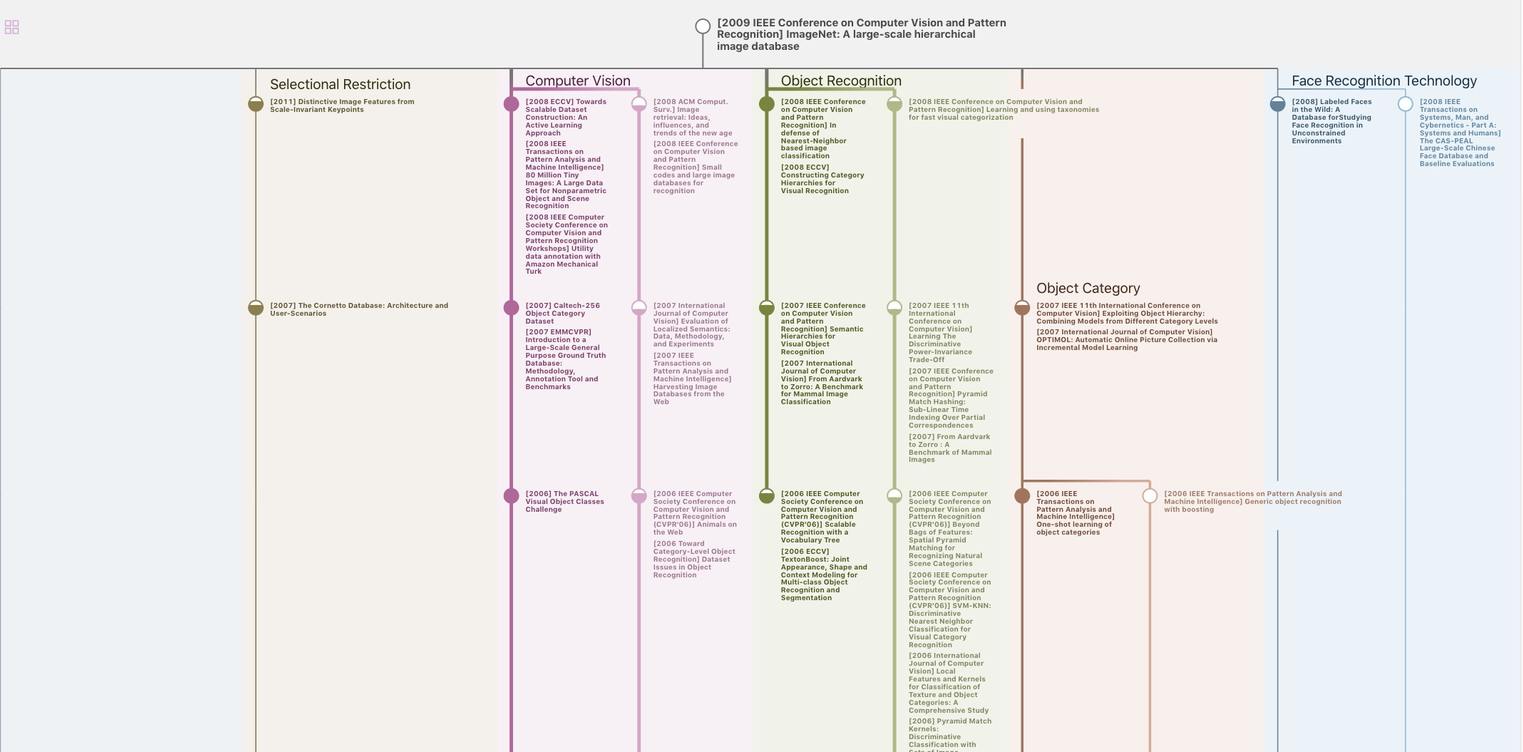
生成溯源树,研究论文发展脉络
Chat Paper
正在生成论文摘要